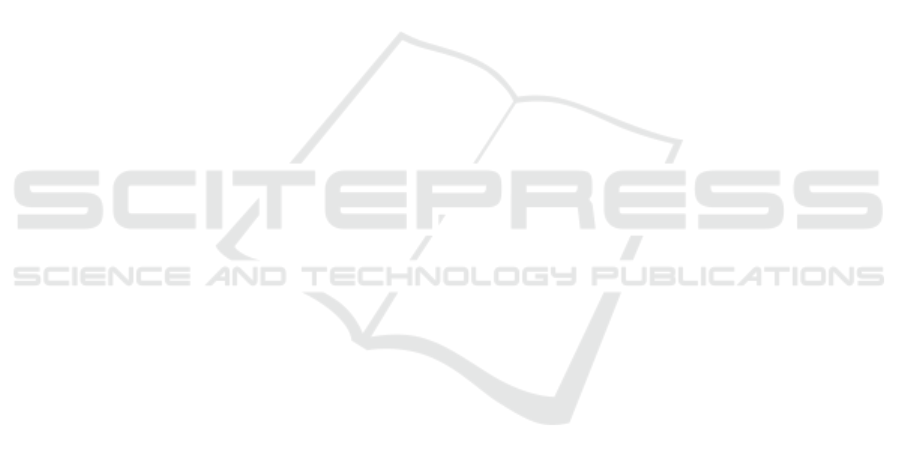
both positively and negatively, enabling yet another
support tool for teachers.
The experiment demonstrated an efficiency in
using uses Multi-Agent System as technology for the
proposed approach, considering the proactivity and
communication of the agents, another highlight is
the use of graphics with the emotional state that
facilitates interpretation by teachers.
As a future work, we intend to develop a system
integrated with the Virtual Environment that
conducts Sentiment Analysis in real time, presents
different graphs and checks the emotional state of
each student and each class.
REFERENCES
Alencar, M.A.S.; Netto, J.F.M. (2011) Improving
Cooperation in Virtual Learning Environments Using
Multi- Agent Systems and AIML. Frontiers in
Education Conference - FIE.
Alencar, M.A.S.; Netto, J.F.M. (2017) Melhorando a
Colaboração de um Ambiente Virtual de
Aprendizagem usando um Agente Pedagógico
Animado 3D. XXVIII Brazilian Symposium on
Informatics in Education, v. 1, p. 1417, 2017.
Cambria, E.; Poria, S.; Hazarika, D.; Kwok, K. SenticNet
5: Discovering Conceptual Primitives for Sentiment
Analysis by Means of Context Embeddings. In: AAAI,
pp. 1795-1802 (2018).
Cercel, D.;Trausan-Matu1, S. Modeling Post-Level
Sentiment Evolution in Online Forum Threads. In:
Proceedings of the 7th International ICAART
Conference on Agents and Artificial Intelligence,
Lisbon, Portugal (2015).
Chikersal P.; Poria, S.; Cambria, E. (2015) SeNTU:
Sentiment Analysis of Tweets by Combining a Rule-
Based Classifier with Supervised Learning. In:
Proceedings of the international workshop on semantic
evaluation. Denver, Colorado, USA, SemEval 2015.
Dorri, A; Kanhere, S. S. ; Jurdak, R. Multi-Agent
Systems: A survey. IEEE Access, vol. 6, pp. 28573–
28593, Jul. 2018.
Dragoni, M; Da, C.; Pereira, C.; Tettamanzi, A.G.; Villata,
S. Smack: An argumentation framework for opinion
mining, Proceedings of the Twenty- Fifth International
Joint Conference on Artificial Intelligence, pp.9-15,
2016.
Deloach, S. A.; Wood, M. Developing Multiagent Systems
with agentTool. In: Proceedings of Lecture Notes in
Artificial Intelligence. Springer – Verlag. Berlin, 2001.
