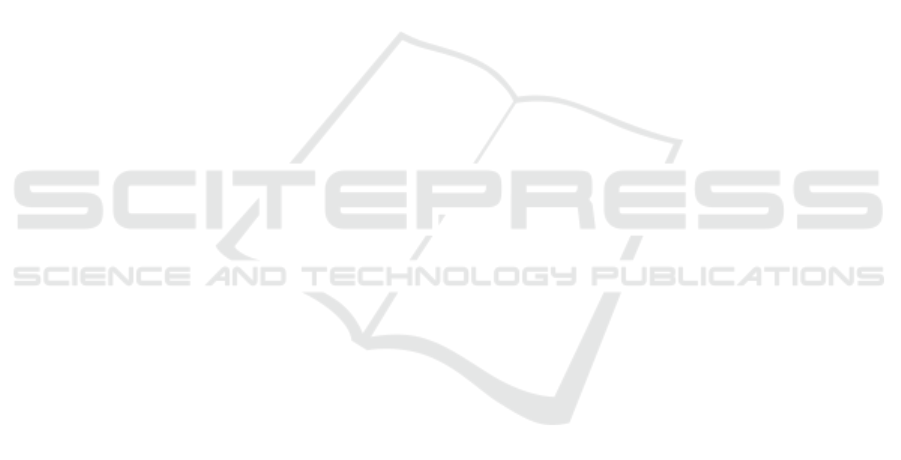
Engelbrecht, A. (2012). Particle swarm optimization: Ve-
locity initialization. In Evolutionary Computation
(CEC), 2012 IEEE Congress on, pages 1–8. IEEE.
Han, F., Sun, W., and Ling, Q.-H. (2014). A novel
strategy for gene selection of microarray data based
on gene-to-class sensitivity information. PloS one,
9(5):e97530.
Han, F., Yang, C., Wu, Y.-Q., Zhu, J.-S., Ling, Q.-H.,
Song, Y.-Q., and Huang, D.-S. (2017). A gene se-
lection method for microarray data based on binary
pso encoding gene-to-class sensitivity information.
IEEE/ACM Transactions on Computational Biology
and Bioinformatics (TCBB), 14(1):85–96.
John, G. H., Kohavi, R., and Pfleger, K. (1994). Irrelevant
features and the subset selection problem. In Machine
Learning Proceedings 1994, pages 121–129. Elsevier.
Kennedy, J. and Eberhart, R. C. (1997). A discrete bi-
nary version of the particle swarm algorithm. In 1997
IEEE International Conference on Systems, Man, and
Cybernetics. Computational Cybernetics and Simula-
tion, volume 5, pages 4104–4108 vol.5.
Khaire, U. M. and Dhanalakshmi, R. (2019). Stability of
feature selection algorithm: A review. Journal of King
Saud University-Computer and Information Sciences.
Kumar, V. and Minz, S. (2014). Feature selection. SmartCR,
4(3):211–229.
Lee, S., Soak, S., Oh, S., Pedrycz, W., and Jeon, M.
(2008). Modified binary particle swarm optimization.
Progress in Natural Science, 18(9):1161–1166.
Lopez-Paz, D., Hennig, P., and Sch
¨
olkopf, B. (2013). The
randomized dependence coefficient. In Advances in
neural information processing systems, pages 1–9.
Mohamad, M. S., Omatu, S., Deris, S., and Yoshioka, M.
(2011). A modified binary particle swarm optimiza-
tion for selecting the small subset of informative genes
from gene expression data. IEEE Transactions on In-
formation Technology in Biomedicine, 15(6):813–822.
Mohammadi, M., Noghabi, H. S., Hodtani, G. A., and
Mashhadi, H. R. (2016). Robust and stable gene se-
lection via maximum–minimum correntropy criterion.
Genomics, 107(2-3):83–87.
Perthame, E., Friguet, C., and Causeur, D. (2016). Stability
of feature selection in classification issues for high-
dimensional correlated data. Statistics and Comput-
ing, 26(4):783–796.
Poli, R. (2009). Mean and variance of the sampling dis-
tribution of particle swarm optimizers during stagna-
tion. IEEE Transactions on Evolutionary Computa-
tion, 13(4):712–721.
Rezaee Jordehi, A. and Jasni, J. (2013). Parameter selec-
tion in particle swarm optimisation: a survey. Journal
of Experimental & Theoretical Artificial Intelligence,
25(4):527–542.
Shipp, M. A., Ross, K. N., Tamayo, P., Weng, A. P., Ku-
tok, J. L., Aguiar, R. C. T., Gaasenbeek, M., An-
gelo, M., Reich, M., Pinkus, G. S., Ray, T. S., Koval,
M. A., Last, K. W., Norton, A., Lister, T. A., Mesirov,
J., Neuberg, D. S., Lander, E. S., Aster, J. C., and
Golub, T. R. (2002). Diffuse large b-cell lymphoma
outcome prediction by gene-expression profiling and
supervised machine learning. Nature Medicine, 8:68
EP –. Article.
Singh, D., Febbo, P. G., Ross, K., Jackson, D. G., Manola,
J., Ladd, C., Tamayo, P., Renshaw, A. A., D’Amico,
A. V., Richie, J. P., Lander, E. S., Loda, M., Kantoff,
P. W., Golub, T. R., and Sellers, W. R. (2002). Gene
expression correlates of clinical prostate cancer be-
havior. Cancer Cell, 1(2):203 – 209.
Van den Bergh, F. and Engelbrecht, A. P. (2010). A conver-
gence proof for the particle swarm optimiser. Funda-
menta Informaticae, 105(4):341–374.
Xue, B., Zhang, M., and Browne, W. N. (2013). Particle
swarm optimization for feature selection in classifica-
tion: A multi-objective approach. IEEE transactions
on cybernetics, 43(6):1656–1671.
Ye, F. (2017). Particle swarm optimization-based automatic
parameter selection for deep neural networks and its
applications in large-scale and high-dimensional data.
PloS one, 12(12):e0188746.
Yong, Z., Dun-wei, G., and Wan-qiu, Z. (2016). Feature
selection of unreliable data using an improved multi-
objective pso algorithm. Neurocomputing, 171:1281–
1290.
Zhang, Y., Gong, D.-w., and Cheng, J. (2017). Multi-
objective particle swarm optimization approach
for cost-based feature selection in classification.
IEEE/ACM Transactions on Computational Biology
and Bioinformatics (TCBB), 14(1):64–75.
ICAART 2020 - 12th International Conference on Agents and Artificial Intelligence
444