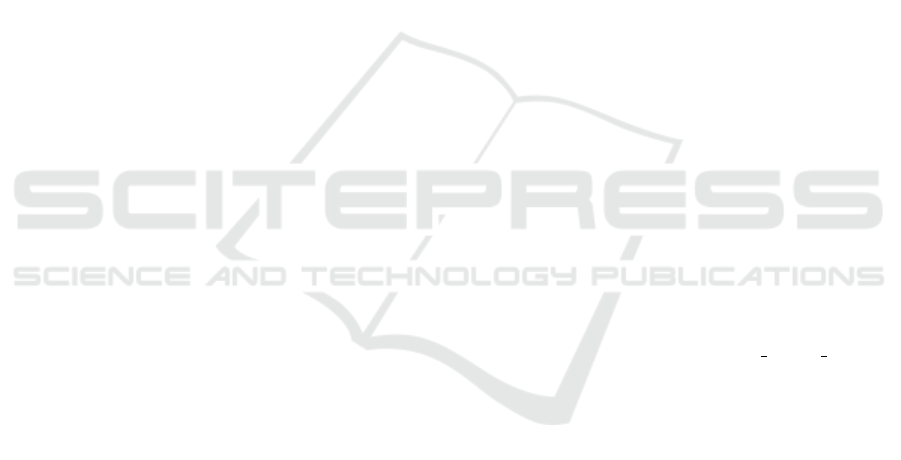
gies and given birth to new approaches such as Prog-
nostics and Health Management (PHM). At the same
time, data management has also become a challenge
that can be neglected no more. As we believe that
ES are relevant solutions for implementing PHM so-
lutions due to their ability to capitalize and process
available knowledge on a systems and its failures, we
propose to combine this systems with CEP solutions.
The purpose of using CEP is to filter to flow of input
data in order to detect the relevant events for further
analysis. In order to match these systems we pro-
pose to implement a model transformation approach
to extract knowledge from the ES knowledge base
and transform it into CEP rules. This transformation
is divided in two steps. The first phase consists in
transforming the relevant concepts of the knowledge
base into generic rules. The second phase transforms
these generic rules into CEP rules conforming to the
Event Processing Language. The purpose of defining
generic rules lies in the improved flexibility granted
to the transformation and the possibility to perform 1-
to-n transformations between these generic rules and
EQL rules.
The limit of this approach is the current lack of
computing implementation in a real case, which our
future work will focus on.
REFERENCES
AFNOR (2018). NF EN 13306 - Maintenance — Termi-
nologie de la maintenance.
Belaunde, M., Casanave, C., DSouza, D., Duddy, K.,
El Kaim, W., Kennedy, A., Frank, W., Frankel, D.,
Hauch, R., and Hendryx, S. (2003). MDA Guide Ver-
sion 1.0. 1.
Bezivin, J. and Briot, J.-P. (2004). Sur les principes de base
de l’ing
´
enierie des mod
`
eles. L’OBJET, 10(4):145–
157.
Bezivin, J., B
¨
uttner, F., Gogolla, M., Jouault, F., Kurtev,
I., and Lindow, A. (2006). Model Transformations?
Transformation Models! In Nierstrasz, O., Whittle,
J., Harel, D., and Reggio, G., editors, Model Driven
Engineering Languages and Systems, Lecture Notes
in Computer Science, pages 440–453. Springer Berlin
Heidelberg.
Bezivin, J. and Gerbe, O. (2001). Towards a precise defi-
nition of the OMG/MDA framework. In Proceedings
16th Annual International Conference on Automated
Software Engineering (ASE 2001), pages 273–280.
Blanchard, B. S., Verma, D. C., and Peterson, E. L. (1995).
Maintainability : a key to effective serviceability and
maintenance management. New York : John Wiley &
Sons, Inc.
Boubeta-Puig, J., Ortiz, G., and Medina-Bulo, I. (2014).
A model-driven approach for facilitating user-friendly
design of complex event patterns. Expert Systems with
Applications, 41(2):445–456.
DePold, H. R. and Gass, F. D. (1999). The Application of
Expert Systems and Neural Networks to Gas Turbine
Prognostics and Diagnostics. Journal of Engineering
for Gas Turbines and Power, 121(4):607–612.
Gertler, J. (1998). Fault Detection and Diagnosis in En-
gineering Systems. CRC Press. Google-Books-ID:
fmPyTbbqKFIC.
Guillen, A. J., Crespo, A., G
´
omez, J. F., and Sanz, M. D.
(2016). A framework for effective management of
condition based maintenance programs in the context
of industrial development of E-Maintenance strate-
gies. Computers in Industry, 82:170–185.
ISO (2012). ISO 13372, Surveillance et diagnostic des ma-
chines — Vocabulaire.
ISO (2017). NF EN ISO 14224 - Petroleum, petrochemical
and natural gas industries - Collection and exchange
of reliability and maintenance data for equipment.
Jackson, P. (1998). Introduction to Expert Systems.
Addison-Wesley Longman Publishing Co., Inc.,
Boston, MA, USA, 3rd edition.
Jardine, A. K. S., Lin, D., and Banjevic, D. (2006). A re-
view on machinery diagnostics and prognostics im-
plementing condition-based maintenance. Mechani-
cal Systems and Signal Processing, 20(7):1483–1510.
Jin, X., Wah, B. W., Cheng, X., and Wang, Y. (2015). Sig-
nificance and Challenges of Big Data Research. Big
Data Research, 2(2):59–64.
Kalogirou, S. A. (2003). Artificial intelligence for the
modeling and control of combustion processes: a re-
view. Progress in Energy and Combustion Science,
29(6):515–566.
Lebold, M., Reichard, K., and Boylan, D. (2003). Uti-
lizing dcom in an open system architecture frame-
work for machinery monitoring and diagnostics. In
2003 IEEE Aerospace Conference Proceedings (Cat.
No.03TH8652), volume 3, pages 3 1227–3 1236.
Lee, J., Jin, C., Liu, Z., and Ardakani, H. D. (2017). Intro-
duction to data-driven methodologies for prognostics
and health management. In Probabilistic prognostics
and health management of energy systems, pages 9–
32. Springer.
Lee, J., Wu, F., Zhao, W., Ghaffari, M., Liao, L., and Siegel,
D. (2014). Prognostics and health management design
for rotary machinery systems—Reviews, methodol-
ogy and applications. Mechanical Systems and Signal
Processing, 42(1):314–334.
Levine, P. and Pomerol, J.-C. (1990). Syst
`
emes interactifs
d’aide
`
a la d
´
ecision et syst
`
emes experts. Herm
`
es.
Liebowitz, J. (1995). Expert systems: A short introduction.
Engineering Fracture Mechanics, 50(5):601–607.
Truptil, S., B
´
enaben, F., Salatge, N., Hanachi, C., Cha-
purlat, V., Pignon, J.-P., and Pingaud, H. (2010). Me-
diation Information System Engineering for Interoper-
ability Support in Crisis Management. In Popplewell,
K., Harding, J., Poler, R., and Chalmeta, R., edi-
tors, Enterprise Interoperability IV, pages 187–197.
Springer London.
Vachtsevanos, G., Lewis, F., Roemer, M., Hess, A., and Wu,
B. (2006). Systems Approach to CBM/PHM. In Intel-
MODELSWARD 2020 - 8th International Conference on Model-Driven Engineering and Software Development
214