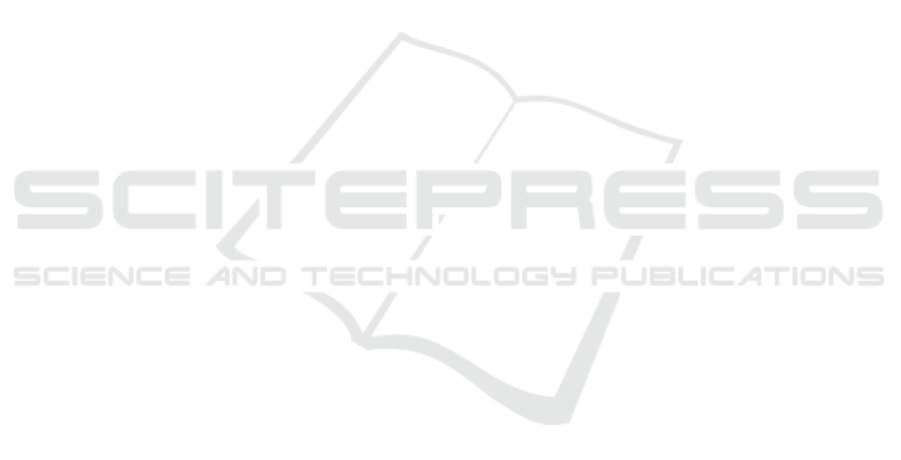
corpus statistics for a multilingual representation of
concepts and entities. Artificial Intelligence, 240:36–
64.
Chang, K., Yih, W., and Meek, C. (2013). Multi-relational
latent semantic analysis. In Proceedings of the
2013 Conference on Empirical Methods in Natural
Language Processing, EMNLP 2013, 18-21 October
2013, Grand Hyatt Seattle, Seattle, Washington, USA,
A meeting of SIGDAT, a Special Interest Group of the
ACL, pages 1602–1612.
Chen, T., Xu, R., He, Y., and Wang, X. (2015). Improving
distributed representation of word sense via wordnet
gloss composition and context clustering. In Proceed-
ings of the 53rd Annual Meeting of the Association for
Computational Linguistics and the 7th International
Joint Conference on Natural Language Processing of
the Asian Federation of Natural Language Processing,
ACL 2015, July 26-31, 2015, Beijing, China, Volume
2: Short Papers, pages 15–20.
Erk, K. and Pad
´
o, S. (2008). A structured vector space
model for word meaning in context. In Proceedings
of the 2008 Conference on Empirical Methods in Nat-
ural Language Processing, pages 897–906.
Faruqui, M., Dodge, J., Jauhar, S. K., Dyer, C., Hovy, E. H.,
and Smith, N. A. (2015). Retrofitting word vectors to
semantic lexicons. In NAACL HLT 2015, The 2015
Conference of the North American Chapter of the As-
sociation for Computational Linguistics: Human Lan-
guage Technologies, Denver, Colorado, USA, May 31
- June 5, 2015, pages 1606–1615.
Faruqui, M., Tsvetkov, Y., Rastogi, P., and Dyer, C. (2016).
Problems with evaluation of word embeddings using
word similarity tasks. In Proceedings of the 1st Work-
shop on Evaluating Vector-Space Representations for
NLP, pages 30–35, Berlin, Germany. Association for
Computational Linguistics.
Finkelstein, L., Gabrilovich, E., Matias, Y., Rivlin, E.,
Solan, Z., Wolfman, G., and Ruppin, E. (2002). Plac-
ing search in context: the concept revisited. ACM
Trans. Inf. Syst., 20(1):116–131.
Ganitkevitch, J., Van Durme, B., and Callison-Burch, C.
(2013). Ppdb: The paraphrase database. In Proceed-
ings of the 2013 Conference of the North American
Chapter of the Association for Computational Lin-
guistics: Human Language Technologies, pages 758–
764.
Gerz, D., Vulic, I., Hill, F., Reichart, R., and Korhonen, A.
