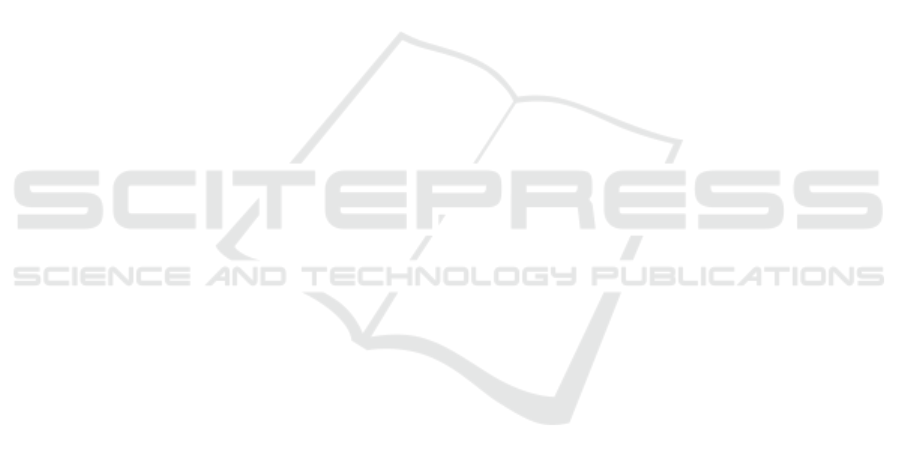
were found upon building. Although we achieved to
built valid schemas, a lot more remains to be done,
meaning that Winventor still has a lot of room for im-
provement. In particular, our analysis indicates that
further gains could be achieved via more accurate se-
mantic analysis of each sentence. Additionally, the
use of other techniques like viewing the an anaphora
resolution problem as a pointing problem might help
to the selection of better pronoun targets for every de-
veloped schema (Lee et al., 2017).
Future studies will have to identify mechanisms
through which we can develop large amounts of high
quality schemas. Among possible directions we have
the automation of the schema validation process with
the use of crowdworkers for further processing. An
updated version of Winventor that will act as the col-
laboration platform for the crowd, on one side, and
experts, on the other side, might drive us to a more
efficient way to produce large amounts of fruitful
schemas in the shortest time possible.
ACKNOWLEDGMENTS
This work was supported by funding from the EU’s
Horizon 2020 Research and Innovation Programme
under grant agreements no. 739578 and no. 823783,
and from the Government of the Republic of Cyprus
through the Directorate General for European Pro-
grammes, Coordination, and Development. The au-
thors would like to thank Ernest Davis for sharing his
thoughts and suggestions on this line of research.
REFERENCES
Amsili, P. and Seminck, O. (2017). A Google-Proof Collec-
tion of French Winograd Schemas. In Proceedings of
the 2nd Workshop on Coreference Resolution Beyond
OntoNotes (CORBON 2017), pages 24–29.
Antunes, J., Lins, R. D., Lima, R., Oliveira, H., Riss,
M., and Simske, S. J. (2018). Automatic Cohesive
Summarization with Pronominal Anaphora Resolu-
tion. Computer Speech & Language, 52:141–164.
Budukh, T. U. (2013). An Intelligent Co-reference Resolver
for Winograd Schema Sentences Containing Resolved
Semantic Entities. Master’s thesis, Arizona State Uni-
versity.
Deepa, K. A. and Deisy, C. (2017). Statistical Pair Pruning
Towards Target Class in Learning-Based Anaphora
Resolution for Tamil. International Journal of Ad-
vanced Intelligence Paradigms, 9(5-6):437–463.
Heilman, M. and Smith, N. A. (2009). Question Gener-
ation via Overgenerating Transformations and Rank-
ing. Technical report, Carnegie-Mellon Univ Pitts-
burgh Pa Language Technologies Inst.
Huddleston, R. and Pullum, G. (2005). The Cambridge
Grammar of the English Language. Zeitschrift f
¨
ur An-
glistik und Amerikanistik, 53(2):193–194.
Isaak, N. and Michael, L. (2016). Tackling the Winograd
Schema Challenge Through Machine Logical Infer-
ences. In Pearce, D. and Pinto, H. S., editors, STAIRS,
volume 284 of Frontiers in Artificial Intelligence and
Applications, pages 75–86. IOS Press.
Isaak, N. and Michael, L. (2018). Using the Winograd
Schema Challenge as a CAPTCHA. In Lee, D., Steen,
A., and Walsh, T., editors, GCAI-2018. 4th Global
Conference on Artificial Intelligence, volume 55 of
EPiC Series in Computing, pages 93–106. EasyChair.
Isaak, N. and Michael, L. (2019). WinoFlexi: A Crowd-
sourcing Platform for the Development of Winograd
Schemas. In Liu, J. and Bailey, J., editors, AI 2019:
Advances in Artificial Intelligence, pages 289–302,
Cham. Springer International Publishing.
Kocijan, V., Cretu, A.-M., Camburu, O.-M., Yordanov, Y.,
and Lukasiewicz, T. (2019). A Surprisingly Robust
Trick for Winograd Schema Challenge. arXiv preprint
arXiv:1905.06290.
Lee, C., Jung, S., and Park, C.-E. (2017). Anaphora Res-
olution with Pointer Networks. Pattern Recognition
Letters, 95:1–7.
Levesque, H., Davis, E., and Morgenstern, L. (2012). The
Winograd Schema Challenge. In Thirteenth Inter-
national Conference on the Principles of Knowledge
Representation and Reasoning.
Levesque, H. J. (2014). On Our Best behaviour. Artificial
Intelligence, 212:27–35.
Michael, L. (2013). Machines with Websense. In Proc. of
11th International Symposium on Logical Formaliza-
tions of Commonsense Reasoning (Commonsense 13).
Mitkov, R. (1998). Robust Pronoun Resolution with Lim-
ited Knowledge . In Proceedings of the 17th in-
ternational conference on Computational linguistics-
Volume 2, pages 869–875. Association for Computa-
tional Linguistics.
Morgenstern, L., Davis, E., and Ortiz, C. L. (2016).
Planning, Executing, and Evaluating the Winograd
Schema Challenge. AI Magazine, 37(1):50–54.
Rahman, A. and Ng, V. (2012). Resolving Complex Cases
of Definite Pronouns: The Winograd Schema Chal-
lenge. In Proceedings of the 2012 Joint Conference
on Empirical Methods in Natural Language Process-
ing and Computational Natural Language Learning,
EMNLP-CoNLL ’12, pages 777–789, Stroudsburg,
PA, USA. Association for Computational Linguistics.
Seely, J. (2013). Oxford Guide to Effective Writing and
Speaking: How to Communicate Clearly. OUP Ox-
ford.
Sharma, A., Vo, N. H., Aditya, S., and Baral, C. (2015). To-
wards Addressing the Winograd Schema Challenge -
Building and Using a Semantic Parser and a Knowl-
edge Hunting Module. In Proceedings of the Twenty-
Fourth International Joint Conference on Artificial In-
telligence, IJCAI, pages 25–31.
Winventor: A Machine-driven Approach for the Development of Winograd Schemas
35