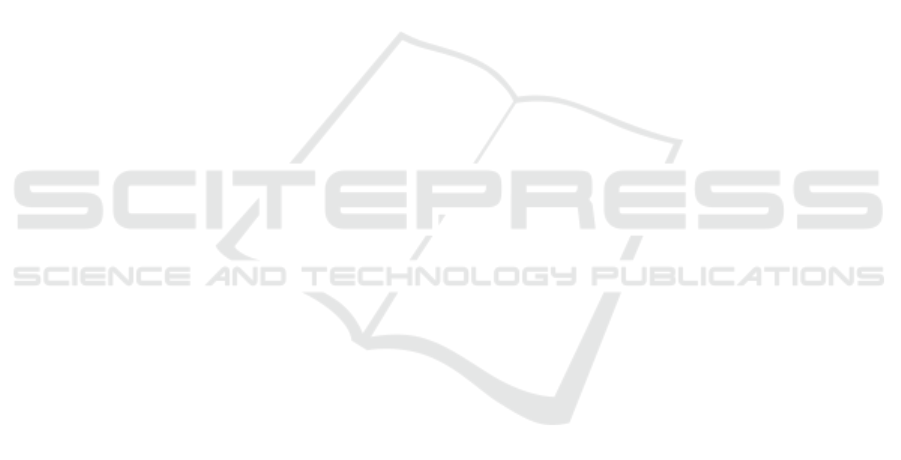
mechanism. In Proceedings of the IEEE International
Conference on Computer Vision, pages 4836–4845.
Cordts, M., Omran, M., Ramos, S., Rehfeld, T., Enzweiler,
M., Benenson, R., Franke, U., Roth, S., and Schiele,
B. (2016). The cityscapes dataset for semantic urban
scene understanding. In Proceedings of the IEEE con-
ference on computer vision and pattern recognition,
pages 3213–3223.
Dredze, M. and Crammer, K. (2008). Online methods for
multi-domain learning and adaptation. In Proceed-
ings of the Conference on Empirical Methods in Nat-
ural Language Processing, pages 689–697. Associa-
tion for Computational Linguistics.
Gordon, D., Farhadi, A., and Fox, D. (2018). Re3: Re al-
time recurrent regression networks for visual tracking
of generic objects. IEEE Robotics and Automation
Letters, 3(2):788–795.
Grabner, H., Grabner, M., and Bischof, H. (2006). Real-
time tracking via on-line boosting. In Bmvc, volume 1,
page 6.
Grabner, H., Leistner, C., and Bischof, H. (2008). Semi-
supervised on-line boosting for robust tracking. In
European conference on computer vision, pages 234–
247. Springer.
Hare, S., Golodetz, S., Saffari, A., Vineet, V., Cheng, M.-
M., Hicks, S. L., and Torr, P. H. (2015). Struck:
Structured output tracking with kernels. IEEE trans-
actions on pattern analysis and machine intelligence,
38(10):2096–2109.
Held, D., Thrun, S., and Savarese, S. (2016). Learning to
track at 100 fps with deep regression networks. In Eu-
ropean Conference on Computer Vision, pages 749–
765. Springer.
Jepson, A. D., Fleet, D. J., and El-Maraghi, T. F. (2003).
Robust online appearance models for visual tracking.
IEEE transactions on pattern analysis and machine
intelligence, 25(10):1296–1311.
Jiang, H., Fels, S., and Little, J. J. (2007). A linear pro-
gramming approach for multiple object tracking. In
2007 IEEE Conference on Computer Vision and Pat-
tern Recognition, pages 1–8. IEEE.
Kalal, Z., Matas, J., and Mikolajczyk, K. (2010). Pn
learning: Bootstrapping binary classifiers by struc-
tural constraints. In 2010 IEEE Computer Society
Conference on Computer Vision and Pattern Recog-
nition, pages 49–56. IEEE.
Li, B., Yan, J., Wu, W., Zhu, Z., and Hu, X. (2018). High
performance visual tracking with siamese region pro-
posal network. In Proceedings of the IEEE Confer-
ence on Computer Vision and Pattern Recognition,
pages 8971–8980.
Maninis, K.-K., Caelles, S., Pont-Tuset, J., and Van Gool,
L. (2018). Deep extreme cut: From extreme points to
object segmentation. In Proceedings of the IEEE Con-
ference on Computer Vision and Pattern Recognition,
pages 616–625.
Milan, A., Leal-Taixé, L., Reid, I., Roth, S., and Schindler,
K. (2016). Mot16: A benchmark for multi-object
tracking. arXiv preprint arXiv:1603.00831.
Milan, A., Roth, S., and Schindler, K. (2013). Continuous
energy minimization for multitarget tracking. IEEE
transactions on pattern analysis and machine intelli-
gence, 36(1):58–72.
Nam, H. and Han, B. (2016). Learning multi-domain con-
volutional neural networks for visual tracking. In Pro-
ceedings of the IEEE Conference on Computer Vision
and Pattern Recognition, pages 4293–4302.
Pinheiro, P. O., Collobert, R., and Dollár, P. (2015). Learn-
ing to segment object candidates. In Advances in
Neural Information Processing Systems, pages 1990–
1998.
Pirsiavash, H., Ramanan, D., and Fowlkes, C. C. (2011).
Globally-optimal greedy algorithms for tracking a
variable number of objects. In CVPR 2011, pages
1201–1208. IEEE.
Redmon, J. and Farhadi, A. (2018). Yolov3: An incremental
improvement. arXiv preprint arXiv:1804.02767.
Ross, D. A., Lim, J., Lin, R.-S., and Yang, M.-H. (2008).
Incremental learning for robust visual tracking. Inter-
national journal of computer vision, 77(1-3):125–141.
Tao, R., Gavves, E., and Smeulders, A. W. (2016). Siamese
instance search for tracking. In Proceedings of the
IEEE conference on computer vision and pattern
recognition, pages 1420–1429.
Varda, K. (2008). Protocol buffers: Google’s data inter-
change format. Google Open Source Blog, Available
at least as early as Jul, 72.
Wu, Y., Lim, J., and Yang, M.-H. (2013). Online object
tracking: A benchmark. In Proceedings of the IEEE
conference on computer vision and pattern recogni-
tion, pages 2411–2418.
Wu, Y., Lim, J., and Yang, M.-H. (2015). Object tracking
benchmark. IEEE Transactions on Pattern Analysis
and Machine Intelligence, 37(9):1834–1848.
Xu, N., Price, B., Cohen, S., Yang, J., and Huang, T. (2017).
Deep grabcut for object selection. arXiv preprint
arXiv:1707.00243.
Yun, S., Choi, J., Yoo, Y., Yun, K., and Young Choi, J.
(2017). Action-decision networks for visual tracking
with deep reinforcement learning. In Proceedings of
the IEEE conference on computer vision and pattern
recognition, pages 2711–2720.
Zhuang, B., Lin, G., Shen, C., and Reid, I. (2016). Fast
training of triplet-based deep binary embedding net-
works. In Proceedings of the IEEE Conference
on Computer Vision and Pattern Recognition, pages
5955–5964.
ICPRAM 2020 - 9th International Conference on Pattern Recognition Applications and Methods
332