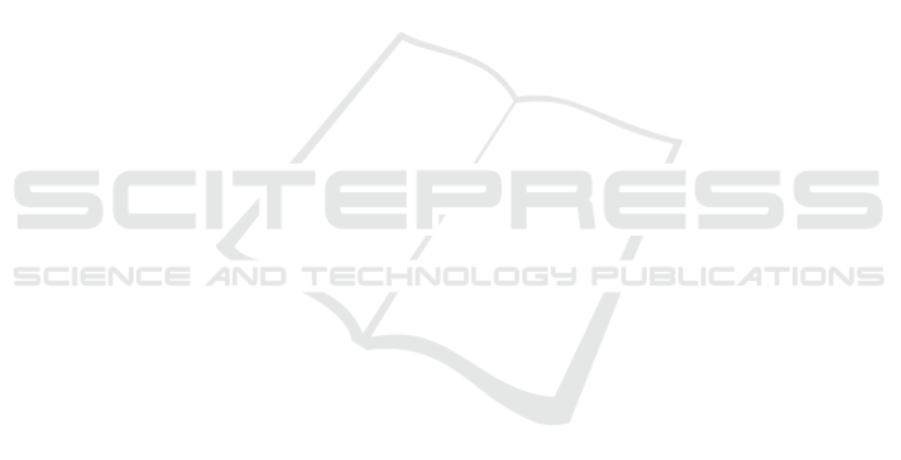
and Knowledge in Applications and Services, pages
324–334, Cham. Springer International Publishing.
Hone, K. (2014). Usability measurement for speech sys-
tems : Sassi revisited. In SIGCHI Conference Paper,
Toronto.
ISO/TC 159/SC 4 Ergonomics of human-system interaction
(2010). Part 210: Human-centred design for interac-
tive systems. In Ergonomics of human-system inter-
action, volume 1, page 32. International Organization
for Standardization (ISO), Brussels.
Kinsella, B. and Mutchler, A. (2018). Voice assistant
consumer adoption report. https://voicebot.ai/wp-
content/uploads/2019/01/voice-assistant-consumer-
adoption-report-2018-voicebot.pdf.
Klein, A. M., Hinderks, A., Rauschenberger, M., and
Thomaschewski, J. (2020a). Protocol for Exploring
Voice Assistant Risks and Potential with Technology-
based Users. https://doi.org/10.13140/RG.2.2.21473.
12646.
Klein, A. M., Hinderks, A., Schrepp, M., and
Thomaschewski, J. (2020b). Construction of
UEQ+ Scales for Voice Quality. In Proceedings of
the Conference on Mensch Und Computer, MuC
’20, page 1–5, New York, NY, USA. Association for
Computing Machinery.
Klein, A. M., Hinderks, A., Schrepp, M., and
Thomaschewski, J. (2020c). Measuring User
Experience Quality of Voice Assistants. In 2020
15th Iberian Conference on Information Systems and
Technologies (CISTI), pages 1–4. IEEE.
Knote, R., Janson, A., S
¨
ollner, M., and Leimeister, J. M.
(2019). Classifying smart personal assistants: An em-
pirical cluster analysis. In Proceedings of the 52nd
Hawaii International Conference on System Sciences.
Labs, L. (2017). Neues Botnetz
¨
uber IoT-
Ger
¨
ate [New botnet about IoT devices].
https://www.heise.de/security/meldung/Neues-
Botnetz-ueber-IoT-Geraete-3867237.html.
Laugwitz, B., Held, T., and Schrepp, M. (2008). Construc-
tion and evaluation of a user experience questionnaire.
In Symposium of the Austrian HCI and usability engi-
neering group, volume 5298, pages 63–76. Springer.
Minge, Michael and Riedel, Laura (2013). meCUE – Ein
modularer Fragebogen zur Erfassung des Nutzungser-
lebens. In: S. Boll, S.Maaß & R. Malaka (Hrsg.):
Mensch und Computer 2013: Interaktive Vielfalt (S.
89-98). M
¨
unchen, Oldenbourg Verlag.
Porcheron, M., Fischer, J. E., and Sharples, S. (2017).
“do animals have accents?”: Talking with agents in
multi-party conversation. In Proceedings of the 2017
ACM Conference on Computer Supported Coopera-
tive Work and Social Computing, CSCW ’17, page
207–219, New York, NY, USA. Association for Com-
puting Machinery.
Pyae, A. and Joelsson, T. N. (2018). Investigating the us-
ability and user experiences of voice user interface:
A case of google home smart speaker. In Proceed-
ings of the 20th International Conference on Human-
Computer Interaction with Mobile Devices and Ser-
vices Adjunct, MobileHCI ’18, page 127–131, New
York, NY, USA. Association for Computing Machin-
ery.
Rauschenberger, M., Schrepp, M., Cota, M. P., Olschner,
S., and Thomaschewski, J. (2013). Efficient Mea-
surement of the User Experience of Interactive Prod-
ucts. How to use the User Experience Questionnaire
(UEQ). Example: Spanish Language. International
Journal of Artificial Intelligence and Interactive Mul-
timedia (IJIMAI), 2(1):39–45.
Ripa, G., Torre, M., Firmenich, S., and Rossi, G. (2019).
End-user development of voice user interfaces based
on web content. In Malizia, A., Valtolina, S., Morch,
A., Serrano, A., and Stratton, A., editors, End-User
Development, pages 34–50, Cham. Springer Interna-
tional Publishing.
Scherschel, F. A. (2017). Mirai-Botnetz lernt
neue Tricks [Mirai botnet learns new tricks].
https://www.heise.de/security/meldung/Mirai-
Botnetz-lernt-neue-Tricks-3670226.html.
Schirrmacher, D. (2016). Source Code von m
¨
achtigem
DDoS-Tool Mirai ver
¨
offentlicht [Source code
of powerful DDoS tool Mirai released].
https://www.heise.de/security/meldung/Source-
Code-von-maechtigem-DDoS-Tool-Mirai-
veroeffentlicht-3345809.html?view=print.
Schrepp, M. and Thomaschewski, J. (2019). Design and
Validation of a Framework for the Creation of User
Experience Questionnaires. International Journal
of Interactive Multimedia and Artificial Intelligence,
5(7):88–95.
Sciuto, A., Saini, A., Forlizzi, J., and Hong, J. I. (2018).
“hey alexa, what’s up?”: A mixed-methods studies
of in-home conversational agent usage. In Proceed-
ings of the 2018 Designing Interactive Systems Con-
ference, DIS ’18, page 857–868, New York, NY, USA.
Association for Computing Machinery.
SPLENDID RESEARCH GmbH (2019). Digitale sprachas-
sistenten. https://www.splendid-research.com/de/
studie-digitale-sprachassistenten.html.
Statista (2020). MINT Studienanf
¨
anger an deutschen
Hochschulen bis 2018/2019 (STEM First-year
students at German universities until 2018/2019).
https://de.statista.com/statistik/daten/studie/28346/
umfrage/anzahl-der-mint-studienanfaenger/.
Tas, S., Hildebrandt, C., and Arnold, R. (2019). Voice as-
sistants in germany. https://www.wik.org.
Tractica (2020). Tractica. https://tractica.omdia.
com/newsroom/press-releases/voice-and-speech-
recognition-software-market-to-reach-6-9-billion-
by-2025/.
Tuzovic, S. and Paluch, S. (2018). Conversational Com-
merce – A New Era for Service Business Develop-
ment?, pages 81–100. Springer Fachmedien Wies-
baden, Wiesbaden.
WEBIST 2020 - 16th International Conference on Web Information Systems and Technologies
154