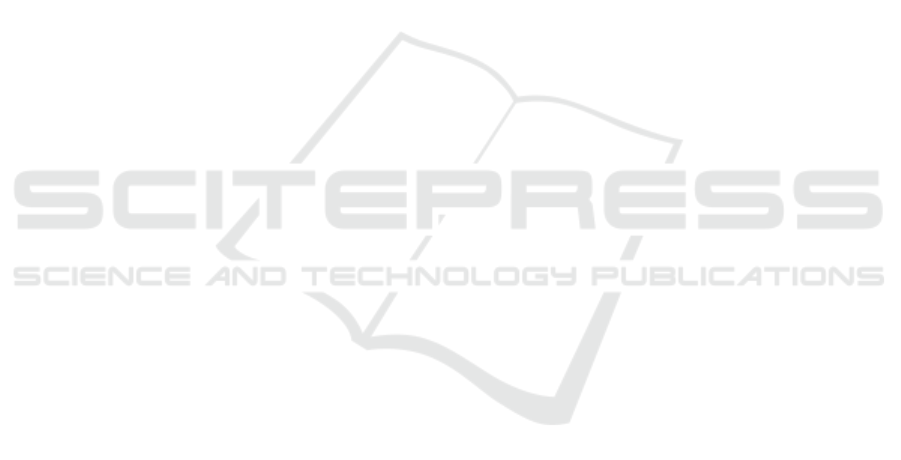
label (with binary values 1 and 2) is used for iden-
tifying which camera that an image is coming from,
we found it hard to extract the exact camera IDs from
CUHK03. Thus we only test our model without en-
abling the view-specific learning on this dataset. In
table 3, we show the results of our proposed method
on CUHK03. Remarkably, although the MRFA mod-
ule is not guided by camera ID, our model still out-
performs all other methods by a large margin.
5 CONCLUSION
In this work, we introduce a novel multi-receptive
field attention module which brings a considerable
performance boost to a strip-based person re-ID net-
work. Besides, we propose a horizontal data augmen-
tation strategy which is shown to be particularly help-
ful against misalignment issues. Combined with the
idea of injecting view information through the atten-
tion module, our proposed model achieves superior
performance comparing to current state-of-the-art on
three widely used person re-identification benchmark
datasets.
REFERENCES
Cai, H., Wang, Z., and Cheng, J. (2019). Multi-
scale body-part mask guided attention for person re-
identification. In 2019 The IEEE Conference on
Computer Vision and Pattern Recognition Workshop
(CVPRW).
Chang, X., Hospedales, T. M., and Xiang, T. (2018). Multi-
level factorisation net for person re-identification.
2018 IEEE/CVF Conference on Computer Vision and
Pattern Recognition.
Chen, Y., Zhu, X., and Gong, S. (2017). Person re-
identification by deep learning multi-scale represen-
tations. In 2017 IEEE International Conference on
Computer Vision Workshops (ICCVW), pages 2590–
2600.
Fu, Y., Wei, Y., Zhou, Y., Shi, H., Huang, G., Wang, X.,
Yao, Z., and Huang, T. (2018). Horizontal pyramid
matching for person re-identification. arXiv preprint
arXiv:1804.05275.
Gray, D., Brennan, S., and Tao, H. (2007). Evaluating ap-
pearance models for recognition, reacquisition, and
tracking.
He, K., Zhang, X., Ren, S., and Sun, J. (2015). Deep resid-
ual learning for image recognition. arXiv preprint
arXiv:1512.03385.
Hermans, A., Beyer, L., and Leibe, B. (2017). In defense
of the triplet loss for person re-identification. arXiv
preprint arXiv:1703.07737.
Li, W., Zhao, R., Xiao, T., and Wang, X. (2014). Deep-
reid: Deep filter pairing neural network for person re-
identification. In The IEEE Conference on Computer
Vision and Pattern Recognition (CVPR).
Li, W., Zhu, X., and Gong, S. (2018). Harmonious attention
network for person re-identification. In Proceedings of
the IEEE Conference on Computer Vision and Pattern
Recognition, pages 2285–2294.
Ristani, E., Solera, F., Zou, R., Cucchiara, R., and Tomasi,
C. (2016). Performance measures and a data set
for multi-target, multi-camera tracking. In European
Conference on Computer Vision workshop on Bench-
marking Multi-Target Tracking.
Sun, Y., Zheng, L., Deng, W., and Wang, S. (2017). Svd-
net for pedestrian retrieval. 2017 IEEE International
Conference on Computer Vision (ICCV).
Sun, Y., Zheng, L., Yang, Y., Tian, Q., and Wang, S. (2018).
Beyond part models: Person retrieval with refined part
pooling (and a strong convolutional baseline). In Pro-
ceedings of the European Conference on Computer Vi-
sion (ECCV), pages 480–496.
Szegedy, C., Vanhoucke, V., Ioffe, S., Shlens, J., and Wojna,
Z. (2016). Rethinking the inception architecture for
computer vision. In The IEEE Conference on Com-
puter Vision and Pattern Recognition (CVPR).
Wang, C., Zhang, Q., Huang, C., Liu, W., and Wang, X.
(2018a). Mancs: A multi-task attentional network
with curriculum sampling for person re-identification.
In The European Conference on Computer Vision
(ECCV).
Wang, G., Yuan, Y., Chen, X., Li, J., and Zhou, X. (2018b).
Learning discriminative features with multiple granu-
larities for person re-identification. In Proceedings of
the 26th ACM International Conference on Multime-
dia, MM ’18, pages 274–282, New York, NY, USA.
ACM.
Yang, F., Yan, K., Lu, S., Jia, H., Xie, X., and Gao, W.
(2019). Attention driven person re-identification. Pat-
tern Recognition, 86:143 – 155.
Yu, H., Wu, A., and Zheng, W. (2018). Unsupervised per-
son re-identification by deep asymmetric metric em-
bedding. IEEE Transactions on Pattern Analysis and
Machine Intelligence, pages 1–1.
Zhang, X., Luo, H., Fan, X., Xiang, W., Sun, Y., Xiao,
Q., Jiang, W., Zhang, C., and Sun, J. (2017). Aligne-
dreid: Surpassing human-level performance in person
re-identification. arXiv preprint arXiv:1711.08184.
Zheng, L., Shen, L., Tian, L., Wang, S., Wang, J., and Tian,
Q. (2015). Scalable person re-identification: A bench-
mark. In The IEEE International Conference on Com-
puter Vision (ICCV).
Zheng, Z., Zheng, L., and Yang, Y. (2018). Pedestrian align-
ment network for large-scale person re-identification.
IEEE Transactions on Circuits and Systems for Video
Technology, page 1–1.
Zhong, Z., Zheng, L., Cao, D., and Li, S. (2017a). Re-
ranking person re-identification with k-reciprocal en-
coding. 2017 IEEE Conference on Computer Vision
and Pattern Recognition (CVPR).
Zhong, Z., Zheng, L., Kang, G., Li, S., and Yang, Y.
(2017b). Random erasing data augmentation. arXiv
preprint arXiv:1708.04896.
ICAART 2020 - 12th International Conference on Agents and Artificial Intelligence
420