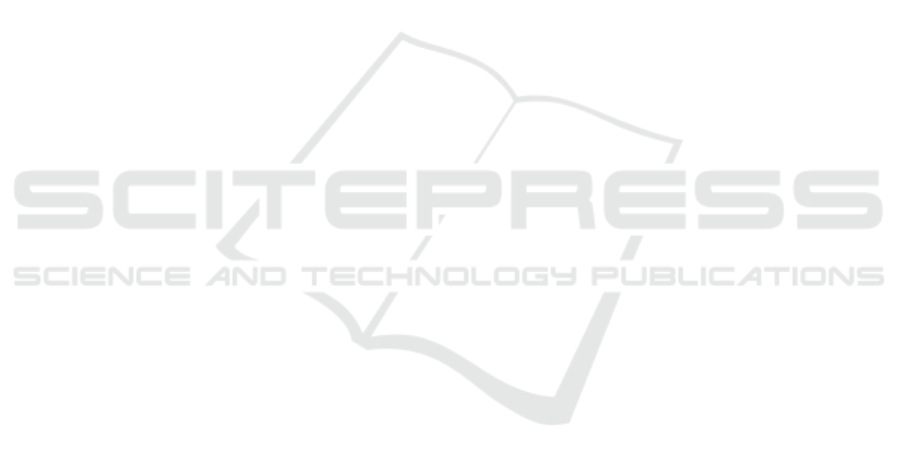
the val label without considering spontaneity is 0.761,
but when considering MAE, it is 0.749. Therefore, by
considering spontaneity, we were able to show a con-
tribution to emotion estimation.
6 FUTURE WORKS
In this study, we experimented with three sim-
ple layers of DNN. However, if the network was
changed, the optimal hyperparameter settings could
also change. Moreover, we simply decided hyper-
parameters by experiments, hence it will be a future
work to use known optimization algorithms to decide
them. Further, we used a normal distribution with
variance 1 as the distribution for measuring KL di-
vergence, but it may be possible to improve the ac-
curacy of emotion estimation by changing the vari-
ance and verifying the change in VAT accuracy. Fur-
thermore, in this study, we conducted a cross-corpus
experiment between Japanese and English, however
as a future task, we will investigate the improvement
of robustness by VAT by conducting a cross-corpus
experiment in other languages and culture areas. In
addition, in this study, we compared the estimation
accuracy using IEMOCAP. IEMOCAP was used for
both training and evaluating. Therefore it is consid-
ered future works to evaluate the contribution of esti-
mation accuracy considering spontaneity using other
language corpora. Finally, we need to conduct sub-
jective assessment experiments to understand how the
estimation error affects the human perception.
ACKNOWLEDGEMENTS
This work was supported by JSPS KAKENHI Grant
Numbers JP17H04705, JP18H03229, JP18H03340,
18K19835, JP19H04113, JP19K12107.
REFERENCES
Busso, C., Bulut, M., Lee, C.-C., Kazemzadeh, A., Mower,
E., Kim, S., Chang, J. N., Lee, S., and Narayanan,
S. S. (2008). Iemocap: Interactive emotional dyadic
motion capture database. Language resources and
evaluation, 42(4):335.
Dufour, R., Estève, Y., and Deléglise, P. (2014). Character-
izing and detecting spontaneous speech: Application
to speaker role recognition. Speech communication,
56:1–18.
Dufour, R., Jousse, V., Estève, Y., Béchet, F., and Linarès,
G. (2009). Spontaneous speech characterization and
detection in large audio database. SPECOM, St. Pe-
tersburg.
Eyben, F., Wöllmer, M., and Schuller, B. (2010). Opens-
mile: the munich versatile and fast open-source au-
dio feature extractor. In Proceedings of the 18th ACM
international conference on Multimedia, pages 1459–
1462. ACM.
Goodfellow, I. J., Shlens, J., and Szegedy, C. Explaining
and harnessing adversarial examples. corr (2015).
Han, K., Yu, D., and Tashev, I. (2014). Speech emotion
recognition using deep neural network and extreme
learning machine. In Fifteenth annual conference of
the international speech communication association.
Kamaruddin, N., Wahab, A., and Quek, C. (2012). Cultural
dependency analysis for understanding speech emo-
tion. Expert Systems with Applications, 39(5):5115–
5133.
Kim, J., Englebienne, G., Truong, K. P., and Evers, V.
(2017). Towards speech emotion recognition" in the
wild" using aggregated corpora and deep multi-task
learning. arXiv preprint arXiv:1708.03920.
Kim, J., Lee, S., and Narayanan, S. S. (2010). An ex-
ploratory study of manifolds of emotional speech. In
Acoustics Speech and Signal Processing (ICASSP),
2010 IEEE International Conference on, pages 5142–
5145. IEEE.
Kuwahara, T., Sei, Y., Tahara, Y., Orihara, R., and Ohsuga,
A. (2019). Model smoothing using virtual adversarial
training for speech emotion estimation. In 2019 IEEE
International Conference on Big Data, Cloud Com-
puting, Data Science & Engineering (BCD), pages
60–64. IEEE.
Laukka, P. (2005). Categorical perception of vocal emotion
expressions. Emotion, 5(3):277.
Laukka, P., Juslin, P., and Bresin, R. (2005). A dimensional
approach to vocal expression of emotion. Cognition
& Emotion, 19(5):633–653.
Li, L., Zhao, Y., Jiang, D., Zhang, Y., Wang, F., Gonzalez,
I., Valentin, E., and Sahli, H. (2013). Hybrid deep neu-
ral network–hidden markov model (dnn-hmm) based
speech emotion recognition. In Affective Computing
and Intelligent Interaction (ACII), 2013 Humaine As-
sociation Conference on, pages 312–317. IEEE.
Mangalam, K. and Guha, T. (2017). Learning spontane-
ity to improve emotion recognition in speech. arXiv
preprint arXiv:1712.04753.
Miyato, T., Maeda, S.-i., Ishii, S., and Koyama, M. (2018).
Virtual adversarial training: a regularization method
for supervised and semi-supervised learning. IEEE
transactions on pattern analysis and machine intelli-
gence.
Miyato, T., Maeda, S.-i., Koyama, M., Nakae, K., and Ishii,
S. (2015). Distributional smoothing with virtual ad-
versarial training. arXiv preprint arXiv:1507.00677.
Mori, H., Satake, T., Nakamura, M., and Kasuya, H. (2011).
Constructing a spoken dialogue corpus for studying
paralinguistic information in expressive conversation
and analyzing its statistical/acoustic characteristics.
Speech Communication, 53(1):36–50.
ICAART 2020 - 12th International Conference on Agents and Artificial Intelligence
576