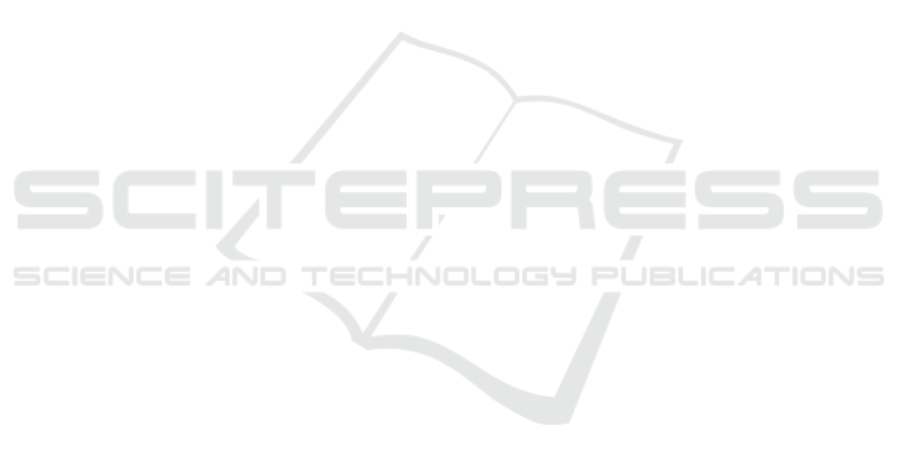
peated games. In Proceedings of the 8th International
Conference on Agents and Artificial Intelligence - Vol-
ume 1: ICAART,, pages 47–55.
Govindan, S. and Wilson, R. (2003). A global newton
method to compute nash equilibria. Journal of Eco-
nomic Theory, 110(1):65–86.
Harsanyi, J. C. and Selten, R. (1972). A generalized nash
solution for two-person bargaining games with incom-
plete information. Management Science, 18(5-part-
2):80–106.
Hennig, P. (2013). Fast probabilistic optimization from
noisy gradients. In ICML (1), pages 62–70.
Jiang, A. X. and Leyton-Brown, K. (2010). Bayesian
action-graph games. In Advances in Neural Informa-
tion Processing Systems, pages 991–999.
Kho, J., Rogers, A., and Jennings, N. R. (2009). Decen-
tralized control of adaptive sampling in wireless sen-
sor networks. ACM Transactions on Sensor Networks
(TOSN), 5(3):19.
Kitano, H., Tadokoro, S., Noda, I., Matsubara, H., Taka-
hashi, T., Shinjou, A., and Shimada, S. (1999).
Robocup rescue: Search and rescue in large-scale dis-
asters as a domain for autonomous agents research. In
Systems, Man, and Cybernetics, 1999. IEEE SMC’99
Conference Proceedings. 1999 IEEE International
Conference on, volume 6, pages 739–743. IEEE.
Koller, D. and Milch, B. (2003). Multi-agent influence di-
agrams for representing and solving games. Games
and Economic Behavior, 45(1):181–221.
Kostelnik, P., Hudec, M., and
ˇ
Samulka, M. (2002). Dis-
tributed learning in behaviour based mobile robot con-
trol. Intelligent Technologies-Theory and Applica-
tions.
Leslie, D. S. and Collins, E. J. (2006). Generalised weak-
ened fictitious play. Games and Economic Behavior,
56(2):285–298.
Madhavan, R., Fregene, K., and Parker, L. (2004). Dis-
tributed cooperative outdoor multirobot localization
and mapping. Autonomous Robots, 17(1):23–39.
Miyasawa, K. (1961). On the convergence of learning pro-
cess in a 2x2 non-zero-person game.
Monderer, D. and Shapley, L. (1996). Potential games.
Games and Economic Behavior, 14:124–143.
Nachbar, J. (1990). Evolutionary’ selection dynamics in
games: Convergence and limit properties. Interna-
tional Journal of Game Theory, 19:59–89.
