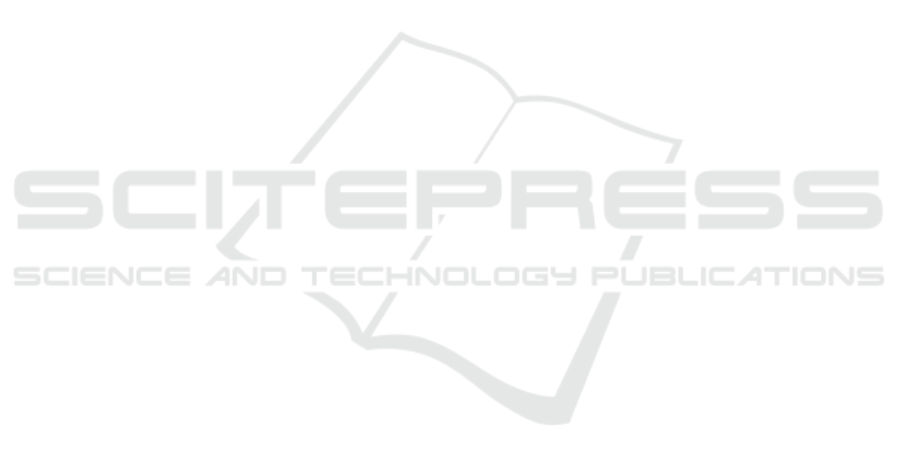
for their subproblems.
The obtained results offered important insights
and provided the evidence for the validity of multia-
gent system approach. Single run of multiagent opti-
mization results in optimal control actions for all con-
trollable devices in the community. These are in the
form of consumed and/or injected energy as well as
temperature set points for thermal loads. The system
demonstrated the ability to offer solutions satisfying
all the constraints whenever feasible solutions exist.
These constraints involve allowed state of charge of
battery, temperature limits in each time unit and en-
ergy balance in each node of the network. The sug-
gested consumption profiles are meaningful. The en-
ergy consumption for flexible loads is scheduled in
times when renewable energy is available. The ex-
port of energy is scheduled so that local demand is
met first and only excess of local supply is fed into
the main grid.
As opposed to many existing studies in the liter-
ature that consider artificial case studies where the
number of prosumers and consumers are carefully se-
lected, the presented study involved a real-world com-
munity of households. These households have simi-
lar characteristics and are located in a close neighbor-
hood. As a result, rooftop solar panels produce renew-
able energy in same times during the day and in simi-
lar amounts. The consumption patterns of households
are also similar, which is due to social and economic
similarities of neighbors. Often, this leads to the situ-
ation when solar panels intensively produce electric-
ity virtually all households have the energy in excess.
In such times, the community has a surplus of cheap
energy and no local demand to use it. As a result, an
energy sharing mechanism is not fully appreciated by
community members. One possible way to address it
can be through considering more flexible loads. An-
other can be through encouraging households without
PV panels to join the community. Such new commu-
nity members would benefit from consuming the sur-
plus of local renewable energy. Importantly, house-
holds that are the energy producers will also be better
off due to differences in prices for export to the main
grid and for selling to community peers.
As future work, we will address the issue of a fair
distribution of costs and profits between community
members.
ACKNOWLEDGEMENTS
This research has received funding from the European
Union’s Horizon 2020 research and innovation pro-
gramme under grant agreement No 774431 (DRIvE).
REFERENCES
Boyd, S., Parikh, N., Chu, E., Peleato, B., and Eckstein, J.
(2011). Distributed optimization and statistical learn-
ing via the alternating direction method of multipliers.
Found. Trends Mach. Learn., 3(1):1–122.
Kantamneni, A., Brown, L. E., Parker, G., and Weaver,
W. W. (2015). Survey of multi-agent systems for mi-
crogrid control. Engineering Applications of Artificial
Intelligence, 45:192–203.
Kraning, M., Chu, E., Lavaei, J., and Boyd, S. (2014). Dy-
namic network energy management via proximal mes-
sage passing. Found. Trends Optim., 1(2):73–126.
Lilliu, F., Vinyals, M., Denysiuk, R., and Recupero, D. R.
(2019). A novel payment scheme for trading renew-
able energy in smart grid. In Proceedings of the Tenth
ACM International Conference on Future Energy Sys-
tems, e-Energy ’19, pages 111–115.
Long, C., Wu, J., Zhou, Y., and Jenkins, N. (2018). Peer-to-
peer energy sharing through a two-stage aggregated
battery control in a community microgrid. Applied
Energy, 226:261–276.
Mihaylov, M., Jurado, S., Avellana, N., Van Moffaert, K.,
de Abril, I. M., and Now
´
e, A. (2014). Nrgcoin: Vir-
tual currency for trading of renewable energy in smart
grids. In 11th International Conference on the Euro-
pean Energy Market, pages 1–6.
Paola, A. D., Angeli, D., and Strbac, G. (2017). Price-
based schemes for distributed coordination of flexible
demand in the electricity market. IEEE Transactions
on Smart Grid, 8:3104–3116.
Shamsi, P., Xie, H., Longe, A., and Joo, J. (2016). Eco-
nomic dispatch for an agent-based community micro-
grid. IEEE Trans Smart Grid, 7(5):2317–24.
Sousa, T., Soares, T., Pinson, P., Moret, F., Baroche, T., and
Sorin, E. (2019). Peer-to-peer and community-based
markets: A comprehensive review. Renewable and
Sustainable Energy Reviews, 104:367 – 378.
Tasdighi, M., Ghasemi, H., and Rahimi-Kian, A. (2014).
Residential microgrid scheduling based on smart me-
ters data and temperature dependent thermal load
modeling. IEEE Transactions on Smart Grid, 5:349–
357.
Vinyals, M., Velay, M., and Sisinni, M. (2018). A multi-
agent system for energy trading between prosumers.
In 14th International Conference on Distributed Com-
puting and Artificial Intelligence, pages 79–86.
Yang, P. and Nehorai, A. (2014). Joint optimization of hy-
brid energy storage and generation capacity with re-
newable energy. IEEE Transactions on Smart Grid,
5(4):1566–1574.
Multiagent System for Community Energy Management
39