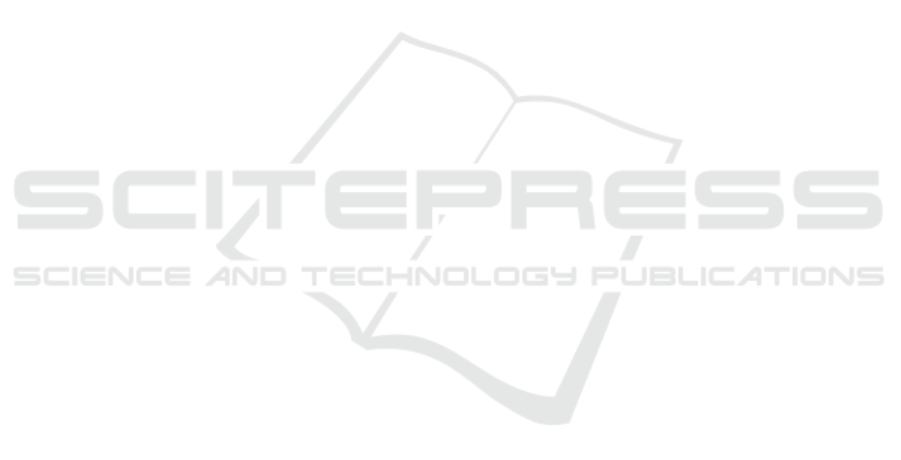
tion for user-centric explainable ai. In International
Cross-Domain Conference for Machine Learning and
Knowledge Extraction, pages 321–341. Springer.
Cirqueira, D., Pinheiro, M., Braga, T., Jacob Jr, A., Rein-
hold, O., Alt, R., and Santana,
´
A. (2017). Improv-
ing relationship management in universities with sen-
timent analysis and topic modeling of social media
channels: learnings from ufpa. In Proceedings of the
International Conference on Web Intelligence, pages
998–1005.
Cirqueira, D., Pinheiro, M. F., Jacob, A., Lobato, F., and
Santana, A. (2018). A literature review in prepro-
cessing for sentiment analysis for brazilian portuguese
social media. In 2018 IEEE/WIC/ACM International
Conference on Web Intelligence (WI), pages 746–749.
IEEE.
de Almeida, G. R., Cirqueira, D. R., and Lobato, F. M.
(2017). Improving social crm through eletronic word-
of-mouth: a case study of reclameaqui. In Anais
Estendidos do XXIII Simp
´
osio Brasileiro de Sistemas
Multim
´
ıdia e Web, pages 107–110. SBC.
Donthu, N. and Gustafsson, A. (2020). Effects of covid-
19 on business and research. Journal of business re-
search, 117:284.
Doshi-Velez, F. and Kim, B. (2017). Towards a rigorous sci-
ence of interpretable machine learning. arXiv preprint
arXiv:1702.08608.
Ehsan, U., Purdy, C., Kelley, C., Polepeddi, L., and Davis,
N. Unpack that tweet: A traceable and interpretable
cognitive modeling system.
Hasan, A., Moin, S., Karim, A., and Shamshirband, S.
(2018). Machine learning-based sentiment analysis
for twitter accounts. Mathematical and Computa-
tional Applications, 23(1):11.
Hase, P. and Bansal, M. (2020). Evaluating explainable
ai: Which algorithmic explanations help users predict
model behavior? arXiv preprint arXiv:2005.01831.
Holzinger, A., Carrington, A., and M
¨
uller, H. (2020). Mea-
suring the quality of explanations: the system caus-
ability scale (scs). KI-K
¨
unstliche Intelligenz, pages
1–6.
Honegger, M. (2018). Shedding light on black box ma-
chine learning algorithms: Development of an ax-
iomatic framework to assess the quality of methods
that explain individual predictions. arXiv preprint
arXiv:1808.05054.
