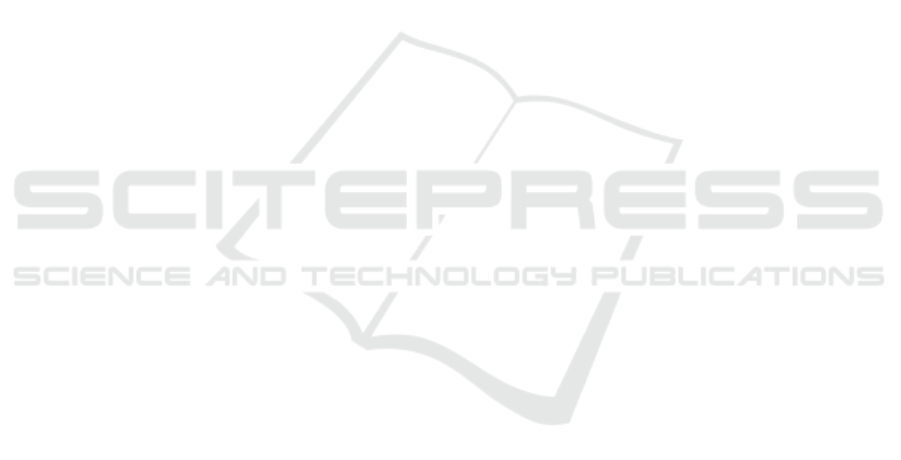
Chen, X., Fang, H., Lin, T., Vedantam, R., Gupta, S., Doll
´
ar,
P., and Zitnick, C. L. (2015). Microsoft COCO cap-
tions: Data collection and evaluation server. CoRR,
abs/1504.00325.
Chew, C. and Eysenbach, G. (2010). Pandemics in the age
of twitter: Content analysis of tweets during the 2009
h1n1 outbreak. PLOS ONE, 5(11):1–13.
French, J. H. (2017). Image-based memes as sentiment pre-
dictors. In i-Society, pages 80–85.
Fu, J., Liu, J., Tian, H., Li, Y., Bao, Y., Fang, Z., and Lu,
H. (2019). Dual attention network for scene segmen-
tation. In cvpr, pages 3146–3154.
Fukui, A., Park, D. H., Yang, D., Rohrbach, A., Darrell, T.,
and Rohrbach, M. (2016). Multimodal compact bilin-
ear pooling for visual question answering and visual
grounding. arXiv preprint arXiv:1606.01847.
Gurari, D., Zhao, Y., Zhang, M., and Bhattacharya, N.
(2020). Captioning images taken by people who are
blind.
He, K., Zhang, X., Ren, S., and Sun, J. (2015). Deep
residual learning for image recognition. CoRR,
abs/1512.03385.
He, S., Zheng, X., Wang, J., Chang, Z., Luo, Y., and Zeng,
D. (2016). Meme extraction and tracing in crisis
events. In ISI, pages 61–66.
JafariAsbagh, M., Ferrara, E., Varol, O., Menczer, F., and
Flammini, A. (2014). Clustering memes in social me-
dia streams. CoRR, abs/1411.0652.
Koch, G., Zemel, R., and Salakhutdinov, R. (2015).
Siamese neural networks for one-shot image recogni-
tion.
Krishna, R., Zhu, Y., Groth, O., Johnson, J., Hata, K.,
Kravitz, J., Chen, S., Kalantidis, Y., Li, L., Shamma,
D. A., Bernstein, M. S., and Li, F. (2016). Vi-
sual genome: Connecting language and vision us-
ing crowdsourced dense image annotations. CoRR,
abs/1602.07332.
Krizhevsky, A., Sutskever, I., and Hinton, G. E. (2012).
Imagenet classification with deep convolutional neu-
ral networks. In Pereira, F., Burges, C. J. C., Bottou,
L., and Weinberger, K. Q., editors, ANIPS 25, pages
1097–1105. Curran Associates, Inc.
