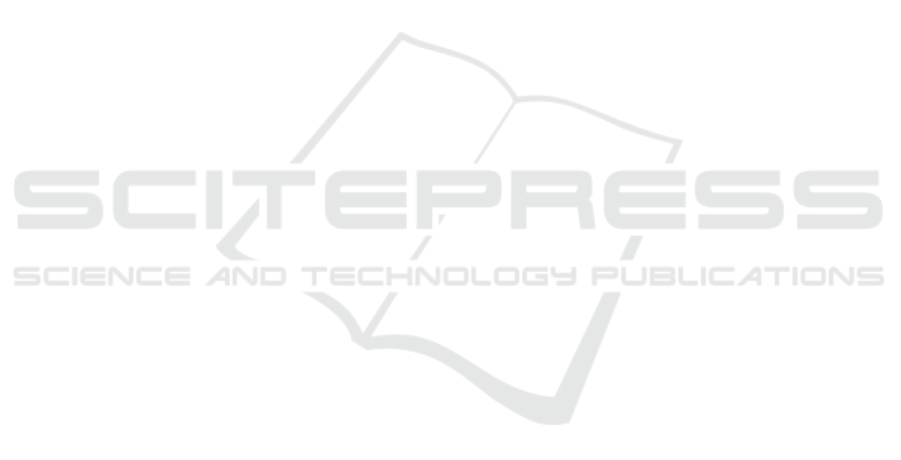
Jafari, H., Courtois, I., Van den Bergh, O., Vlaeyen, J. W.,
and Van Diest, I. (2017). Pain and respiration: a sys-
tematic review. Pain, 158(6):995–1006.
K
¨
achele, M., Thiam, P., Amirian, M., Schwenker, F., and
Palm, G. (2016). Methods for person-centered contin-
uous pain intensity assessment from bio-physiological
channels. IEEE Journal of Selected Topics in Signal
Processing, 10(5):854–864.
Kim, J. and Andr
´
e, E. (2008). Emotion recognition based on
physiological changes in music listening. IEEE Trans-
actions on Pattern Analysis and Machine Intelligence,
30(12):2067–2083.
Kingma, D. and Ba, J. (2014). Adam: A method for
stochastic optimization. International Conference on
Learning Representations.
Kiranyaz, S., Avci, O., Abdeljaber, O., Ince, T., Gabbouj,
M., and Inman, D. J. (2019). 1d convolutional neural
networks and applications: A survey.
Ledowski, T., Ang, B., Schmarbeck, T., and Rhodes, J.
(2009). Monitoring of sympathetic tone to assess post-
operative pain: skin conductance vs surgical stress in-
dex. Anaesthesia, 64(7):727–731.
Loggia, M. L., Juneau, M., and Bushnell, M. C. (2011).
Autonomic responses to heat pain: Heart rate, skin
conductance, and their relation to verbal ratings and
stimulus intensity. PAIN, 152(3):592 – 598.
Lu, L., Zhang, H.-J., and Li, S. Z. (2003). Content-based au-
dio classification and segmentation by using support
vector machines. Multimedia systems, 8(6):482–492.
Lucey, P., Cohn, J. F., Prkachin, K. M., Solomon, P. E., and
Matthews, I. (2011). Painful data: The unbc-mcmaster
shoulder pain expression archive database. In Face
and Gesture 2011, pages 57–64.
Mallik, S., Chowdhury, D., and Chttopadhyay, M. (2019).
Development and performance analysis of a low-cost
mems microphone-based hearing aid with three dif-
ferent audio amplifiers. Innovations in Systems and
Software Engineering, 15(1):17–25.
¨
Ohman, A., Esteves, F., Flykt, A., and Soares, J. J. F. (1993).
Gateways to Consciousness: Emotion, Attention, and
Electrodermal Activity, pages 137–157. Springer US,
Boston, MA.
Petroni, M., Malowany, A. S., Johnston, C. C., and Stevens,
B. J. (1995). Identification of pain from infant cry
vocalizations using artificial neural networks (anns).
In Applications and Science of Artificial Neural Net-
works, volume 2492, pages 729–739. International
Society for Optics and Photonics.
