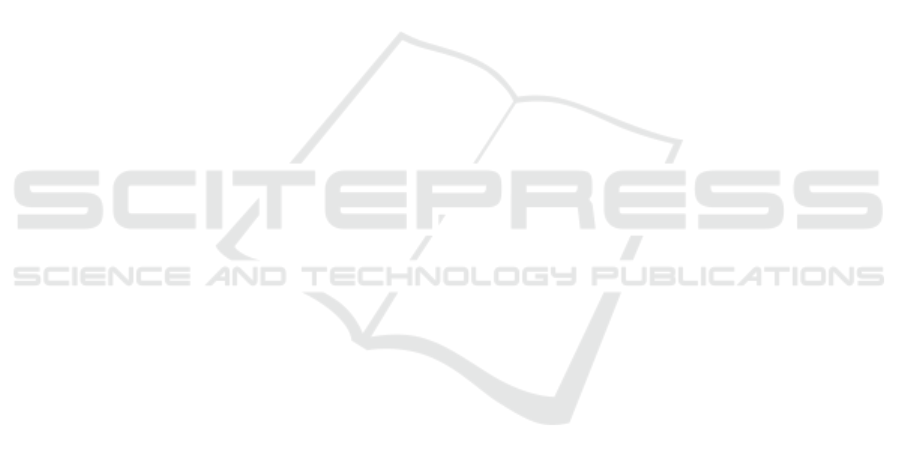
Chong, Y. S. and Tay, Y. H. (2017). Abnormal event de-
tection in videos using spatiotemporal autoencoder.
inInternational Symposium on Neural Networks, pp.
189–196, Springer.
C.Piciarelli, C. G. (2008). a trajectory-based anomalous
event detection,. Trajectory-based anomalous event
detection,”IEEE Transactions on Circuits and Systems
for video Technology, vol. 18, no. 11, pp. 1544–1554,.
C.Szegedy, W. Y. P. S. D. D. V. A. (2015). Going
deeper with convolutions. in Proceedings of the IEEE
confe-rence on computer vision and pattern recogni-
tion,pp.1#9.
D.-S. Pham, B. S. D. Q. P. S. V. (2011). Detection of
cross-channel anomalies from multiple data channels.
nICDM,2011, pp. 527–536.
D.Xu, E. Y. J. N. (2015). Learning deep representations of
appearance and motion for anomalous event detection.
arXiv preprint arXiv:1510.01553.
Hung Vu, T. D. N. D. P. (2018). Detection of un-
known anomalies in streaming videos with genera-
tive energy-based boltzmann models. arXiv preprint
arXiv:1805.01090.
I.Goodfellow, J.-A. M. B. D.-F. S. A. Y. (2014). Generative
adversarial nets. in Advances in neural information
processing systems ,pp.2672#2680.
J.Sun, J. C. H. (2017). Abnormal event detection for video
surveillance using deep one-class learning. Multime-
dia Tools and Applications, pp. 1–15,.
K.He, X. S. J. (2016). Deep residual learning for image
recognition. in Proceedings of the IEEE conference on
computer vision and pattern recognition,pp.770#778.
K.Simonyan, A. (2014). Very deep convolutional networks
for large-scale image recognition. ar Xiv preprint
arXiv:1409.1556.
M. Hasan, J. C. J. N. A. K. R.-C. L. S. D. (2016). “learn-
ing temporal regularity in video sequences,. in CVPR,
2016.
M. Ribeiro, A. E. L. H. S. L. (2017). A study of deep
convolutional auto-encoders for anomaly detection in
videos. Pattern Recognition Letters.
M. Sabokrou, M. F. M. F. R. K. (2017). Deep-
cascade:cascading 3d deep neural networks for fast
anomaly detection and localization in crowded scenes.
IEEE Transactions on Image Processing, vol. 26, no.
4, pp. 1992–2004.
M.Hasan, J. J. A.-C. L. (2017). Learning temporal regular-
ity in video sequences. in Proceedings of the IEEE
conference on computer vision and pattern recogni-
tion,pp.733#742.
