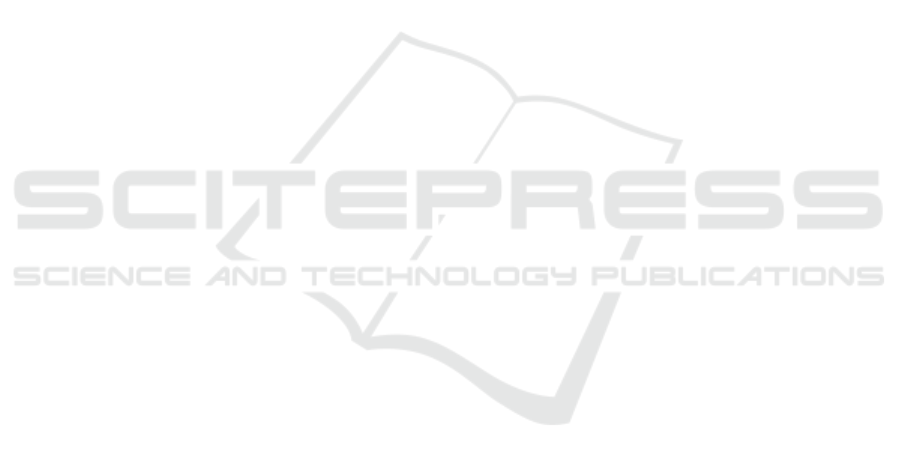
Harry Pratt, Frans Coenen, D. M. B. S. P. H. Y. Z. (2016).
Convolutional neural networks for diabetic retinopa-
thy.
He, K., Zhang, X., Ren, S., and Sun, J. (2015). Delving deep
into rectifiers: Surpassing human-level performance
on imagenet classification. CoRR, abs/1502.01852.
Hu, J., Shen, L., and Sun, G. (2017). Squeeze-and-
excitation networks. CoRR, abs/1709.01507.
Iglovikov, V. and Shvets, A. (2018). Ternausnet: U-net with
VGG11 encoder pre-trained on imagenet for image
segmentation. CoRR, abs/1801.05746.
Kolesnikov, S. (2018). Reproducible and fast dl and rl.
Krause, J., Gulshan, V., Rahimy, E., Karth, P., Widner, K.,
Corrado, G. S., Peng, L., and Webster, D. R. (2017).
Grader variability and the importance of reference
standards for evaluating machine learning models for
diabetic retinopathy. CoRR, abs/1710.01711.
Krogh, A. and Hertz, J. A. (1992). A simple weight decay
can improve generalization. In Moody, J. E., Hanson,
S. J., and Lippmann, R. P., editors, Advances in Neu-
ral Information Processing Systems 4, pages 950–957.
Morgan-Kaufmann.
Lin, T., Goyal, P., Girshick, R. B., He, K., and Doll
´
ar, P.
(2017). Focal loss for dense object detection. CoRR,
abs/1708.02002.
Liyuan Liu, Haoming Jiang, P. H. W. C. X. L. J. G. J. H.
(2019). On the variance of the adaptive learning rate
and beyond. CoRR, abs/1908.03265.
Loshchilov, I. and Hutter, F. (2016). SGDR: stochastic gra-
dient descent with restarts. CoRR, abs/1608.03983.
Lundberg, S. M. and Lee, S.-I. (2017). A unified ap-
proach to interpreting model predictions. In Guyon, I.,
Luxburg, U. V., Bengio, S., Wallach, H., Fergus, R.,
Vishwanathan, S., and Garnett, R., editors, Advances
in Neural Information Processing Systems 30, pages
4765–4774. Curran Associates, Inc.
Michael D. Abrmoff, Joseph M. Reinhardt, S. R. R. J. C. F.
V. B. M. M. N. and Quellec, G. (2010). Automated
early detection of diabetic retinopathy.
Nathan Silberman, Kristy Ahlrich, R. F. and Subramanian,
L. (2010). Case for automated detection of diabetic
retinopathy.
NCBI (2018). The economic impact of sight loss and blind-
ness in the uk adult population.
NCHS (2019). Eye disorders and vision loss among u.s.
adults aged 45 and over with diagnosed diabetes.
Nichol, A., Achiam, J., and Schulman, J. (2018).
On first-order meta-learning algorithms. CoRR,
abs/1803.02999.
Paszke, A., Gross, S., Chintala, S., Chanan, G., Yang, E.,
DeVito, Z., Lin, Z., Desmaison, A., Antiga, L., and
Lerer, A. (2017). Automatic differentiation in Py-
Torch. In NIPS Autodiff Workshop.
Priya, R. and Aruna, P. (2012). Svm and neural network
based diagnosis of diabetic retinopathy.
Rohan T, Frost C, W. N. (1989). Prevention of blindness
by screening for diabetic retinopathy: a quantitative
assessment.
Rubina Sarki, Sandra Michalska, K. A. H. W. Y. Z.
(2019). Convolutional neural networks for mild di-
abetic retinopathy detection: an experimental study.
bioRxiv.
Sahasrabuddhe, P. P. S. P. R. K. M. K. G. D. V. and Meri-
audeau, F. (2018). Indian diabetic retinopathy image
dataset (idrid).
Shaohua Wan, Yan Liang, Y. Z. (2018). Deep convolutional
neural networks for diabetic retinopathy detection by
image classification.
SNEC (2019). Singapore’s eye health.
Szegedy, C., Vanhoucke, V., Ioffe, S., Shlens, J., and Wojna,
Z. (2016). Rethinking the inception architecture for
computer vision. In Proceedings of IEEE Conference
on Computer Vision and Pattern Recognition,.
Tan, M. and Le, Q. V. (2019). Efficientnet: Rethink-
ing model scaling for convolutional neural networks.
cite arxiv:1905.11946Comment: Published in ICML
2019.
van der Maaten, L. and Hinton, G. (2008). Visualizing data
using t-SNE. Journal of Machine Learning Research,
9:2579–2605.
Yung-Hui Li, Nai-Ning Yeh, S.-J. C. and Chung, Y.-C.
(2019). Computer-assisted diagnosis for diabetic
retinopathy based on fundus images using deep con-
volutional neural network.
Deep Learning Approach to Diabetic Retinopathy Detection
509