Hu, G., Bhargava, P., Fuhrmann, S., Ellinger, S., and Spaso-
jevic, N. (2017). Analyzing users’ sentiment towards
popular consumer industries and brands on twitter. In
2017 IEEE International Conference on Data Mining
Workshops (ICDMW), pages 381–388. IEEE.
Islam, T. (2019). Ex-twit: Explainable twitter mining on
health data. arXiv preprint arXiv:1906.02132.
Jabreel, M. and Moreno, A. (2018). Eitaka at semeval-2018
task 1: An ensemble of n-channels convnet and xg-
boost regressors for emotion analysis of tweets. arXiv
preprint arXiv:1802.09233.
James, E. and WOOTEN, L. (2005). Leadership as
(un)usual:: How to display competence in times of
crisis. Organizational Dynamics, 34:141–152.
Jansen, B. J., Zhang, M., Sobel, K., and Chowdury, A.
(2009). Twitter power: Tweets as electronic word of
mouth. Journal of the American society for informa-
tion science and technology, 60(11):2169–2188.
Krouska, A., Troussas, C., and Virvou, M. (2016). The
effect of preprocessing techniques on twitter senti-
ment analysis. In 2016 7th International Conference
on Information, Intelligence, Systems & Applications
(IISA), pages 1–5. IEEE.
Liu, B. (2012). Sentiment analysis and opinion mining.
Synthesis lectures on human language technologies,
5(1):1–167.
Liu, X. (2019). A big data approach to examining social
bots on twitter. Journal of Services Marketing.
Lobato, F., Pinheiro, M., Jacob, A., Reinhold, O., and San-
tana,
´
A. (2016). Social crm: Biggest challenges to
make it work in the real world. In International Con-
ference on Business Information Systems, pages 221–
232. Springer.
Lundberg, S. M. and Lee, S.-I. (2017). A unified approach
to interpreting model predictions. In Advances in neu-
ral information processing systems, pages 4765–4774.
Luo, F., Li, C., and Cao, Z. (2016). Affective-feature-
based sentiment analysis using svm classifier. In 2016
IEEE 20th International Conference on Computer
Supported Cooperative Work in Design (CSCWD),
pages 276–281. IEEE.
Mathews, S. M. (2019). Explainable artificial intelligence
applications in nlp, biomedical, and malware classifi-
cation: A literature review. In Intelligent Computing-
Proceedings of the Computing Conference, pages
1269–1292. Springer.
Medhat, W., Hassan, A., and Korashy, H. (2014). Sentiment
analysis algorithms and applications: A survey. Ain
Shams engineering journal, 5(4):1093–1113.
Mehta, S., Saxena, T., and Purohit, N. (2020). The new
consumer behaviour paradigm amid covid-19: Perma-
nent or transient? Journal of Health Management,
22(2):291–301.
Micu, A., Micu, A. E., Geru, M., and Lixandroiu, R. C.
(2017). Analyzing user sentiment in social media: Im-
plications for online marketing strategy. Psychology &
Marketing, 34(12):1094–1100.
Miller, T. (2019). Explanation in artificial intelligence: In-
sights from the social sciences. Artificial Intelligence,
267:1–38.
Molnar, C. (2019). Interpretable machine learning. Lulu.
com.
Nguyen, D. (2018). Comparing automatic and human eval-
uation of local explanations for text classification.
In Proceedings of the 2018 Conference of the North
American Chapter of the Association for Computa-
tional Linguistics: Human Language Technologies,
Volume 1 (Long Papers), pages 1069–1078.
Pagolu, V. S., Reddy, K. N., Panda, G., and Majhi, B.
(2016). Sentiment analysis of twitter data for pre-
dicting stock market movements. In 2016 interna-
tional conference on signal processing, communica-
tion, power and embedded system (SCOPES), pages
1345–1350. IEEE.
Explainable Sentiment Analysis Application for Social Media Crisis Management in Retail
327