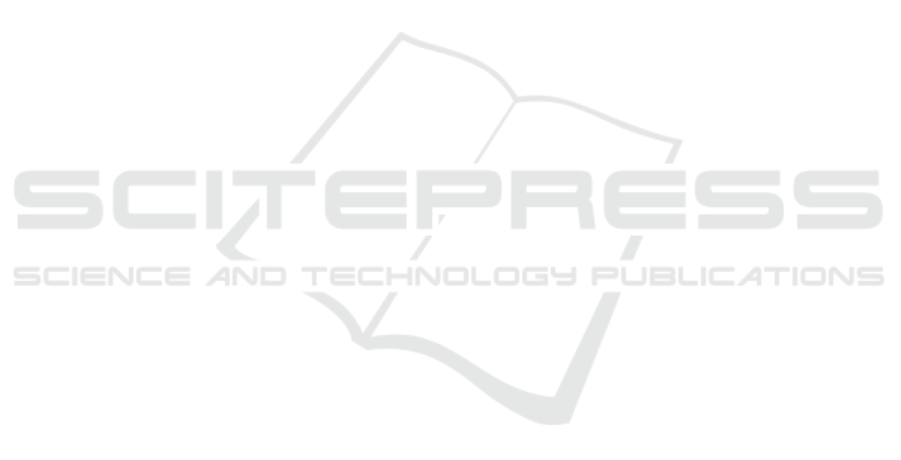
a closer place near the global optima. To evaluate the
proposed approaches, 23 medical datasets were used
from well regarded data repositories including UCI,
Keel and Kaggle. The comparative results showed
that the chaotic operators have enhanced the perfor-
mance of the standard BMFO when used to optimize
the feature search space. For the future, the research
line of metaheuristic based wrapper methods can be
continued by proposing new modification strategies
and adopting other metahueristic algorithms to exam-
ine feature space.
REFERENCES
Al-Madi, N., Faris, H., and Abukhurma, R. (2018).
Cost-sensitive genetic programming for churn predic-
tion and identification of the influencing factors in
telecommunication market. International Journal of
Advanced Science and Technology, pages 13–28.
Alcalá-Fdez, J., Fernández, A., Luengo, J., Derrac, J., Gar-
cía, S., Sánchez, L., and Herrera, F. (2011). Keel data-
mining software tool: data set repository, integration
of algorithms and experimental analysis framework.
Journal of Multiple-Valued Logic & Soft Computing,
17.
Asuncion, A. and Newman, D. (2007). Uci machine learn-
ing repository.
Chuang, L.-Y., Yang, C.-S., Wu, K.-C., and Yang, C.-
H. (2011). Gene selection and classification using
taguchi chaotic binary particle swarm optimization.
Expert Systems with Applications, 38(10):13367–
13377.
Dash, M. and Liu, H. (1997). Feature selection for classifi-
cation. Intelligent data analysis, 1(1-4):131–156.
dos Santos Coelho, L. and Mariani, V. C. (2008). Use of
chaotic sequences in a biologically inspired algorithm
for engineering design optimization. Expert Systems
with Applications, 34(3):1905–1913.
Emary, E., Zawbaa, H. M., and Hassanien, A. E. (2016).
Binary ant lion approaches for feature selection. Neu-
rocomputing, 213:54–65.
Ewees, A. A., El Aziz, M. A., and Hassanien, A. E. (2019).
Chaotic multi-verse optimizer-based feature selection.
Neural Computing and Applications, 31(4):991–1006.
Faris, H., Abukhurma, R., Almanaseer, W., Saadeh, M.,
Mora, A. M., Castillo, P. A., and Aljarah, I. (2019).
Improving financial bankruptcy prediction in a highly
imbalanced class distribution using oversampling and
ensemble learning: a case from the spanish market.
Progress in Artificial Intelligence, pages 1–23.
Goldbloom, A., Hamner, B., Moser, J., and Cukierski, M.
(2017). Kaggle: your homr for data science.
Huang, C.-L. and Wang, C.-J. (2006). A ga-based fea-
ture selection and parameters optimizationfor support
vector machines. Expert Systems with applications,
31(2):231–240.
Jain, I., Jain, V. K., and Jain, R. (2018). Correlation feature
selection based improved-binary particle swarm opti-
mization for gene selection and cancer classification.
Applied Soft Computing, 62:203–215.
Kennedy, J. and Eberhart, R. C. (1997). A discrete bi-
nary version of the particle swarm algorithm. In 1997
IEEE International conference on systems, man, and
cybernetics. Computational cybernetics and simula-
tion, volume 5, pages 4104–4108. IEEE.
Khurma, R. A., Aljarah, I., Sharieh, A., and Mirjalili, S.
(2020). Evolopy-fs: An open-source nature-inspired
optimization framework in python for feature selec-
tion. In Evolutionary Machine Learning Techniques,
pages 131–173. Springer.
Mafarja, M. and Mirjalili, S. (2018). Whale optimization
approaches for wrapper feature selection. Applied Soft
Computing, 62:441–453.
Mirjalili, S. (2015). Moth-flame optimization algorithm: A
novel nature-inspired heuristic paradigm. Knowledge-
Based Systems, 89:228–249.
Mirjalili, S. and Lewis, A. (2013). S-shaped versus v-
shaped transfer functions for binary particle swarm
optimization. Swarm and Evolutionary Computation,
9:1–14.
Sayed, G. I., Darwish, A., and Hassanien, A. E. (2018a). A
new chaotic whale optimization algorithm for features
selection. Journal of classification, 35(2):300–344.
Sayed, G. I., Khoriba, G., and Haggag, M. H. (2018b). A
novel chaotic salp swarm algorithm for global opti-
mization and feature selection. Applied Intelligence,
48(10):3462–3481.
Wolpert, D. H., Macready, W. G., et al. (1997). No free
lunch theorems for optimization. IEEE transactions
on evolutionary computation, 1(1):67–82.
YANG, H.-c., ZHANG, S.-b., DENG, K.-z., and DU, P.-
j. (2007). Research into a feature selection method
for hyperspectral imagery using pso and svm. Jour-
nal of China University of Mining and Technology,
17(4):473–478.
ICPRAM 2020 - 9th International Conference on Pattern Recognition Applications and Methods
182