Picard, R. W. (2000). Emotions Are Physical and Cognitive,
pages 21–45. MIT press, Cambridge, MA.
Saki, F. and Kehtarnavaz, N. (2014). Background noise
classification using random forest tree classifier for
cochlear implant applications. In 2014 IEEE Inter-
national Conference on Acoustics, Speech and Signal
Processing (ICASSP), pages 3591–3595. IEEE.
Schwenker, F. (2018). Multimodal affect classifica-
tion using deep neural networks. Studientexte
zur Sprachkommunikation: Elektronische Sprachsig-
nalverarbeitung 2018, pages 240–246.
Sellner, J., Thiam, P., and Schwenker, F. (2018). Visualizing
facial expression features of pain and emotion data. In
IAPR Workshop on Multimodal Pattern Recognition of
Social Signals in Human-Computer Interaction, pages
101–115. Springer.
Simonyan, K. and Zisserman, A. (2014). Very deep con-
volutional networks for large-scale image recognition.
arXiv preprint arXiv:1409.1556.
Srivastava, N., Hinton, G., Krizhevsky, A., Sutskever, I.,
and Salakhutdinov, R. (2014). Dropout: a simple way
to prevent neural networks from overfitting. The Jour-
nal of Machine Learning Research, 15(1):1929–1958.
Thiam, P., Kessler, V., Amirian, M., Bellmann, P., Layher,
G., Zhang, Y., Velana, M., Gruss, S., Walter, S., Traue,
H. C., Kim, J., Schork, D., Andre, E., Neumann, H.,
and Schwenker, F. (2019). Multi-modal pain inten-
sity recognition based on the senseemotion database.
IEEE Transactions on Affective Computing.
Thiam, P., Kessler, V., and Schwenker, F. (2017). Hierar-
chical combination of video features for personalised
pain level recognition. In ESANN.
Thiam, P. and Schwenker, F. (2017). Multi-modal data fu-
sion for pain intensity assessment and classification.
In 2017 Seventh International Conference on Image
Processing Theory, Tools and Applications (IPTA),
pages 1–6. IEEE.
Turk, D. C. and Gatchel, R. J. (2018). Psychological ap-
proaches to pain management: A practitioner’s hand-
book. Guilford publications.
Turk, D. C., Meichenbaum, D., and Genest, M. (1983). Pain
and behavioral medicine: A cognitive-behavioral per-
spective, volume 1. Guilford Press.
Velana, M., Gruss, S., Layher, G., Thiam, P., Zhang, Y.,
Schork, D., Kessler, V., Meudt, S., Neumann, H.,
Kim, J., Schwenker, F., Andr
´
e, E., Traue, H. C., and
Walter, S. (2016). The senseemotion database: A
multimodal database for the development and system-
atic validation of an automatic pain- and emotion-
recognition system. In MPRSS 2016, pages 127–139.
Wagner, J., Lingenfelser, F., Baur, T., Damian, I., Kistler,
F., and Andr
´
e, E. (2013). The social signal interpre-
tation (ssi) framework: Multimodal signal processing
and recognition in real-time. In Proceedings of the
21st ACM International Conference on Multimedia,
MM ’13, pages 831–834, New York, NY, USA. ACM.
Walter, S., Gruss, S., Ehleiter, H., Junwen Tan, Traue, H. C.,
Werner, P., Al-Hamadi, A., Crawcour, S., Andrade,
A. O., and Moreira da Silva, G. (2013). The biovid
heat pain database data for the advancement and sys-
tematic validation of an automated pain recognition
system. In 2013 IEEE International Conference on
Cybernetics (CYBCO), pages 128–131.
Wang, J.-C., Lee, H.-P., Wang, J.-F., and Lin, C.-B. (2008).
Robust environmental sound recognition for home au-
tomation. IEEE Transactions on Automation Science
and Engineering, 5(1):25–31.
Zwakhalen, S. M., Hamers, J. P., Abu-Saad, H. H., and
Berger, M. P. (2006). Pain in elderly people with
severe dementia: a systematic review of behavioural
pain assessment tools. BMC geriatrics, 6(1):3.
ICPRAM 2020 - 9th International Conference on Pattern Recognition Applications and Methods
150