Nash, J. (1950). Equilibrium points in n-person games.
In Proceedings of the National Academy of Science,
USA, volume 36, pages 48–49.
Oliehoek, F. A., Spaan, M. T., Dibangoye, J. S., and Am-
ato, C. (2010). Heuristic search for identical payoff
bayesian games. In Proceedings of the 9th Interna-
tional Conference on Autonomous Agents and Multia-
gent Systems: volume 1-Volume 1, pages 1115–1122.
International Foundation for Autonomous Agents and
Multiagent Systems.
Paruchuri, P., Pearce, J. P., Tambe, M., Ordonez, F., and
Kraus, S. (2007a). An efficient heuristic for security
against multiple adversaries in stackelberg games. In
AAAI Spring Symposium: Game Theoretic and Deci-
sion Theoretic Agents, pages 38–46.
Paruchuri, P., Pearce, J. P., Tambe, M., Ordonez, F., and
Kraus, S. (2007b). An efficient heuristic for security
against multiple adversaries in stackelberg games. In
AAAI Spring Symposium: Game Theoretic and Deci-
sion Theoretic Agents, pages 38–46.
Raffard, R. L., Tomlin, C. J., and Boyd, S. P. (2004). Dis-
tributed optimization for cooperative agents: Appli-
cation to formation flight. In Decision and Control,
2004. CDC. 43rd IEEE Conference on, volume 3,
pages 2453–2459. IEEE.
Robinson, J. (1951). An iterative method of solving a game.
Annals of Mathematics, 54:296–301.
Rosen, D. M. and Leonard, J. J. Nonparametric density
estimation for learning noise distributions in mobile
robotics.
Ruan, S., Meirina, C., Yu, F., Pattipati, K. R., and Popp,
R. L. (2005). Patrolling in a stochastic environment. In
10 International Symposium on Command and Con-
trol.
Sakka, S. (2013). Bayesian Filtering and Smoothing. Cam-
bridge University Press.
Sandholm, T., Gilpin, A., and Conitzer, V. (2005). Mixed-
integer programming methods for finding nash equi-
libria. In Proceedings of the National Conference on
Artificial Intelligence, volume 20.
Semsar-Kazerooni, E. and Khorasani, K. (2009). Multi-
agent team cooperation: A game theory approach. Au-
tomatica, 45(10):2205 – 2213.
Smyrnakis, M. and Galla, T. (2015). Decentralized optimi-
sation of resource allocation in disaster management.
In City Evacuations: An Interdisciplinary Approach,
pages 89–106. Springer.
Smyrnakis, M., Kladis, G. P., Aitken, J. M., and Veres,
S. M. (2016). Distributed selection of flight forma-
tion in uav missions. Journal of Applied Mathematics
and Bioinformatics, 6(3):93–124.
Smyrnakis, M. and Leslie, D. S. (2010). Dynamic Opponent
Modelling in Fictitious Play. The Computer Journal,
53:1344–1359.
Smyrnakis, M. and Veres, S. M. (2016). Fictitious play for
cooperative action selection in robot teams. Eng. Appl.
of AI, 56:14–29.
Tsitsiklis, J. N. and Athans, M. (1985). On the complex-
ity of decentralized decision making and detection
problems. Automatic Control, IEEE Transactions on,
30(5):440–446.
Verstaevel, N., Boes, J., Nigon, J., d’Amico, D., and
Gleizes, M.-P. (2017). Lifelong machine learning with
adaptive multi-agent systems. In Proceedings of the
9th International Conference on Agents and Artificial
Intelligence - Volume 2: ICAART,, pages 275–286.
Zhang, Y., Schervish, M., Acar, E., and Choset, H. (2001).
Probabilistic methods for robotic landmine search. In
Proceedings of the 2001 IEEE/RSJ International Con-
ference on Intelligent Robots and Systems (IROS ’01),
pages 1525 – 1532.
Multi-model Adaptive Learning for Robots Under Uncertainty
61