M.Ravanbakhsh, M. E. L. C. N. (2017). Abnormal event
detection in videos using generative adversarial nets.
in 2017 IEEE International Conferenceon Image Pro-
cessing (ICIP),pp.1577#1581,IEEE, 2017.
M.Ravanbakhsh, M. H. E. N. S. Z. A. A. M. a. (2018).
Plug-and-play cnn for crowd motion analysis:an ap-
plication in abnormal event detection. in 2018 IEEE
Winter Conference on Applications of Computer Vi-
sion (WACV),pp.1689#1698,IEEE.
M.Sabokrou, M. M. R. M. J. E. (2018a). Avid: Adver-
sarial visual irregularity detection. arXiv preprint
arXiv:1805.09521.
M.Sabokrou, M. M. Z. R. (2018b). Deep-anomaly :fully
convolutional neural network for fast anomaly detec-
tion in crowded scenes. Computer Vision and Image
Understanding,vol.172,pp.88#97.
N.Sebe, M. M. E. L. M. C. R. (2017). Abnormal event
detection in videos using generative adversarial nets.
in Image Processing (ICIP), 2017 IEEE International-
Conference on, pp. 1577–1581, IEEE,.
O.Russakovsky, J. H. J. S. S. Z. A. A. M. a.
(2015). Imagenet large scale visual recognition
challenge. International journal of computer vision
,vol.115,no.3,pp.211#252.
P.Isola, J.-Y. T. A. (2017). Image-to-image translation with
conditional adversarial networks. n Proceedings of
the IEEE conference on computer vision and pattern
recognition ,pp.1125#1134.
P.Sermanet, D. X. M. R. Y. (2013). Overfeat :integrated
recognition ,localization and detection using convolu-
tional networks. arXiv preprint arXiv:1312.6229.
R.Hinami, T. S. (2017). Joint detection and recounting of
abnormal events by learning deep generic knowledge.
in Proceedings of the IEEE International Conference
on Computer Vision,pp.3619#3627.
S.Hamdi, S. K. H. M. (2019). Hybrid deep learning and hof
for anomaly detection. 2019 6th International Confer-
ence on Control, Decision and Information Technolo-
gies (CoDIT), IEEE.
S.Xingjian, Z. H. D. W. W. (2015). Convolutional lstm net-
work :a machine learning approach for precipitation
now casting. in Advances in neural information pro-
cessing systems,pp.802#810.
S.Zhou, W. D. Z. (2015). Unusual event detection in
crowded scenes by trajectory analysis. in Acoustics,
Speech and Signal Processing (ICASSP), 2015 IEEE
International Conference on,pp. 1300–1304, IEEE,.
S.Zhou, W.Shen D.Zeng M.Fang, Y. Z. (2016). Spa-
tial–temporal convolutional neural networks for
anomaly detection and localization in crowded scenes.
Signal Processing: Image Communication, vol. 47,
pp. 358–368.
V.Mahadevan, W. V. N. (2010). Anomaly detection in
crowded scenes. in Computer Vision and Pattern
Recognition (CVPR), 2010 IEEE Conference on, pp.
1975–1981, IEEE.
V.Reddy, C. B. (2011). Improved anomaly detectionin
crowded scenes via cell-based analysis of foreground
speed, sizeand texture. in Computer Vision and Pat-
tern Recognition Workshops(CVPRW), 2011 IEEE
Computer Society Conference on, pp. 55–61,IEEE.
W.Li, V. N. (2014). Anomaly detection and localization in
crowded scenes,. IEEE transactions on pattern analy-
sis and machine intelligence, vol. 36, no. 1, pp. 18–32.
W.Liu, W. D. S. (2018). Future frame prediction for
anomaly detection a new base line. in Proceedings of
the IEEE Conference on Computer Vision and Pattern
Recognition ,pp.6536#6545.
Y.Gong, S. A. F. (2013). A procrustean approach to learn-
ing binary codes for large-scale image retrieval. IEEE
transactions on pattern analysis and machine intelli-
gence ,vol.35,no.12,pp.2916#2929.
ICAART 2020 - 12th International Conference on Agents and Artificial Intelligence
520