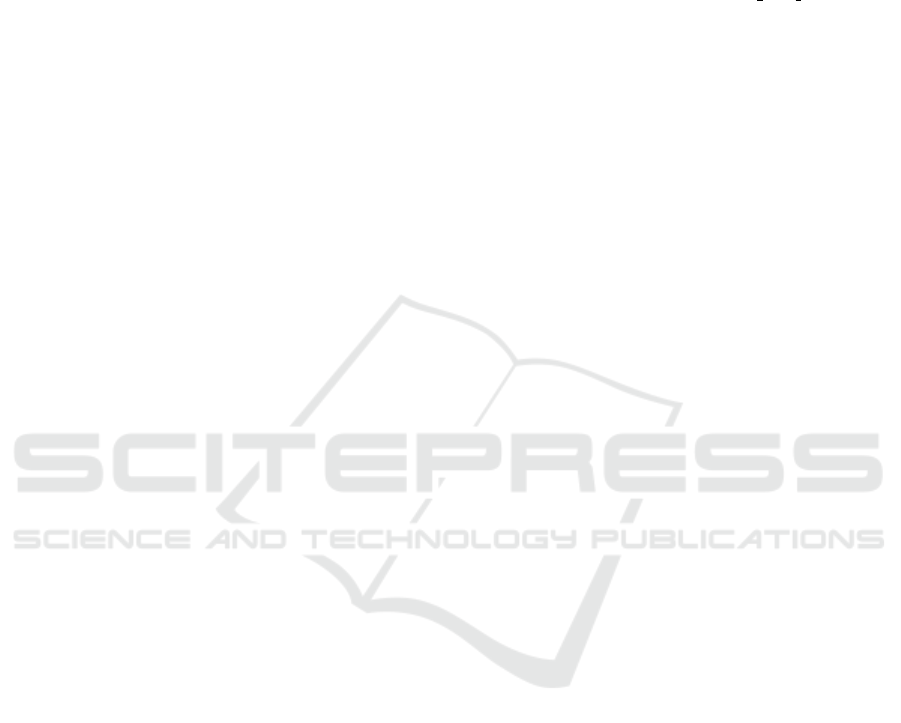
to generate web service request citation. Ghoshal and
Plale (Ghoshal and Plale, 2013) presented the most
relevant approach to ProvAnalyser. They explore the
options of deriving workflow provenance from exist-
ing log files. However, their focus is on collecting
provenance from different types of logs of distributed
applications. Our approach leverages Senaps event
log to capture interoperable provenance and analyse
it to understand and reproduce workflow outputs.
6 CONCLUSION
This work shows that provenance data can be captured
from scientific workflow systems’ event logs that can
verify the quality of their data products and allow the
analysis of workflows execution traces to make them
understandable and reusable. The logs can be filtered
and transformed into standardised provenance data
using a specialised model. This transformation allows
the recording of valuable information into a standard-
ised and workflow system-independent format that is
both interoperable and intelligible to the provenance
users. Also, the storage volumes of the provenance
required to perform data and workflow quality assess-
ments and analysis are smaller than the log size, indi-
cating the practical scalability of this transformation
process. While the workflow execution provenance
recorded from the event log can answer most of the
user queries, it is not always enough and, where it is
not, workflow prospective provenance can be inferred
and used. However, to enable comprehensive prove-
nance analytics, the systems should consider captur-
ing prospective and evolution provenance information
in their logs.
REFERENCES
Altintas, I., Barney, O., and Jaeger-Frank, E. (2006). Prove-
nance collection support in the kepler scientific work-
flow system. In Provenance and Annotation of Data,
pages 118–132, Berlin, Heidelberg. Springer.
Bavoil, L., Callahan, S. P., Crossno, P. J., Freire, J., Schei-
degger, C. E., Silva, C. T., and Vo, H. T. (2005). Vis-
trails: enabling interactive multiple-view visualiza-
tions. In VIS 05 IEEE Visualization, pages 135–142.