(2016). Simverb-3500: A large-scale evaluation set of
verb similarity. In Proceedings of the 2016 Confer-
ence on Empirical Methods in Natural Language Pro-
cessing, EMNLP 2016, Austin, Texas, USA, November
1-4, 2016, pages 2173–2182.
Goikoetxea, J., Soroa, A., and Agirre, E. (2015). Random
walks and neural network language models on knowl-
edge bases. In NAACL HLT 2015, The 2015 Confer-
ence of the North American Chapter of the Associa-
tion for Computational Linguistics: Human Language
Technologies, Denver, Colorado, USA, May 31 - June
5, 2015, pages 1434–1439.
Grave, E., Mikolov, T., Joulin, A., and Bojanowski, P.
(2017). Bag of tricks for efficient text classification.
In Proceedings of the 15th Conference of the Euro-
pean Chapter of the Association for Computational
Linguistics, EACL 2017, Valencia, Spain, April 3-7,
2017, Volume 2: Short Papers, pages 427–431.
Gutmann, M. and Hyv
¨
arinen, A. (2010). Noise-contrastive
estimation: A new estimation principle for unnormal-
ized statistical models. In Proceedings of the Thir-
teenth International Conference on Artificial Intelli-
gence and Statistics, pages 297–304.
Harris, Z. S. (1954). Distributional structure. Word, 10(2-
3):146–162.
Hill, F., Reichart, R., and Korhonen, A. (2015). Simlex-999:
Evaluating semantic models with (genuine) similar-
ity estimation. Computational Linguistics, 41(4):665–
695.
Huang, E. H., Socher, R., Manning, C. D., and Ng, A. Y.
(2012). Improving word representations via global
context and multiple word prototypes. In Proceed-
ings of the 50th Annual Meeting of the Association
for Computational Linguistics: Long Papers-Volume
1, pages 873–882. Association for Computational Lin-
guistics.
Kiela, D., Hill, F., and Clark, S. (2015). Specializing word
embeddings for similarity or relatedness. In Proceed-
ings of the 2015 Conference on Empirical Methods in
Natural Language Processing, EMNLP 2015, Lisbon,
Portugal, September 17-21, 2015, pages 2044–2048.
Lee, Y., Yen, T., Huang, H., Shiue, Y., and Chen, H. (2018).
Gensense: A generalized sense retrofitting model. In
Proceedings of the 27th International Conference on
Computational Linguistics, COLING 2018, Santa Fe,
New Mexico, USA, August 20-26, 2018, pages 1662–
1671.
Lengerich, B. J., Maas, A. L., and Potts, C. (2018).
Retrofitting distributional embeddings to knowledge
graphs with functional relations. In Proceedings of the
27th International Conference on Computational Lin-
guistics, COLING 2018, Santa Fe, New Mexico, USA,
August 20-26, 2018, pages 2423–2436.
Li, J. and Jurafsky, D. (2015). Do multi-sense embed-
dings improve natural language understanding? arXiv
preprint arXiv:1506.01070.
Li, W. and McCallum, A. (2005). Semi-supervised se-
quence modeling with syntactic topic models. In
AAAI, volume 5, pages 813–818.
Liu, Q., Jiang, H., Wei, S., Ling, Z., and Hu, Y. (2015a).
Learning semantic word embeddings based on ordi-
nal knowledge constraints. In Proceedings of the 53rd
Annual Meeting of the Association for Computational
Linguistics and the 7th International Joint Conference
on Natural Language Processing of the Asian Federa-
tion of Natural Language Processing, ACL 2015, July
26-31, 2015, Beijing, China, Volume 1: Long Papers,
pages 1501–1511.
Liu, Y., Liu, Z., Chua, T.-S., and Sun, M. (2015b). Topical
word embeddings. In Twenty-Ninth AAAI Conference
on Artificial Intelligence.
Mikolov, T., Chen, K., Corrado, G., and Dean, J. (2013a).
Efficient estimation of word representations in vector
space. CoRR, abs/1301.3781.
Mikolov, T., Grave, E., Bojanowski, P., Puhrsch, C., and
Joulin, A. (2018). Advances in pre-training distributed
ICAART 2020 - 12th International Conference on Agents and Artificial Intelligence
118