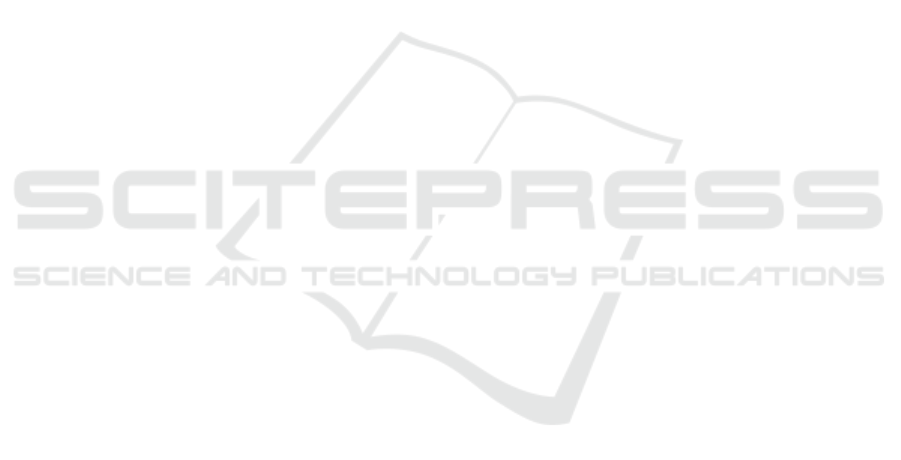
Hallikainen, H. and Laukkanen, T. (2018). National cul-
ture and consumer trust in e-commerce. International
Journal of Information Management, 38(1):97–106.
Howard, J. (2019). Bandwagon effect and authority bias.
In Cognitive Errors and Diagnostic Mistakes, pages
21–56. Springer.
Im, J., Chandrasekharan, E., Sargent, J., Lighthammer, P.,
Denby, T., Bhargava, A., Hemphill, L., Jurgens, D.,
and Gilbert, E. (2020). Still out there: Modeling and
identifying russian troll accounts on Twitter. In ACM
Conference on Web science, pages 1–10.
Jain, N., Kumar, S., Kumar, A., Shamsolmoali, P., and
Zareapoor, M. (2018). Hybrid deep neural networks
for face emotion recognition. Pattern Recognition Let-
ters, 115:101–106.
Jiang, L., Zhang, L., Yu, L., and Wang, D. (2019). Class-
specific attribute weighted naive Bayes. Pattern
Recognition, 88:321–330.
Kamvar, S. D., Schlosser, M. T., and Garcia-Molina, H.
(2003). The EigenTrust algorithm for reputation man-
agement in P2P networks. In WWW, pages 640–651.
Kim, Y. and Peterson, R. A. (2017). A meta-analysis of
online trust relationships in e-commerce. Journal of
Interactive Marketing, 38:44–54.
Kyriazidou, I., Drakopoulos, G., Kanavos, A., Makris, C.,
and Mylonas, P. (2019). Towards predicting mentions
to verified Twitter accounts: Building prediction mod-
els over MongoDB with keras. In WEBIST, pages 25–
33.
Lewis, M., Zettersten, M., and Lupyan, G. (2019). Distri-
butional semantics as a source of visual knowledge.
PNAS, 116(39):19237–19238.
Li, X., Jiang, P., Chen, T., Luo, X., and Wen, Q. (2020). A
survey on the security of blockchain systems. Future
Generation Computer Systems, 107:841–853.
Liang, G., Weller, S. R., Luo, F., Zhao, J., and Dong,
Z. Y. (2018). Distributed blockchain-based data pro-
tection framework for modern power systems against
cyber attacks. IEEE Transactions on smart grid,
10(3):3162–3173.
Lumbreras, A. and Gavald
`
a, R. (2012). Applying trust met-
rics based on user interactions to recommendation in
social networks. In ASONAM, pages 1159–1164.
Massa, P. and Bhattacharjee, B. (2004). Using trust in rec-
ommender systems: An experimental analysis. In
International conference on trust management, pages
221–235. Springer.