Fei, H., Li, H. (2018). The Study of Learners’ Emotional
Analysis Based on MOOC. In: Xiao J., Mao
ZH.,Suzumura T., Zhang L. J. (eds) Cognitive
Computing – ICCC 2018. ICCC 2018. Lecture Notes
in Computer Science, vol 10971. Springer, Cham
Fontes, L. M. O.; Valentim, R. A. M.; Neto, F. M. M.;
Souza, R. C. A. Multi-Agent Architecture for
Monitoring Tutoring Activities. In VLEs. IEEE Latin
America Transactions, vol. 14, no. 10, pp. 4327-4333,
2016.
Gontzis, A.F.; Karachristos, C.V.; Panagiotakopoulos,
C.T.; Stavropoulos, E.C.; Verykios, V.S. Sentiment
Analysis to track Emotion and Polarity in Student
Fora, Proc. of PCI 2017.
Grawemeyer, B.; Mavrikis, M.; Holmes, W.; Gutiérrez-
Santos, S.; Wiedmann, M.; Rummel, N. (2017).
Affective Learning: Improving Engagement and
Enhancing Learning with Affect-Aware
Feedback. User Modeling and User-Adapted
Interaction, 27(1), 119–158.
Guo, S.; H¨ohn, S.; Xu, F.; Schommer, C.: PERSEUS: A
Personalization Framework for Sentiment
Categorization With Recurrent Neural Network. In:
International Conference on Agents and Artificial
Intelligence, Funchal 16-18 January 2018. p. 9 (2018)
Kumi-Yeboah, A.; Dogbey, J.; Yuan, G. (2017). Online
Collaborative Learning Activities: The Perceptions of
Culturally Diverse Graduate Students. Online
Learning, 21(4), 5-28. doi: 10.24059/olj.v21i4.1277
Lima, D. P. R.; Gerosa, M. A.; Netto, J. F. M. Using
Awareness Information to Enhance Online Discussion
Forums: A Systematic Mapping Study. In: Frontiers in
Education, 2018, San Jose, CA. 2018 Frontiers in
Education Conference - Fostering Innovation through
Diversity, 2018.
Liu, B. Opinions, Sentiment, and Emotion in Text.
Cambridge University Press, p. 381, 2015.
Madhok, R; Goel, S; Garg, S. SentiMozart: Music
Generation based on Emotions. In: Proceedings of the
10th International ICAART Conference on Agents and
Artificial Intelligence, Madeira, Portugal (2018).
Mohammad, S. M. (2016). Sentiment Analysis: Detecting
Valence, Emotions, and Other Affectual States from
text. Emotion Measurement
Pang, Bo; Lee, L. (2008), Opinion Mining and Sentiment
Analysis, Foundations and Trends in Information
Retrieval: Vol. 2: No. 1–2, pp 1-135
Picard, R.W. (2003). Affective Computing: Challenges.
International Journal of Human-Computer Studies,
Volume 59, Issues 1-2, July 2003, pp. 55-64.
Piryani, R. ; Madhavi, D; Singh, V. K. Analytical
Mapping of Opinion Mining and Sentiment Analysis
Research During 2000-2015, Inf. Process. Manag.,
vol. 53, no. 1, pp. 122-150, 2016.
Plutchik, R. (1984). Emotions: A General
Psychoevolutionary Theory. Approaches to Emotion,
1984, 197-219.
Poria, S.; Cambria, E.; Bajpai , R.; Hussain, A. 2017. A
Review of Affective Computing: From Unimodal
Analysis to Multimodal Fusion. Information Fusion.
Rodrigues, R.G.; das Dores, R.M.; Camilo-Junior, C.G.;
Rosa, T.C. SentiHealth-Cancer: a Sentiment Analysis
Tool to Help Detecting mood of patients in online
social networks. Int. J. Med. Inform. 85, 80–95 (2016)
Roorda, D. L., Jak, S., Zee, M., Oort, F. J., & Koomen, H.
M. Y. (2017). Affective Teacher–Student
Relationships and Students’ Engagement and
ICAART 2020 - 12th International Conference on Agents and Artificial Intelligence
568