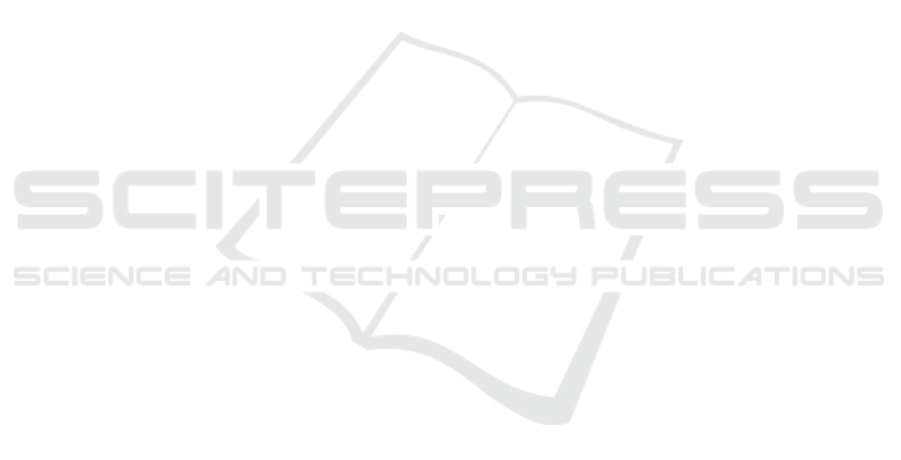
ACKNOWLEDGEMENTS
We thank Gaelle Bonnet-Loosli for providing support
with indefinite learning and R. Duin, Delft University
for variety support with DisTools and PRTools.
FMS, MM are supported by the ESF program
WiT-HuB/2014-2020, project IDA4KMU, StMBW-W-
IX.4-170792.
FMS, CR are supported by the FuE program of
the StMWi,project OBerA, grant number IUK-1709-
0011// IUK530/010.
REFERENCES
Alabdulmohsin, I. M., Ciss
´
e, M., Gao, X., and Zhang, X.
(2016). Large margin classification with indefinite
similarities. Machine Learning, 103(2):215–237.
Chen, H., Tino, P., and Yao, X. (2009). Probabilistic classi-
fication vector machines. IEEE Transactions on Neu-
ral Networks, 20(6):901–914.
Duin, R. P. W. and Pekalska, E. (2010). Non-euclidean
dissimilarities: Causes and informativeness. In
SSPR&SPR 2010, pages 324–333.
Filippone, M. (2009). Dealing with non-metric dissimilar-
ities in fuzzy central clustering algorithms. Int. J. of
Approx. Reasoning, 50(2):363–384.
Gu, S. and Guo, Y. (2012). Learning SVM classifiers with
indefinite kernels. In Proc. of the 26th AAAI Conf. on
AI, July 22-26, 2012.
Gusfield, D. (1997). Algorithms on Strings, Trees, and Se-
quences: Computer Science and Computational Biol-
ogy. Cambridge University Press.
Haasdonk, B. (2005). Feature space interpretation of SVMs
with indefinite kernels. IEEE TPAMI, 27(4):482–492.
Haasdonk, B. and Pekalska, E. (2008). Indefinite kernel
fisher discriminant. In 19th International Conference
on Pattern Recognition (ICPR 2008), December 8-11,
2008, Tampa, Florida, USA, pages 1–4. IEEE Com-
puter Society.
Higham, N. (1988). Computing a nearest symmetric posi-
tive semidefinite matrix. Linear Algebra and Its Ap-
plications, 103(C):103–118.
Hodgetts, C. and Hahn, U. (2012). Similarity-based asym-
metries in perceptual matching. Acta Psychologica,
139(2):291–299.
Ilic, M., Turner, I. W., and Saad, Y. (2007). Linear system
solution by null-space approximation and projection
(SNAP). Numerical Lin. Alg. with Applic., 14(1):61–
82.