Obimbo, C., Speller, A., Myers, K., Burke, A., and Blatz,
M. (2018). Internet worms and the weakest link: Hu-
man error. In CSCI, pages 120–123. IEEE.
O’Donovan, J. and Smyth, B. (2005). Trust in recommender
systems. In IUI, pages 167–174.
Oliveira, T., Alhinho, M., Rita, P., and Dhillon, G. (2017).
Modelling and testing consumer trust dimensions in e-
commerce. Computers in Human Behavior, 71:153–
164.
Papaoikonomou, T., Kardara, M., Tserpes, K., and Var-
varigou, T. A. (2013). The strength of negative opin-
ions. In EANN, volume 384, pages 90–99.
Patil, S., Deshpande, S., and Potgantwar, A. D. (2017).
Product recommendation using multiple filtering
mechanisms on Apache Spark. International Journal
of Scientific Research in Network Security and Com-
munication, 5(3):76–83.
Ranganathan, H., Chakraborty, S., and Panchanathan, S.
(2016). Multimodal emotion recognition using deep
learning architectures. In WACV, pages 1–9. IEEE.
Richardson, M., Agrawal, R., and Domingos, P. M. (2003).
Trust management for the Semantic Web. In ISWC,
pages 351–368.
Risnanto, S., Rahim, Y. B. A., and Herman, N. S. (2019).
Preparatory component for adoption e-voting. In
TSSA, pages 31–34. IEEE.
Salahdine, F. and Kaabouch, N. (2019). Social engineering
attacks: A survey. Future Internet, 11(4):89.
Schirmer, A. and Adolphs, R. (2017). Emotion perception
from face, voice, and touch: Comparisons and conver-
gence. Trends in cognitive sciences, 21(3):216–228.
Sengupta, J., Ruj, S., and Bit, S. D. (2020). A comprehen-
sive survey on attacks, security issues and blockchain
solutions for IoT and IIoT. Journal of Network and
Computer Applications, 149.
Sharma, A. and Sharma, T. (2017). HR analytics and perfor-
mance appraisal system. Management Research Re-
view.
Shen, M., Tang, X., Zhu, L., Du, X., and Guizani, M.
(2019). Privacy-preserving support vector machine
training over blockchain-based encrypted IoT data
in smart cities. IEEE Internet of Things Journal,
6(5):7702–7712.
Sur, P. and Cand
`
es, E. J. (2019). A modern maximum-
likelihood theory for high-dimensional logistic regres-
sion. PNAS, 116(29):14516–14525.
Tavakolifard, M., Almeroth, K. C., and Gulla, J. A. (2013).
Does social contact matter? modelling the hidden
Web of trust underlying Twitter. In WWW, pages 981–
988.
Terzi, D. S., Terzi, R., and Sagiroglu, S. (2017). Big data
analytics for network anomaly detection from netflow
data. In UBMK, pages 592–597. IEEE.
Ventocilla, E. (2019). Big data programming with Apache
Spark. In Data science in practice, pages 171–194.
Springer.
Wang, M. and Chen, H. (2020). Chaotic multi-swarm whale
optimizer boosted support vector machine for medical
diagnosis. Applied Soft Computing, 88.
Wang, S.-H., Phillips, P., Dong, Z.-C., and Zhang, Y.-D.
(2018). Intelligent facial emotion recognition based
on stationary wavelet entropy and Jaya algorithm.
Neurocomputing, 272:668–676.
Wu, S., Sanghavi, S., and Dimakis, A. G. (2019). Sparse lo-
gistic regression learns all discrete pairwise graphical
models. In NIPS, pages 8071–8081.
Xue, P., Li, B., Wang, N., and Zhu, T. (2019). Emotion
recognition from human gait features based on DCT
transform. In International Conference on Human
Centered Computing, pages 511–517. Springer.
DMMLACS 2020 - 1st International Special Session on Data Mining and Machine Learning Applications for Cyber Security
414