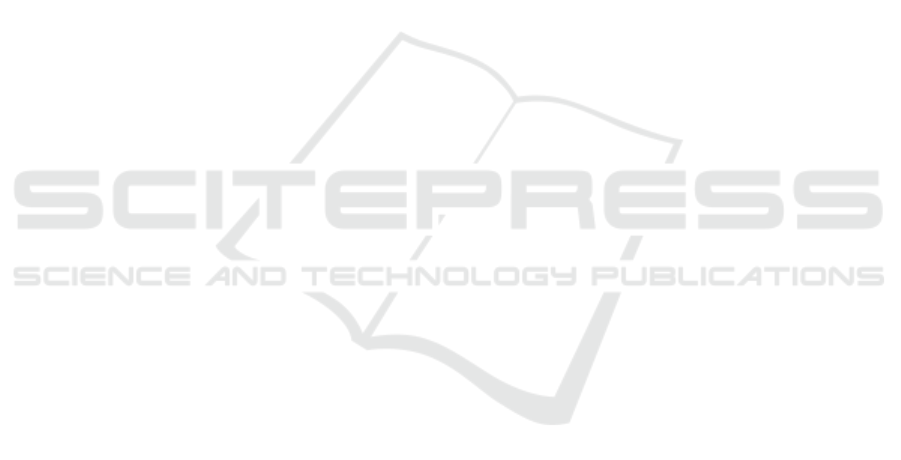
Deecke, L., Vandermeulen, R., Ruff, L., Mandt, S., and
Kloft, M. (2018). Image anomaly detection with gen-
erative adversarial networks. In Joint European Con-
ference on Machine Learning and Knowledge Discov-
ery in Databases, pages 3–17. Springer.
Deng, J., Dong, W., Socher, R., Li, L.-J., Li, K., and Fei-Fei,
L. (2009). Imagenet: A large-scale hierarchical image
database. In Proc. 2009 IEEE conference on computer
vision and pattern recognition, pages 248–255. Ieee.
Fraley, C. and Raftery, A. E. (2002). Model-based clus-
tering, discriminant analysis, and density estima-
tion. Journal of the American statistical Association,
97(458):611–631.
G
¨
ornitz, N., Kloft, M., Rieck, K., and Brefeld, U. (2013).
Toward supervised anomaly detection. Journal of Ar-
tificial Intelligence Research, 46:235–262.
Hasegawa, T., Ogata, J., Murakawa, M., and Ogawa, T.
(2018). Tandem connectionist anomaly detection:
Use of faulty vibration signals in feature represen-
tation learning. In Proc. 2018 IEEE International
Conference on Prognostics and Health Management
(ICPHM), pages 1–7. IEEE.
He, K., Zhang, X., Ren, S., and Sun, J. (2016). Deep resid-
ual learning for image recognition. In Proceedings of
the IEEE conference on computer vision and pattern
recognition, pages 770–778.
Kingma, D. P. and Welling, M. (2013). Auto-encoding vari-
ational bayes. arXiv preprint arXiv:1312.6114.
Krizhevsky, A., Hinton, G., et al. (2009). Learning multiple
layers of features from tiny images. Technical report,
Citeseer.
Laskov, P., D
¨
ussel, P., Sch
¨
afer, C., and Rieck, K. (2005).
Learning intrusion detection: supervised or unsuper-
vised? In Proc. International Conference on Image
Analysis and Processing, pages 50–57. Springer.
LeCun, Y., Cortes, C., and Burges, C. J. (1998). The
mnist database of handwritten digits, 1998. URL
http://yann. lecun. com/exdb/mnist, 10:34.
Liu, F. T., Ting, K. M., and Zhou, Z.-H. (2008). Isolation
forest. In 2008 Eighth IEEE International Conference
on Data Mining, pages 413–422. IEEE.
Masci, J., Meier, U., Cires¸an, D., and Schmidhuber, J.
(2011). Stacked convolutional auto-encoders for hi-
erarchical feature extraction. In International Con-
ference on Artificial Neural Networks, pages 52–59.
Springer.
Moyne, J. and Iskandar, J. (2017). Big data analytics for
smart manufacturing: Case studies in semiconductor
manufacturing. Processes, 5(3):39.
Oja, E. (1983). Subspace methods of pattern recognition,
volume 6. Research Studies Press.
Parzen, E. (1962). On estimation of a probability density
function and mode. The annals of mathematical statis-
tics, 33(3):1065–1076.
Schlegl, T., Seeb
¨
ock, P., Waldstein, S. M., Schmidt-Erfurth,
U., and Langs, G. (2017). Unsupervised anomaly de-
tection with generative adversarial networks to guide
marker discovery. In International Conference on In-
formation Processing in Medical Imaging, pages 146–
157. Springer.
Sch
¨
olkopf, B., Platt, J. C., Shawe-Taylor, J., Smola, A. J.,
and Williamson, R. C. (2001). Estimating the support
of a high-dimensional distribution. Neural computa-
tion, 13(7):1443–1471.
Scholkopf, B. and Smola, A. J. (2001). Learning with ker-
nels: support vector machines, regularization, opti-
mization, and beyond. MIT press.
Tax, D. M. and Duin, R. P. (2004). Support vector data
description. Machine learning, 54(1):45–66.
Wang, Y., Wong, J., and Miner, A. (2004). Anomaly in-
trusion detection using one class svm. In Proceedings
from the Fifth Annual IEEE SMC Information Assur-
ance Workshop, 2004., pages 358–364. IEEE.
Watanabe, S. and Pakvasa, N. (1973). Subspace method of
pattern recognition. In Proc. 1st. IJCPR, pages 25–32.
Ye, J., Iwata, M., Takumi, K., Murakawa, M., Tetsuya, H.,
Kubota, Y., Yui, T., and Mori, K. (2014). Statistical
impact-echo analysis based on grassmann manifold
learning: Its preliminary results for concrete condition
assessment. In Proc. EWSHM - 7th European Work-
shop on Structural Health Monitoring.
Zhou, C. and Paffenroth, R. C. (2017). Anomaly detec-
tion with robust deep autoencoders. In Proceedings of
the 23rd ACM SIGKDD International Conference on
Knowledge Discovery and Data Mining, pages 665–
674. ACM.
ICPRAM 2020 - 9th International Conference on Pattern Recognition Applications and Methods
158