Jain, A. and Zongker, D. (1997). Representation and recog-
nition of handwritten digits using deformable tem-
plates. IEEE Transactions on Pattern Analysis and
Machine Intelligence, 19(12):1386–1391.
Kohonen, T. and Somervuo, P. (2002). How to make large
self-organizing maps for nonvectorial data. Neural
Netw., 15(8-9):945–952.
Laub, J. (2004). Non-metric pairwise proximity data. PhD
thesis, Berlin Institute of Technology.
Ling, H. and Jacobs, D. W. (2007). Shape classification
using the inner-distance. IEEE Trans. Pattern Anal.
Mach. Intell., 29(2):286–299.
Loosli, G. (2019). Trik-svm: an alternative decomposition
for kernel methods in krein spaces. In Verleysen, M.,
editor, In Proceedings of the 27th European Sympo-
sium on Artificial Neural Networks (ESANN) 2019,
pages 79–94, Evere, Belgium. d-side publications.
Loosli, G., Canu, S., and Ong, C. S. (2016). Learning svm
in krein spaces. IEEE Transactions on Pattern Analy-
sis and Machine Intelligence, 38(6):1204–1216.
Luss, R. and d’Aspremont, A. (2009). Support vector ma-
chine classification with indefinite kernels. Mathemat-
ical Programming Computation, 1(2-3):97–118.
Mises, R. V. and Pollaczek-Geiringer, H. (1929). Praktische
verfahren der gleichungsaufl
¨
osung . ZAMM - Journal
of Applied Mathematics and Mechanics / Zeitschrift
f
¨
ur Angewandte Mathematik und Mechanik, 9(2):152–
164.
Mokbel, B. (2016). Dissimilarity-based learning for com-
plex data. PhD thesis, Bielefeld University.
Musco, C. and Woodruff, D. P. (2017). Sublinear time low-
rank approximation of positive semidefinite matrices.
CoRR, abs/1704.03371.
Neuhaus, M. and Bunke, H. (2006). Edit distance based ker-
nel functions for structural pattern classification. Pat-
tern Recognition, 39(10):1852–1863.
Pekalska, E. and Duin, R. (2005). The dissimilarity repre-
sentation for pattern recognition. World Scientific.
Pekalska, E., Harol, A., Duin, R. P. W., Spillmann, B., and
Bunke, H. (2006). Non-euclidean or non-metric mea-
sures can be informative. In Structural, Syntactic, and
Statistical Pattern Recognition, Joint IAPR Interna-
tional Workshops, SSPR 2006 and SPR 2006, Hong
Kong, China, August 17-19, 2006, Proceedings, pages
871–880.
Sakoe, H. and Chiba, S. (1978). Dynamic program-
ming algorithm optimization for spoken word recog-
nition. Acoustics, Speech and Signal Processing,
IEEE Transactions on, 26(1):43–49.
Sanyal, A., Kanade, V., and Torr, P. H. S. (2018). Low
rank structure of learned representations. CoRR,
abs/1804.07090.
Scheirer, W. J., Wilber, M. J., Eckmann, M., and Boult,
T. E. (2014). Good recognition is non-metric. Pat-
tern Recognition, 47(8):2721–2731.
Schleif, F. and Ti
˜
no, P. (2015). Indefinite proximity learn-
ing: A review. Neural Computation, 27(10):2039–
2096.
Schnitzer, D., Flexer, A., and Widmer, G. (2012). A fast
audio similarity retrieval method for millions of music
tracks. Multimedia Tools and Appl., 58(1):23–40.
Shawe-Taylor, J. and Cristianini, N. (2004). Kernel Meth-
ods for Pattern Analysis and Discovery. Cambridge
University Press.
Strassen, V. (1969). Gaussian elimination is not optimal.
Numerische Mathematik, 13(4):354–356.
ICPRAM 2020 - 9th International Conference on Pattern Recognition Applications and Methods
50