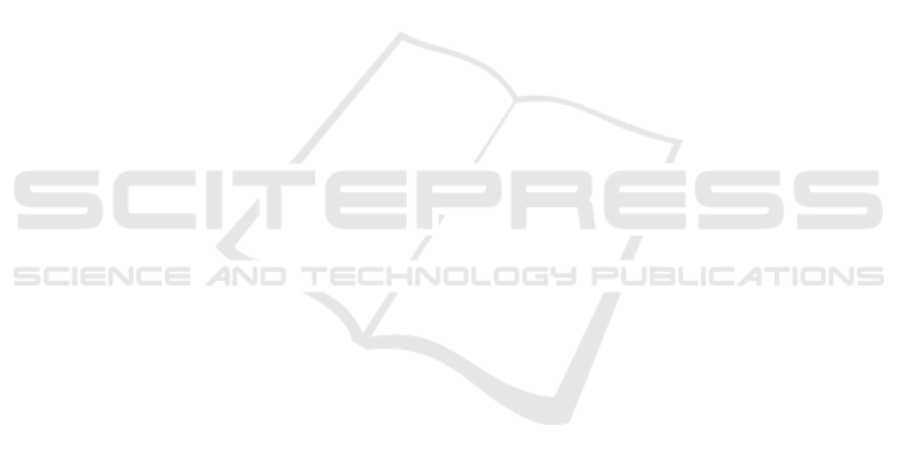
better. As we mentioned before this is caused by the
increased complexity of the discontinuous charging
model. From the point of comparison of the solution
time between different scenarios, the winter scenario
has increased computation time for both models. This
is caused by the decreased capacity of the battery.
In the table 5, there is a comparison of the count
of the charging intervals that were used for charg-
ing (UCI) during a specific scenario for each dataset.
From the results, we cannot say which type of charg-
ing uses less charging intervals. However, a change
can be seen in the number of charging events that
took multiple intervals (MIC). There the continuous
charging uses usually less number of multiple inter-
val charging events than the discontinuous charging,
but the count of the used interval during a multiple in-
terval charging was less in the case of discontinuous
charging. The last column IC shows the number of in-
terrupted charging events for discontinuous charging.
We can see that the number of interrupted charging
events is higher with the winter scenario.
5 CONCLUSION
In this paper we propose changes to the linear math-
ematical model, that would enable the discontinu-
ous charging. The new model was tested by IP
solver Xpress IVE and the results of the discontinuous
charging model were compared to the results of the
continuous charging model. From the results, we can
conclude, that in the case of minimizing the number
of the used electric vehicles on the selected datasets
the discontinuous charging model does not give bet-
ter results, moreover the computational time is higher.
Despite the obtained results, we see a potential
of the discontinuous charging model with the use of
different objective functions, for example minimizing
the length of deadheading trips between the service
trips respectively service trips and chargers. There-
fore, more experiments need to be conducted with the
presented model in the future, but with different ob-
jective functions. On the other hand, the proposed
models are complex and the solution time indicates
that the use of these models is not possible on large
scale problems. Therefore, the use of heuristics is ad-
vised on the larger-scale problems.
ACKNOWLEDGEMENTS
This work was supported by the research grants
VEGA 1/0089/19 ”Data analysis methods and de-
cisions support tools for service systems supporting
electric vehicles” and VEGA 1/0689/19 ”Optimal de-
sign and economically efficient charging infrastruc-
ture deployment for electric buses in public trans-
portation of smart cities”.
REFERENCES
Bunte, S. and Kliewer, N. (2009). An overview on vehicle
scheduling models. Public Transport, 1(4):299 – 317.
Chao, Z. and Xiaohong, C. (2013). Optimizing battery
electric bus transit vehicle scheduling with battery ex-
changing: Model and case study. Procedia - Social
and Behavioral Sciences, 96:2725 – 2736. Intelligent
and Integrated Sustainable Multimodal Transporta-
tion Systems Proceedings from the 13th COTA Inter-
national Conference of Transportation Professionals
(CICTP2013).
Czimmermann, P. (2006). On a certain transport schedul-
ing problem for heterogeneous bus fleet. Communica-
tions, pages 17–18.
Janovec, M. and Koh
´
ani, M. (2019a). Battery degradation
impact on the electric bus fleet scheduling. In 2019
International Conference on Information and Digital
Technologies (IDT), pages 190–197.
Janovec, M. and Koh
´
ani, M. (2019b). Exact approach to
the electric bus fleet scheduling. Transportation Re-
search Procedia, 40:1380 – 1387. TRANSCOM 2019
13th International Scientific Conference on Sustain-
able, Modern and Safe Transport.
Kim, J., Song, I., and Choi, W. (2015). An electric bus with
a battery exchange system. Energies, 8:6806–6819.
Millner, A. (2010). Modeling lithium ion battery degrada-
tion in electric vehicles. In 2010 IEEE Conference on
Innovative Technologies for an Efficient and Reliable
Electricity Supply, pages 349 – 356.
Rogge, M., van der Hurk, E., Larsen, A., and Sauer, D. U.
(2018). Electric bus fleet size and mix problem with
optimization of charging infrastructure. Applied En-
ergy, 211:282 – 295.
Sassi, O. and Oulamara, A. (2017). Electric vehicle
scheduling and optimal charging problem: complex-
ity, exact and heuristic approaches. International
Journal of Production Research, 55(2):519–535.
van Kooten Niekerk, M. E., van den Akker, J. M., and
Hoogeveen, J. A. (2017). Scheduling electric vehi-
cles. Public Transport, 9(1):155 – 176.
Wood, E., Neubauer, J., Brooker, A. D., Gonder, J., and
Smith, K. A. (2012). Variability of battery wear in
light duty plug-in electric vehicles subject to ambi-
ent temperature, battery size, and consumer usage:
Preprint. NREL Report No. CP-5400-53953.
Yanjin, H., Yong, Y., Zhizhen, L., and Linlin, S. (2016). A
discontinuous coordinated charging strategy for elec-
tric vehicles. In 2016 IEEE 11th Conference on In-
dustrial Electronics and Applications (ICIEA), pages
1099–1102.
ZeEUS project (2016). Zeeus ebus report: An overview of
electric buses in europe.
ICORES 2020 - 9th International Conference on Operations Research and Enterprise Systems
186