Belhajjame, K., Zhao, J., Garijo, D., Gamble, M., Hettne,
K., Palma, R., Mina, E., Corcho, O., G
´
omez-P
´
erez,
J. M., Bechhofer, S., et al. (2015). Using a suite of on-
tologies for preserving workflow-centric research ob-
jects. Journal of Web Semantics, 32:16–42.
Car, N. J., Stanford, L. S., and Sedgmen, A. (2016). En-
abling web service request citation by provenance in-
formation. In Provenance and Annotation of Data and
Processes - 6th International Provenance and Anno-
tation Workshop, McLean, VA, USA, June 7-8, 2016,
Proceedings, pages 122–133.
Car, N. J., Stenson, M. P., and Hartcher, M. (2014).
A provenance methodology and architecture
for scientific projects containing automated
and manual processes. [accessed through:
http://academicworks.cuny.edu/cc conf hic/57].
Cuevas-Vicentt
´
ın, V., Lud
¨
ascher, B., Missier, P., Belhaj-
jame, K., Chirigati, F., Wei, Y., Dey, S., Kianmajd,
P., Koop, D., Bowers, S., et al. (2016). Provone:
A prov extension data model for scientific workflow
provenance (2015). https://purl.dataone.org/provone-
v1-dev. [Online; accessed 12-Dec-2019].
Curcin, V. (2017). Embedding data provenance into the
learning health system to facilitate reproducible re-
search. Learning Health Systems, 1(2):e10019.
Fu, X., Ren, R., Zhan, J., Zhou, W., Jia, Z., and Lu, G.
(2012). Logmaster: Mining event correlations in logs
of large-scale cluster systems. In 2012 IEEE 31st Sym-
posium on Reliable Distributed Systems, pages 71–80.
Gaaloul, W., Gaaloul, K., Bhiri, S., Haller, A., and
Hauswirth, M. (2009). Log-based transactional work-
flow mining. Distributed and Parallel Databases,
25(3):193–240.
Garijo, D. and Gil, Y. (2011). A new approach for publish-
ing workflows: Abstractions, standards, and linked
data. In Proceedings of the 6th Workshop on Work-
flows in Support of Large-scale Science, WORKS ’11,
pages 47–56, New York, NY, USA. ACM.
Ghoshal, D. and Plale, B. (2013). Provenance from log
files: A bigdata problem. In Proceedings of the Joint
EDBT/ICDT 2013 Workshops, EDBT ’13, pages 290–
297, New York, NY, USA. ACM.
Goecks, J., Nekrutenko, A., and Taylor, J. (2010). Galaxy:
a comprehensive approach for supporting accessible,
reproducible, and transparent computational research
in the life sciences. Genome biology, 11(8):R86.
Gunter, D., Tierney, B., Crowley, B., Holding, M., and Lee,
J. (2000). Netlogger: A toolkit for distributed sys-
tem performance analysis. In Proceedings 8th Inter-
national Symposium on Modeling, Analysis and Sim-
ulation of Computer and Telecommunication Systems
(Cat. No. PR00728), pages 267–273. IEEE.
Herschel, M., Diestelk
`
amper, R., and Ben Lahmar, H.
(2017). A survey on provenance: What for? what
form? what from? The VLDB Journal-The Interna-
tional Journal on Very Large Data Bases, 26(6):881–
906.
Jiang, W., Hu, C., Pasupathy, S., Kanevsky, A., Li, Z., and
Zhou, Y. (2009). Understanding customer problem
troubleshooting from storage system logs. In Proc-
cedings of the 7th Conference on File and Storage
Technologies, FAST ’09, pages 43–56, Berkeley, CA,
USA. USENIX Association.
Kim, J., Deelman, E., Gil, Y., Mehta, G., and Ratnakar, V.
(2008). Provenance trails in the wings/pegasus sys-
tem. Concurrency and Computation: Practice and
Experience, 20(5):587–597.
Moreau and Missier (2013). World Wide Web Consortium
”PROV-DM: The PROV Data Model” W3C Recom-
MODELSWARD 2020 - 8th International Conference on Model-Driven Engineering and Software Development
114