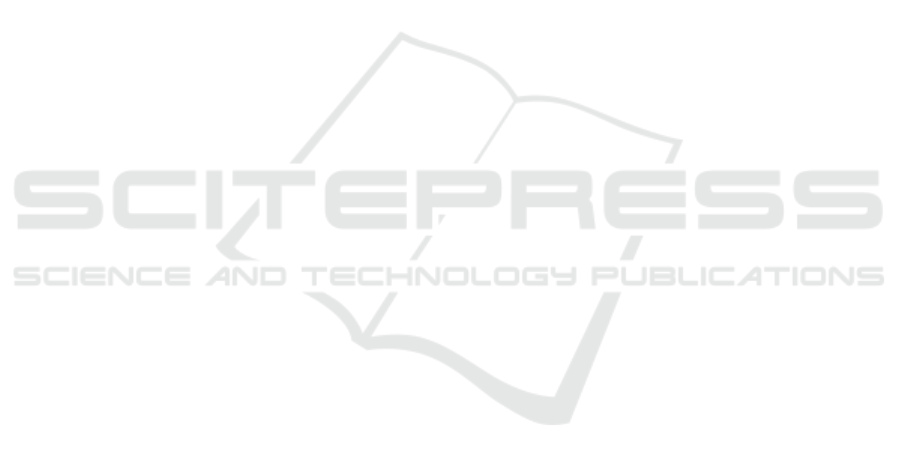
dirichlet allocation. the Journal of machine Learning
research, 3:993–1022.
Cai, D., He, X., and Han, J. (2005). Document clustering
using locality preserving indexing. IEEE Transactions
on Knowledge and Data Engineering, 17(12):1624–
1637.
Chen, C., Zhu, J., and Zhang, X. (2014). Robust bayesian
max-margin clustering. In Advances in Neural Infor-
mation Processing Systems, pages 532–540.
Csurka, G., Dance, C., Fan, L., Willamowski, J., and Bray,
C. (2004). Visual categorization with bags of key-
points. In Workshop on statistical learning in com-
puter vision, ECCV, volume 1, pages 1–2. Prague.
Fan, W., Bouguila, N., Du, J.-X., and Liu, X. (2018). Ax-
ially symmetric data clustering through dirichlet pro-
cess mixture models of watson distributions. IEEE
transactions on neural networks and learning systems,
30(6):1683–1694.
Fan, W., Sallay, H., and Bouguila, N. (2016). Online
learning of hierarchical pitman–yor process mixture
of generalized dirichlet distributions with feature se-
lection. IEEE transactions on neural networks and
learning systems, 28(9):2048–2061.
Goodfellow, I., Pouget-Abadie, J., Mirza, M., Xu, B.,
Warde-Farley, D., Ozair, S., Courville, A., and Ben-
gio, Y. (2014). Generative adversarial nets. In
Advances in neural information processing systems,
pages 2672–2680.
He, K., Zhang, X., Ren, S., and Sun, J. (2016). Deep resid-
ual learning for image recognition. In Proceedings of
the IEEE Conference on Computer Vision and Pattern
Recognition, pages 770–778.
Hoffman, M. D., Blei, D. M., Wang, C., and Paisley, J. W.
(2013). Stochastic variational inference. Journal of
Machine Learning Research, 14(1):1303–1347.
Honkela, A., Raiko, T., Kuusela, M., Tornio, M., and
Karhunen, J. (2010). Approximate riemannian con-
jugate gradient learning for fixed-form variational
bayes. Journal of Machine Learning Research,
11(Nov):3235–3268.
Honkela, A., Tornio, M., Raiko, T., and Karhunen, J.
(2007). Natural conjugate gradient in variational in-
ference. In International Conference on Neural Infor-
mation Processing, pages 305–314. Springer.
Kingma, D. P. and Welling, M. (2014). Stochastic gradi-
ent vb and the variational auto-encoder. In Second In-
ternational Conference on Learning Representations,
ICLR.
Krizhevsky, A., Sutskever, I., and Hinton, G. E. (2012). Im-
agenet classification with deep convolutional neural
networks. In Advances in neural information process-
ing systems, pages 1097–1105.
Kulis, B. and Jordan, M. I. (2012). Revisiting k-means:
New algorithms via bayesian nonparametrics. In Pro-
ceedings of the 29th International Conference on Ma-
chine Learning (ICML-12), pages 513–520.
Kurihara, K. and Welling, M. (2009). Bayesian k-means as
a maximization-expectation algorithm. Neural com-
putation, 21(4):1145–1172.
Lim, K.-L. and Wang, H. (2018). Fast approximation of
variational bayes dirichlet process mixture using the
maximization–maximization algorithm. International
Journal of Approximate Reasoning, 93:153–177.
Liu, L., Shen, C., Wang, L., van den Hengel, A., and Wang,
C. (2014). Encoding high dimensional local features
by sparse coding based fisher vectors. In Advances in
Neural Information Processing Systems, pages 1143–
1151.
Mandt, S., Hoffman, M. D., and Blei, D. M. (2017).
Stochastic gradient descent as approximate bayesian
inference. The Journal of Machine Learning Re-
search, 18(1):4873–4907.
Neal, R. M. and Hinton, G. E. (1998). A view of the em
algorithm that justifies incremental, sparse, and other
variants. In Learning in graphical models, pages 355–
368. Springer.
Nguyen, V., Phung, D., Le, T., and Bui, H. (2017). Dis-
criminative bayesian nonparametric clustering. In IJ-
CAI 2017: Proceedings of the 26th International Joint
Conference on Artificial Intelligence, pages 2550–
2556. AAAI Press.
Paisley, J., Blei, D. M., and Jordan, M. I. (2012). Variational
bayesian inference with stochastic search. In Proceed-
ings of the 29th International Coference on Interna-
tional Conference on Machine Learning, pages 1363–
1370. Omnipress.
Paisley, J., Wang, C., Blei, D. M., and Jordan, M. I. (2015).
Nested hierarchical dirichlet processes. IEEE trans-
actions on pattern analysis and machine intelligence,
37(2):256–270.
Ranganath, R., Gerrish, S., and Blei, D. (2014). Black box
variational inference. In Artificial Intelligence and
Statistics, pages 814–822.
Rezende, D. J. and Mohamed, S. (2015). Variational in-
ference with normalizing flows. In Proceedings of
the 32nd International Conference on International
Conference on Machine Learning-Volume 37, pages
1530–1538. JMLR. org.
Robbins, H. and Monro, S. (1985). A stochastic approxi-
mation method. In Herbert Robbins Selected Papers,
pages 102–109. Springer.
Sethuraman, J. (1994). A constructive definition of dirichlet
priors. Statistica sinica, pages 639–650.
Titterington, D. M. (2011). The em algorithm, variational
approximations and expectation propagation for mix-
tures. Mixtures: Estimation and Applications, 896.
Wang, X., Lu, L., Shin, H.-C., Kim, L., Bagheri, M.,
Nogues, I., Yao, J., and Summers, R. M. (2017). Un-
supervised joint mining of deep features and image
labels for large-scale radiology image categorization
and scene recognition. In Applications of Computer
Vision (WACV), 2017 IEEE Winter Conference on,
pages 998–1007. IEEE.
Welling, M. and Teh, Y. W. (2011). Bayesian learning via
stochastic gradient langevin dynamics. In Proceed-
ings of the 28th International Conference on Machine
Learning (ICML-11), pages 681–688.
ICPRAM 2020 - 9th International Conference on Pattern Recognition Applications and Methods
42