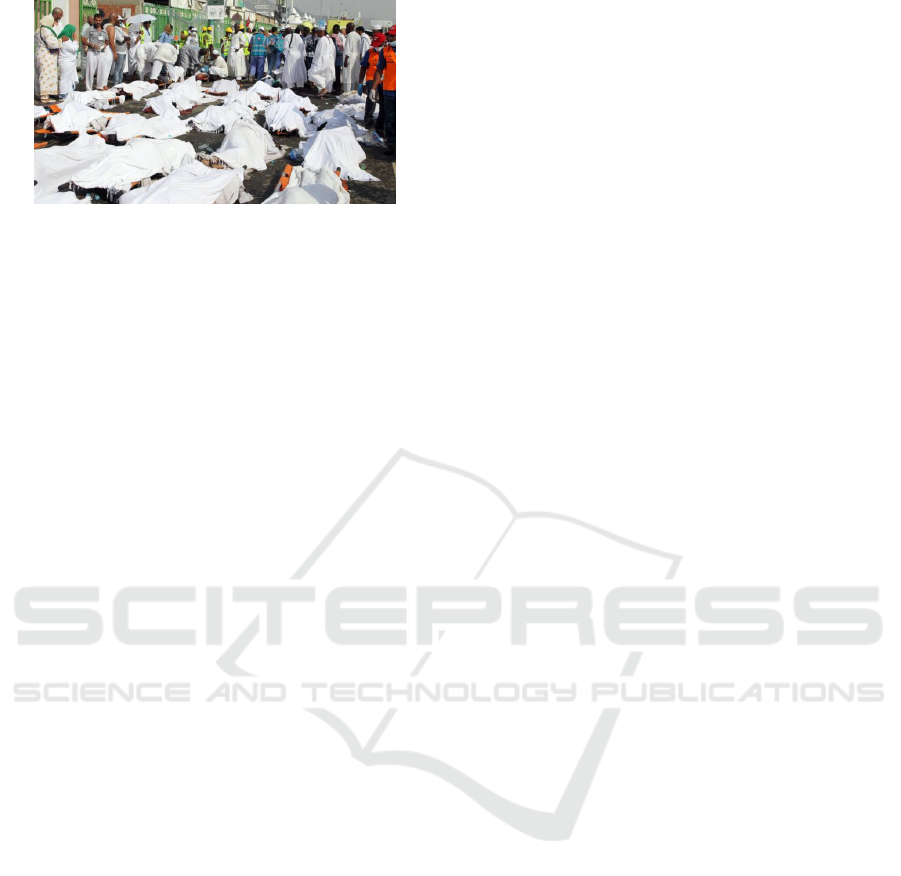
Figure 4: Tragic human stampedes.
at Mina. This pattern prevents crows and panic stam-
ples while stoning the devil by taking into considera-
tion number of predistians within a specific time pe-
riod and limited space location of the tent city.
The remaining part of the paper is organized as
follows. Section 2 tackles some related works of some
human mobility models previously suggested for dif-
ferent and under diverse constraints. Section 3 high-
lights the mobility features, the main motivation, the
detailed process and properties of the proposed hu-
man mobility model. Section 4 discusses the obtained
pattern, and finally the conclusion.
2 RELATED WORKS
Human motion management research field knows
many human mobility models that have been pro-
posed till now. These patterns are primary applied to
extract and recognize human whereabouts for mobile
networks. Among these patterns, we propose a brief
description of the most used at network simulations:
In (Jeon et al., 2017), the authors present the
Community-based mobility model (CMM) where the
motion of mobile nodes are based on socialization be-
havior and human decision. These devices are carried
by Humans which by nature they tend to gather and
establish communities. All movements are in groups
and between groups. This mobility heavily depends
on the social relationships among devices. An Inter-
action matrix is used to model structure of connex-
ions between people carrying devices. The strength
of interaction between any two nodes i and j are rep-
resented by an element m(i,j). A connectivity ma-
trix (C) is picked up where if and only if m(i,j) is
bigger than a specific threshold (often 0.25) leads to
C(i,j)=1 and if it is less than 0.25, the c(i,j)=0. From
this matrix, we can detect structures of relationships
into a community via algorithms which already pro-
posed Girvan and Newman (Girvan and Newman,
2001). Communities are initially located within a def-
inite place or a square inside a grid. Moreover, a
given device may change its current position after a
time-interval t. And, the next waypoint (destination)
mainly relies on the location of other nodes with a
strong social connectivity. Furthermore, every node is
found in various communities at different times. That
reflects real-life activity where a person is at differ-
ent locations within a day. For instance, a person is
mostly at the office from morning till afternoon, at the
coffee shop in the evening with friends, and at home
at night with family. So, a person changes communi-
ties belonging within a day at different locations and
at different times.
In (Ibadah et al., 2018), Self-Similar Least Ac-
tion Walk (SLAW) has been introduced which com-
bines the main features of human motion, as: least-
action trip planning (LATP), truncated power-law
inter-contact times, heavy-tail flight and pause-time
distribution, self-similar dispersal of destinations, and
heterogeneously bounded mobility areas of individu-
als.
The authors analyze major parts of Dartmouth
WLAN traces to suggest Time-Variant Community
Model (TVCM). They recognize two main mobility
features. They differentiated skewed location visit-
ing preferences and periodical re-appearance at the
same position. Skewed location visiting preferences
demonstrates how one node can spend its time at di-
verse places. However, periodical re-appearance at
the same position displays the period in which a de-
vice appears at the same place again. According to
traces, a node spends at a specific AP an average of
65% of its online time and more than 95% at few than
5 APs (Batabyal and Bhaumik, 2015).
In (Wang et al., 2015), Sociological Orbit aware
Location Approximation and Routing (SOLAR) con-
siders repeated visits of a given node to a set of lo-
cations. It is noticed that people routinely located
at a specific place for an appointed period of their
daily lives. The authors call these locations as ”hubs”.
Thus, the ”mobility profile” of a person is the repeti-
tion of inter-hub motion which is in generally periodic
or orbital. In SOLAR, the whole experimental area is
divided into several hubs. Every individual randomly
selects a set of hubs which it can visit, as a hub-list.
Based on the ”hub-stay time”, each node remains or
travel inside the hub. And then, it moves to the next
hub. The intra-hub motion can adopt random walk or
random waypoint and the inter-hub is linear.
In (Mei and Stefa, 2009), Small World in Motion
(SWIM) is presented where its concept is based on
two human mobility facts; 1. Human tend to mostly
visit some popular places and frequently near to their
home. And, they seldom visit far places and less pop-
ular. And, 2. Human spend less time at less popular
places and most time at the most popular. However,
PECCS 2020 - 10th International Conference on Pervasive and Parallel Computing, Communication and Sensors
68