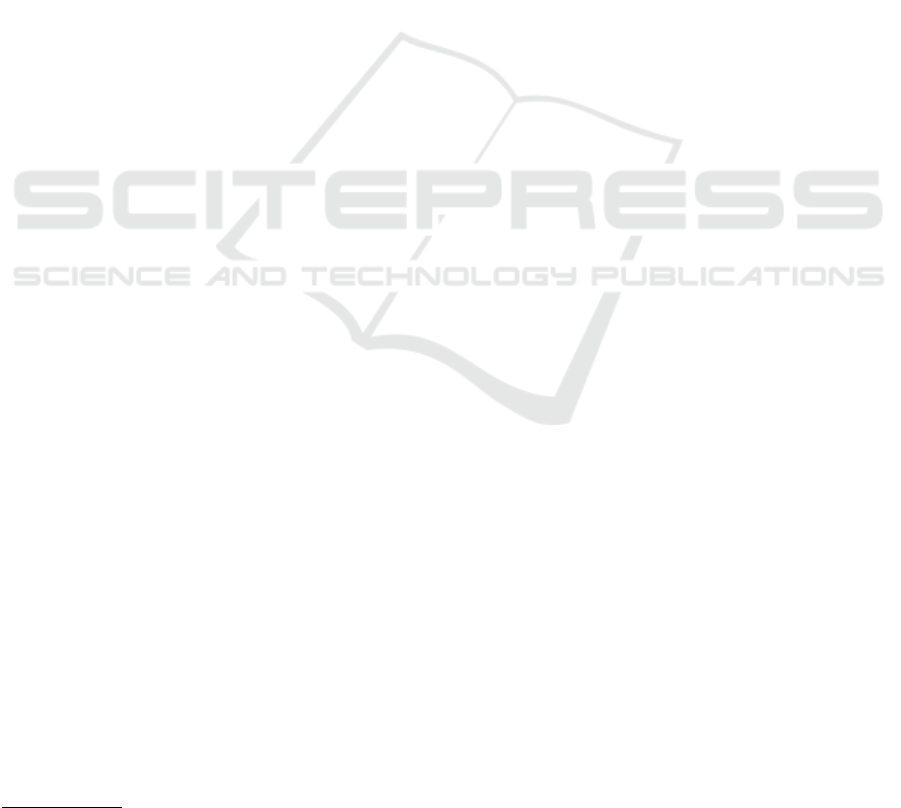
In 2014, there were only 20 reviews, while 3, 931
reviews were posted in 2015, 11, 709 in 2016 and
17, 305 in 2017 (up to the end of September). That
is, AHS experienced a high user growth.
A category has on average 94.73 reviews and a
provider has 7.11 reviews on average. Providers sell
on average in 3.52 categories, while categories have
on average 13.31 providers having received reviews.
Categories have up to 1, 338 reviews. Providers serve
up to 78 categories.
When it comes to the star ratings on AHS, it can
be said that they are overwhelmingly positive, hav-
ing on average 4.76 stars. This is interesting as it is
consistent with a study finding that quantitative rat-
ings are on average positive (Chevalier and Mayzlin,
2006). In general, the distribution of star ratings is un-
equal. The absolute majority of reviews is rated with
five stars: 29, 305. Four stars were given to 1, 813 re-
views, three to 665, two to 411 and one star was given
to 771 reviews in total.
When taking into consideration the data from the
analysis table, there are findings to be made. The data
consist of the combinations of every provider and ev-
ery category he has received reviews in. That is, for
example, a provider with reviews in ten different cat-
egories would appear in ten data rows. Such com-
binations are from now on called provider-category
combinations.
There are 16, 306 provider-category combinations
in total. Further, there are 11, 307 combinations with
one review, 2, 718 with two reviews and 2, 551 with
three or more reviews. Thus, there are many providers
having served and received reviews in several cate-
gories only once. There are 2.02 reviews on average
per combination.
For research question five, it is interesting to know
how many providers serve in one, two etc. cate-
gories. While the average lies at 3.52 categories per
provider, taking all provider-category combinations
even with less than three reviews into consideration,
2, 262 providers have just one category, 814 have two
and 407 have three categories. The number drops fur-
ther, e.g. there are only 43 providers with ten cate-
gories. The number quickly becomes single-digit.
Regarding the time at which reviews were given,
between the first and last review of the provider-
category combinations are on average 242 days, i.e.
8 months
6
. While there is a combination which re-
ceived all three reviews on the same day, the maxi-
mal distance between the first and last review is 1, 019
days, i.e. 2 years and 10 months.
When it comes to the sentiment scores, there are
different interesting information to be found: On av-
6
To simplify the calculation, the months have 30 days.
erage, the About Me texts and reviews are ca. two
thirds positive. That is, About Me texts are about
69.6% positive and 30.4% negative. Similar values
can be found concerning the average sentiment scores
or reviews. They are 22.2% negative and 77.8% pos-
itive.
The next Sections answer the research questions.
4.2 Q1: Performance of the Best Rated
Categories over Time
This Section develops answers to Q1. For dealing
with the research questions of this paper, the acquired
and aggregated data were further processed. Then,
line diagrams were developed. The first research
question asks for a comparison of the best rated cat-
egories of providers with the other categories over
time. Thus, there is a comparison of the best with
the next three best categories per every provider.
The best and most rated categories of this Section
are those who overall, at the end of the period con-
sidered, have the best and most ratings overall (aver-
age/total number of all ratings regardless of the time
point).
The data of this paper, as Figure 1 demonstrates,
are separated into ten relative time intervals which
represent ten percent each (x-axis). Further, this paper
uses average star ratings (y-axis). That is, the diagram
provides an overview of the best rated categories in
the form of their average ratings over time.
Every review was assigned to a relative time point.
Thus, these show at which time the review was given
relative to the other reviews of a provider in the
corresponding category. An example is a provider
who received three reviews in the category “Row-
ing Machine Assembly” on the following dates: 10th
Febuary 2017, 21st Feburary 2017 and 30th February
2017. Here, one time interval (a tenth) is two days
long. The first date would mark the beginning of the
time (zero in the diagram), the second date is close to
the middle (60), while the third review marks the last
possible time point (100). As the second review falls
into the interval between two time points (50 and 60),
it is categorized as the latter, i.e. 60.
In the end, all best, second-best etc. categories’
ratings are averaged for every time point. This way of
building the diagram is used for all other figures in the
following Sections of this Section. Of course, it was
adapted according to the current necessities. When
there were two best rated categories with the same star
rating, one was randomly chosen, as a combination
of those delivered an unsatisfying picture. All above
remarks apply to the most rated categories, too.
What Reviews in Local Online Labour Markets Reveal about the Performance of Multi-service Providers
267