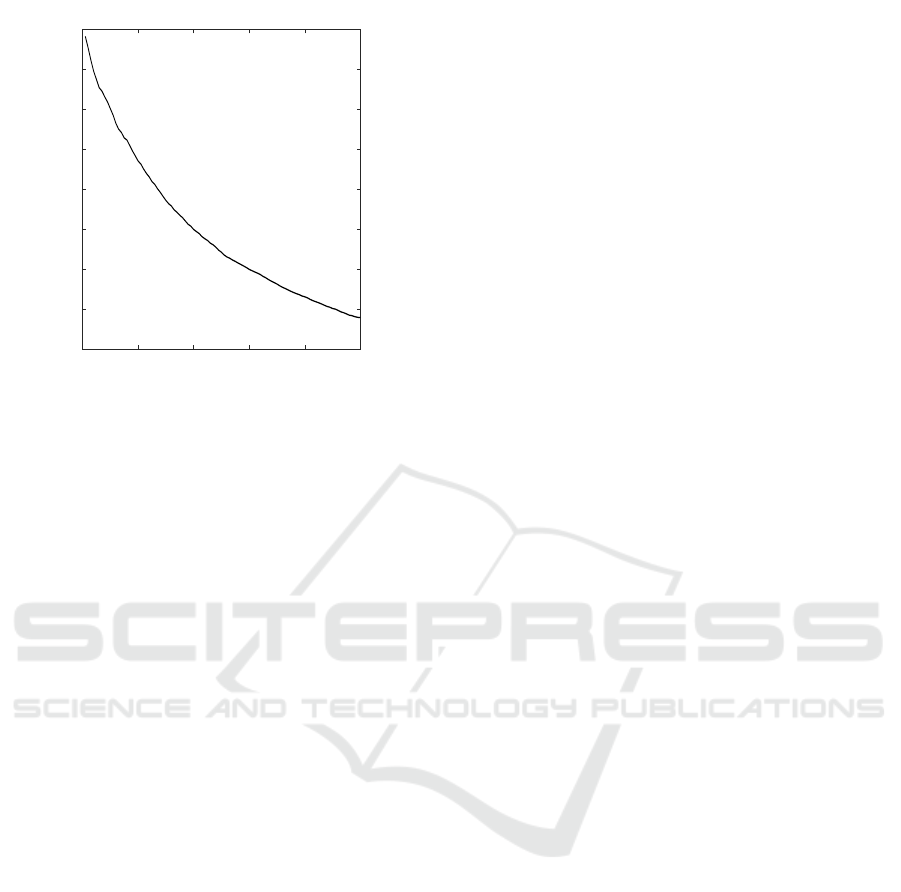
0 20 40 60 80 100
top x%
0.15
0.2
0.25
0.3
0.35
0.4
0.45
0.5
0.55
AUPR
Figure 5: Average AUPR for inferred networks of reliable
top x% edges.
posed method can translate a problem to use other es-
timators of residuals ε, η and independence measures
instead of HKRR and J. The score of p-value offered
by the permutation test can be interpreted as the sig-
nificance level, and this threshold is easier to deter-
mine than other scores such as the conditional mutual
information. Additionally, the reliability of p-value is
defined.
The proposed method was applied to inferring
artificial gene networks from the Dream4 challenge
datasets, and had the better performance in terms of
AUROC and AUPR. Although the proposed method
infers an part of network by omitting low reliable
p-values, there an advantage to find unknown asso-
ciations. Furthermore, merging parts of network of
high reliable p-values inferred by various estimators
would be a promising strategy to identify whole net-
work structure.
The proposed method would be basically ex-
tended to the inference of associative networks in case
that the dataset is many-dimensional, that is, X and
Y can be multidimensional. This method may prove
useful, for instance, in the examination of associations
between layers or pathways in transomics datasets.
The HKRR method is employed to infer ε and η in
the method proposed above. However, if p(x|Z) or
p(y|Z) can have a multi-modal distribution for some
fixed Z, HKRR is no longer suitable. The appropriate
method for inferring ε and η depends on the distri-
bution of the data in question, and demands further
study.
ACKNOWLEDGEMENTS
This work was supported by the Creating informa-
tion utilization platform by integrating mathemati-
cal and information sciences, and development to so-
ciety, CREST (JPMJCR1912) from the Japan Sci-
ence and Technology Agency (JST) and by the Japan
Society for the Promotion of Science (JSPS) KAK-
ENHI Grant Number (JP18H04801, JP18H02431)
and Kayamori Foundation of Informational Science
Advancement.
REFERENCES
Cawley, G. and Talbota, N. (2004). Fast exact leave-one-out
cross-validation of sparse least-squares support vector
machines. Neural Networks, 17:1467–1475.
Cawley, G. C., Talbota, N., Foxalla, R. J., Dorlingb, S. R.,
and Mandic, D. P. (2004). Heteroscedastic kernel
ridge regression. Neurocomputing, 57:105–124.
Cover, T. M. and Thomas, J. (2006). Elements of Informa-
tion Theory. Wiley-Interscience New York, NY, USA,
2nd edition.
Daub, C. O., Steuer, R., Selbig, J., and Kloska, S.
(2004). Estimating mutual information using b-
spline functions–an improved similarity measure for
analysing gene expression data. BMC bioinformatics,
5(1):118.
Davis, J. and Goadrich, M. (2006). The relationship be-
tween precision-recall and roc curves. In Proceed-
ings of the 23th international conference on Machine
learning (ICML’06), pages 233–240.
Edgington, E. and Onghena, P. (2007). Randomization
Tests. Chapman and Hall/CRC, 4th edition.
Faith, J., Hayete, B., Thaden, J., Mogno, I., Wierzbowski,
J., Cottarel, G., Kasif, S., Collins, J., and Gardner,
T. (2007). Large-scale mapping and validation of es-
cherichia coli transcriptional regulation from a com-
pendium of expression profiles. PLoS Biol., 5(1):e8.
Guo, S., Jiang, Q., Chen, L., and Guo, D. (2016). Gene reg-
ulatory network inference using pls-based methods.
BMC Bioinformatics, 17(545).
Hastie, T., Tibshirani, R., and Friedman, J. (2001). The
Elements of Statistical Learning. Springer New York
Inc, NY, USA.
Haury, A.-C., Mordelet, F., Vera-Licona, P., and Vert, J.-
P. (2012). Tigress: trustful inference of gene regula-
tion using stability selection. BMC systems biology,
6(1):145.
Irrthum, A., Wehenkel, L., Geurts, P., et al. (2010). Inferring
regulatory networks from expression data using tree-
based methods. PloS one, 5(9):e12776.
Margolin, A., Nemenman, I., Basso, K., Wiggins, C.,
Stolovitzky, G., Favera, R., and Califano, A. (2006).
Aracne: An algorithm for the reconstruction of gene
regulatory networks in a mammalian cellular context.
BMC Bioinformatics, 7(57).
Approximate Conditional Independence Test using Residuals
303