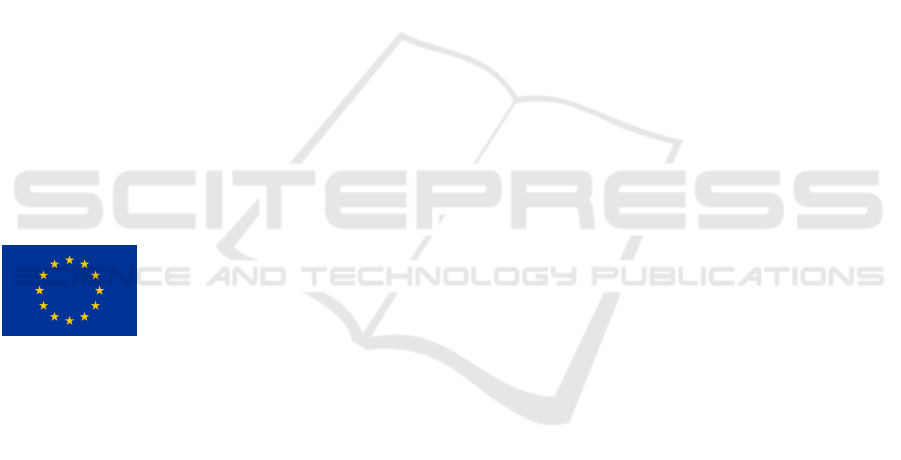
factors in modern retail, like recommender systems
(MacKenzie et al., 2013) and technologies that have
the potential to enrich customer experience like AR
(Papagiannidis et al., 2017).
To conclude, retailers needs to focus on solving
the customers’ problem, aiming to create a holistic
experience along the shopping journey. As creating
a shopping assistant which creates a more immersive
experience, and by leveraging machine learning tech-
niques can provide a more personalize in-store experi-
ence to the customers. These shopping assistant, such
as an XARSAA, can enhance the digital transforma-
tion of brick-and-mortar stores and thus, help the shift
in the physical environments in order to blur the per-
ception of channels for customers toward omnichan-
nel retail.
Therefore, as the retail sector moves forward, and
most retailers face challenges to keep up the competi-
tion, this study can help traditional brick-and-mortar
stores managers to create strategies, as well as it gives
insights for the practitioners who are working on the
transition toward the omnichannel model, in order to
strengthen their market position and become more re-
silient to online competition.
ACKNOWLEDGEMENT
This research is a part of the
European Training Network
project PERFORM that has
received funding from the Eu-
ropean Union’s Horizon 2020
research and innovation program under the Marie
Skodowska-Curie grant agreement No 765395. This
research reflects only the authors’ view, the European
Commission is not responsible for any use that may
be made of the information it contains.
REFERENCES
Adadi, A. and Berrada, M. (2018). Peeking inside the black-
box: A survey on explainable artificial intelligence
(xai). IEEE Access, 6:52138–52160.
Amatriain, X. and Basilico, J. (2015). Recommender sys-
tems in industry: A netflix case study. In Recom-
mender systems handbook, pages 385–419. Springer.
Antony, R., Khanapuri, V. B., and Jain, K. (2018). Customer
expectations and moderating role of demographics in
fresh food retail. International Journal of Retail &
Distribution Management.
Chen, X., Zhang, Y., and Qin, Z. (2019). Dynamic explain-
able recommendation based on neural attentive mod-
els. In Proceedings of the AAAI Conference on Artifi-
cial Intelligence, volume 33, pages 53–60.
Cheng, Z., Chang, X., Zhu, L., Kanjirathinkal, R. C.,
and Kankanhalli, M. (2019). Mmalfm: Explainable
recommendation by leveraging reviews and images.
ACM Transactions on Information Systems (TOIS),
37(2):1–28.
Cirqueira, D., Helfert, M., and Bezbradica, M. (2019a).
Towards preprocessing guidelines for neural network
embedding of customer behavior in digital retail. In
Proceedings of the 2019 3rd International Symposium
on Computer Science and Intelligent Control, pages
1–6.
Cirqueira, D., Hofer, M., Nedbal, D., Helfert, M., and
Bezbradica, M. (2019b). Customer purchase behav-
ior prediction in e-commerce: A conceptual frame-
work and research agenda. In International Workshop
on New Frontiers in Mining Complex Patterns, pages
119–136. Springer.
Cirqueira, D., Nedbal, D., Helfert, M., and Bezbrad-
ica, M. (2020). Scenario-based requirements elicita-
tion for user-centric explainable ai. In International
Cross-Domain Conference for Machine Learning and
Knowledge Extraction, pages 321–341. Springer.
Clickworker (2020a). Enterprise online survey software &
tools - surveygizmo.
Clickworker (2020b). Our crowd - the clickworkers.
Cohen, J. (1992). Quantitative methods in psychology: A
power primer. Psychol. Bull., 112:1155–1159.
Creedon, F. (2016). The framework for REVIEWS: an ex-
ploration into design principles for an electronic med-
ical early warning system observation chart. PhD the-
sis, University College Cork.
Gao, J., Wang, X., Wang, Y., and Xie, X. (2019). Ex-
plainable recommendation through attentive multi-
view learning. In Proceedings of the AAAI Conference
on Artificial Intelligence, volume 33, pages 3622–
3629.
Gregor, S. (2006). The nature of theory in information sys-
tems. MIS quarterly, pages 611–642.
Hamilton, K. (2009). Low-income families: experiences
and responses to consumer exclusion. International
journal of sociology and social policy.
Hausman, A. V. and Siekpe, J. S. (2009). The effect of
web interface features on consumer online purchase
intentions. Journal of business research, 62(1):5–13.
He, X., Chen, T., Kan, M.-Y., and Chen, X. (2015). Tri-
rank: Review-aware explainable recommendation by
modeling aspects. In Proceedings of the 24th ACM In-
ternational on Conference on Information and Knowl-
edge Management, pages 1661–1670.
Hoffman, R. R., Mueller, S. T., Klein, G., and Litman, J.
(2018). Metrics for explainable ai: Challenges and
prospects. arXiv preprint arXiv:1812.04608.
Huang, X., Fang, Q., Qian, S., Sang, J., Li, Y., and Xu,
C. (2019). Explainable interaction-driven user model-
ing over knowledge graph for sequential recommen-
dation. In Proceedings of the 27th ACM International
Conference on Multimedia, pages 548–556.
IBM (2020). Ibm spss statistics 26.
WUDESHI-DR 2020 - Special Session on User Decision Support and Human Interaction in Digital Retail
316