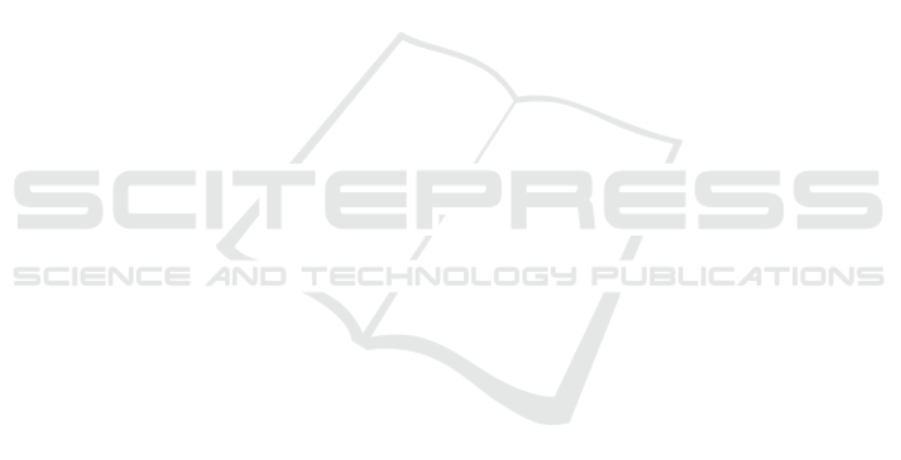
e.g. magnetic field, was compensated by modelling
the joints’ constraints in observation model and using
the body-IMU calibration results, i.e. joint axes and
positions. In order to monitor the strength exercises,
a personalized identification approach was proposed,
which doesn’t require a large labelled training dataset.
The idea is to use a template signal captured, where
users are instructed to perform the movements cor-
rectly according to their ability and health conditions.
Therefore, an online template matching algorithm is
optimized and applied to estimated position, which
led to improved accuracy and execution time. The ex-
perimental results of this validation showed relatively
good results, considering high intensity of the move-
ments. For further improvement in future , in order to
compensate for the intensive dynamic movements, an
outlier rejection approach can be implemented. Ad-
ditionally performance of EKF can be improved by
adaptive tuning of the noise covariances.
REFERENCES
Bleser, G., Steffen, D., Reiss, A., Weber, M., Hendeby, G.,
and Fradet, L. (2015). Personalized physical activity
monitoring using wearable sensors. In Smart health,
pages 99–124. Springer.
Chardonnens, J., Favre, J., Cuendet, F., Gremion, G., and
Aminian, K. (2013). A system to measure the kine-
matics during the entire ski jump sequence using iner-
tial sensors. Journal of biomechanics, 46(1):56–62.
Cheng, P. and Oelmann, B. (2010). Joint-angle measure-
ment using accelerometers and gyroscopes?a survey.
IEEE Transactions on instrumentation and measure-
ment, 59(2):404–414.
Dejnabadi, H., Jolles, B. M., Casanova, E., Fua, P., and
Aminian, K. (2006). Estimation and visualization of
sagittal kinematics of lower limbs orientation using
body-fixed sensors. IEEE Transactions on Biomedi-
cal Engineering, 53(7):1385–1393.
Fod, A., Matari
´
c, M. J., and Jenkins, O. C. (2002). Auto-
mated derivation of primitives for movement classifi-
cation. Autonomous robots, 12(1):39–54.
Harada, T., Mori, T., and Sato, T. (2007). Development of
a tiny orientation estimation device to operate under
motion and magnetic disturbance. The International
Journal of Robotics Research, 26(6):547–559.
Janus, B. and Nakamura, Y. (2005). Unsupervised proba-
bilistic segmentation of motion data for mimesis mod-
eling. In ICAR’05. Proceedings., 12th International
Conference on Advanced Robotics, 2005., pages 411–
417. IEEE.
Kok, M., Hol, J. D., and Sch
¨
on, T. B. (2014). An
optimization-based approach to human body motion
capture using inertial sensors. IFAC Proceedings Vol-
umes, 47(3):79–85.
Lin, J. F. and Kuli
´
c, D. (2012). Human pose recovery us-
ing wireless inertial measurement units. Physiological
measurement, 33(12):2099.
Lin, J. F.-S. and Kuli
´
c, D. (2013). Online segmentation of
human motion for automated rehabilitation exercise
analysis. IEEE Transactions on Neural Systems and
Rehabilitation Engineering, 22(1):168–180.
Luinge, H. J., Roetenberg, D., and Slycke, P. J. (2011).
Inertial sensor kinematic coupling. US Patent App.
12/534,526.
Morris, D., Saponas, T. S., Guillory, A., and Kelner, I.
(2014). Recofit: using a wearable sensor to find, rec-
ognize, and count repetitive exercises. In Proceedings
of the SIGCHI Conference on Human Factors in Com-
puting Systems, pages 3225–3234. ACM.
Optitrack (2019). Optitrack flex 13 (accessed 9/17/2014).
Reiss, A., Hendeby, G., Bleser, G., and Stricker, D.
(2010). Activity recognition using biomechanical
model based pose estimation. In 5th European Con-
ference on Smart Sensing and Context (EuroSSC),
pages 42–55.
Sakurai, Y., Faloutsos, C., and Yamamuro, M. (2007).
Stream monitoring under the time warping distance.
In 2007 IEEE 23rd International Conference on Data
Engineering, pages 1046–1055.
Salehi, S., Bleser, G., Reiss, A., and Stricker, D. (2015).
Body-imu autocalibration for inertial hip and knee
joint tracking. In Proceedings of the 10th EAI Interna-
tional Conference on Body Area Networks, BodyNets
’15, pages 51–57, ICST, Brussels, Belgium, Bel-
gium. ICST (Institute for Computer Sciences, Social-
Informatics and Telecommunications Engineering).
Salehi, S., Bleser, G., and Stricker, D. (2014). Design and
development of low-cost smart training pants (stants).
In 4th International Conference on Wireless Mobile
Communication and Healthcare, At Athen, Greece,
pages 39–44. IEEE.
Seel, T., Raisch, J., and Schauer, T. (2014). Imu-based
joint angle measurement for gait analysis. Sensors,
14(4):6891–6909.
Um, T. T., Babakeshizadeh, V., and Kuli
´
c, D. (2017). Ex-
ercise motion classification from large-scale wearable
sensor data using convolutional neural networks. In
2017 IEEE/RSJ International Conference on Intelli-
gent Robots and Systems (IROS), pages 2385–2390.
IEEE.
Yan, X., Li, H., Li, A. R., and Zhang, H. (2017). Wear-
able imu-based real-time motion warning system for
construction workers’ musculoskeletal disorders pre-
vention. Automation in Construction, 74:2–11.
Zhu, R. and Zhou, Z. (2004). A real-time articulated human
motion tracking using tri-axis inertial/magnetic sen-
sors package. IEEE Transactions on Neural systems
and rehabilitation engineering, 12(2):295–302.
Zinnen, A., Blanke, U., and Schiele, B. (2009). An analysis
of sensor-oriented vs. model-based activity recogni-
tion. In 2009 International Symposium on Wearable
Computers, pages 93–100. IEEE.
SENSORNETS 2020 - 9th International Conference on Sensor Networks
104