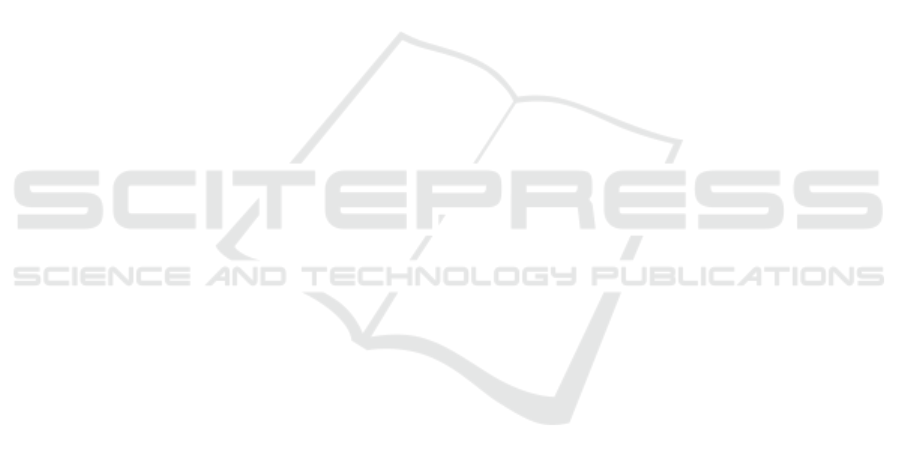
REFERENCES
Abdullah, M. A., Ren, H., Ammar, H. B., Milenkovic, V.,
Luo, R., Zhang, M., and Wang, J. (2019). Wasser-
stein robust reinforcement learning. arXiv preprint
arXiv:1907.13196.
Aksaray, D., Jones, A., Kong, Z., Schwager, M., and Belta,
C. (2016). Q-learning for robust satisfaction of signal
temporal logic specifications. In 2016 IEEE 55th Con-
ference on Decision and Control (CDC), pages 6565–
6570. IEEE.
Al-Ansari, M. A. and Williams, R. J. (1999). Robust, effi-
cient, globally-optimized reinforcement learning with
the parti-game algorithm. In Advances in Neural In-
formation Processing Systems, pages 961–967.
Anderson, C. W., Young, P. M., Buehner, M. R., Knight,
J. N., Bush, K. A., and Hittle, D. C. (2007). Robust re-
inforcement learning control using integral quadratic
constraints for recurrent neural networks. IEEE Trans-
actions on Neural Networks, 18(4):993–1002.
Gu, Z., Jia, Z., and Choset, H. (2018). Adversary a3c for
robust reinforcement learning.
Jones, A., Aksaray, D., Kong, Z., Schwager, M., and Belta,
C. (2015). Robust satisfaction of temporal logic spec-
ifications via reinforcement learning. arXiv preprint
arXiv:1510.06460.
Killian, T. W., Daulton, S., Konidaris, G., and Doshi-
Velez, F. (2017). Robust and efficient transfer learning
with hidden parameter markov decision processes. In
Advances in Neural Information Processing Systems,
pages 6250–6261.
Kinjo, K., Uchibe, E., and Doya, K. (2018). Robustness
of linearly solvable markov games employing inac-
curate dynamics model. Artificial Life and Robotics,
23(1):1–9.
Kretchmar, R. M., Young, P. M., Anderson, C. W., Hit-
tle, D. C., Anderson, M. L., and Delnero, C. C.
(2001). Robust reinforcement learning control with
static and dynamic stability. International Journal of
Robust and Nonlinear Control: IFAC-Affiliated Jour-
nal, 11(15):1469–1500.
Lim, S. H., Xu, H., and Mannor, S. (2013). Reinforce-
ment learning in robust markov decision processes. In
Advances in Neural Information Processing Systems,
pages 701–709.
Loughlin, D. H., Ranjithan, S. R., Brill Jr, E. D., and
Baugh Jr, J. W. (2001). Genetic algorithm approaches
for addressing unmodeled objectives in optimization
problems. Engineering Optimization, 33(5):549–569.
Mania, H., Guy, A., and Recht, B. (2018). Simple random
search provides a competitive approach to reinforce-
ment learning. arXiv preprint arXiv:1803.07055.
Mankowitz, D. J., Mann, T. A., Bacon, P.-L., Precup, D.,
and Mannor, S. (2018). Learning robust options. In
Thirty-Second AAAI Conference on Artificial Intelli-
gence.
Morimoto, J. and Doya, K. (2005). Robust reinforcement
learning. Neural computation, 17(2):335–359.
Oguni., K., Narisawa., K., and Shinohara., A. (2014).
Reducing sample complexity in reinforcement learn-
ing by transferring transition and reward probabili-
ties. In Proceedings of the 6th International Confer-
ence on Agents and Artificial Intelligence - Volume 1:
ICAART,, pages 632–638. INSTICC, SciTePress.
Pattanaik, A., Tang, Z., Liu, S., Bommannan, G., and
Chowdhary, G. (2018). Robust deep reinforcement
learning with adversarial attacks. In Proceedings
of the 17th International Conference on Autonomous
Agents and MultiAgent Systems, pages 2040–2042. In-
ternational Foundation for Autonomous Agents and
Multiagent Systems.
Rajeswaran, A., Ghotra, S., Ravindran, B., and Levine,
S. (2016). Epopt: Learning robust neural network
policies using model ensembles. arXiv preprint
arXiv:1610.01283.
Sami, A. and Memon, A. Y. (2018). Robust optimal control
of continuous time linear system using reinforcement
learning. In 2018 Australian & New Zealand Control
Conference (ANZCC), pages 154–159. IEEE.
Singh, S. P., Barto, A. G., Grupen, R., and Connolly, C.
(1994). Robust reinforcement learning in motion plan-
ning. In Advances in neural information processing
systems, pages 655–662.
Teh, Y., Bapst, V., Czarnecki, W. M., Quan, J., Kirkpatrick,
J., Hadsell, R., Heess, N., and Pascanu, R. (2017).
Distral: Robust multitask reinforcement learning. In
Advances in Neural Information Processing Systems,
pages 4496–4506.
Tessler, C., Efroni, Y., and Mannor, S. (2019). Action robust
reinforcement learning and applications in continuous
control. arXiv preprint arXiv:1901.09184.
Zhan, Z.-H., Li, J., Cao, J., Zhang, J., Chung, H. S.-H.,
and Shi, Y.-H. (2013). Multiple populations for multi-
ple objectives: A coevolutionary technique for solving
multiobjective optimization problems. IEEE transac-
tions on cybernetics, 43(2):445–463.
ICAART 2020 - 12th International Conference on Agents and Artificial Intelligence
428