Li, G., Duan, N., Fang, Y., Gong, M., Jiang, D., and Zhou,
M. (2020). Unicoder-vl: A universal encoder for vi-
sion and language by cross-modal pre-training. In
AAAI, pages 11336–11344.
Machajdik, J. and Hanbury, A. (2010). Affective image
classification using features inspired by psychology
and art theory. page 83–92, New York, NY, USA. As-
sociation for Computing Machinery.
Pennington, J., Socher, R., and Manning, C. (2014). Glove:
Global vectors for word representation. volume 14,
pages 1532–1543.
Perez-Martin, J., Bustos, B., and Saldana, M. (2020). Se-
mantic search of memes on twitter.
Qiao, S., Chen, L.-C., and Yuille, A. (2020). Detectors:
Detecting objects with recursive feature pyramid and
switchable atrous convolution.
Sidorov, O., Hu, R., Rohrbach, M., and Singh, A. (2020).
Textcaps: a dataset for image captioning with reading
comprehension.
Simonyan, K. and Zisserman, A. (2014). Very deep con-
volutional networks for large-scale image recognition.
arXiv 1409.1556.
Suryawanshi, S., Chakravarthi, B. R., Verma, P., Arcan, M.,
McCrae, J. P., and Buitelaar, P. A dataset for troll
classification of TamilMemes.
Szegedy, C., Vanhoucke, V., Ioffe, S., Shlens, J., and Wojna,
Z. (2015). Rethinking the inception architecture for
computer vision. CoRR, abs/1512.00567.
Teney, D., Anderson, P., He, X., and Van Den Hengel, A.
(2018). Tips and tricks for visual question answering:
Learnings from the 2017 challenge. In cvpr, pages
4223–4232.
Thomee, B., Shamma, D. A., Friedland, G., Elizalde, B.,
Ni, K., Poland, D., Borth, D., and Li, L. (2015). The
new data and new challenges in multimedia research.
CoRR, abs/1503.01817.
Truong, B. Q., Sun, A., and Bhowmick, S. S. (2012). Casis:
A system for concept-aware social image search. page
425–428. Association for Computing Machinery.
Tsur, O. and Rappoport, A. (2015). Don’t let me be #misun-
derstood: Linguistically motivated algorithm for pre-
dicting the popularity of textual memes.
V, A. L. P. and Tolunay, E. M. (2018). Dank learning: Gen-
erating memes using deep neural networks. CoRR,
abs/1806.04510.
van der Maaten, L. and Hinton, G. (2008). Visualizing data
using t-sne.
Vinyals, O., Toshev, A., Bengio, S., and Erhan, D. (2015).
Show and tell: A neural image caption generator. In
cvpr, pages 3156–3164.
Weenink, D. Canonical correlation analysis.
Xu, K., Ba, J., Kiros, R., Cho, K., Courville, A., Salakhudi-
nov, R., Zemel, R., and Bengio, Y. (2015). Show, at-
tend and tell: Neural image caption generation with
visual attention. In icml, pages 2048–2057.
Xu, Y., Yu, J., Guo, J., Hu, Y., and Tan, J. (2019).
Fine-grained label learning via siamese network for
cross-modal information retrieval. In Rodrigues, J.
M. F., Cardoso, P. J. S., Monteiro, J., Lam, R.,
Krzhizhanovskaya, V. V., Lees, M. H., Dongarra, J. J.,
and Sloot, P. M., editors, ICCS 2019. Springer Inter-
national Publishing.
Young, P., Lai, A., Hodosh, M., and Hockenmaier, J.
(2014). From image descriptions to visual denota-
tions: New similarity metrics for semantic inference
over event descriptions. ACL, 2:67–78.
Zoph, B., Ghiasi, G., Lin, T.-Y., Cui, Y., Liu, H., Cubuk,
E. D., and Le, Q. V. (2020). Rethinking pre-training
and self-training.
WEBIST 2020 - 16th International Conference on Web Information Systems and Technologies
360