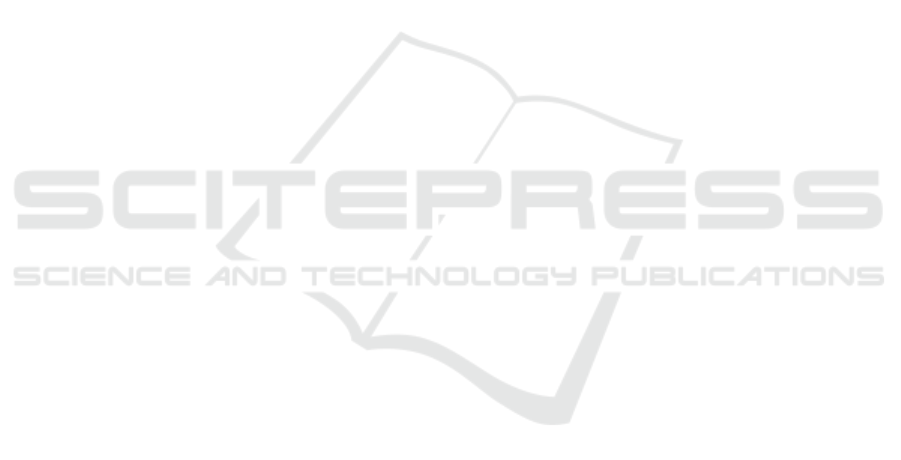
6 CONCLUSIONS
In this work, a novel strategy based in SAX is
proposed to improve the representation of cattle
information gathering through WSNs. As the study
has been promoted within the Digitanimal project,
different insights and requirements from the company
has been considered to define the solution.
The proposed approach is based on the SAX
representation technique. Different combinations
for the parameters of the SAX representation have
been evaluated, and compared with the current
company solution, through a common procedure for
the estimation of the error. Major improvements have
been achieved.
Besides, the present study is the first step towards
the development of higher quality services for the
company. A better accuracy in the representation
of animal behavior could improve real-time problem
detection such as animal calvings or heats.
Next steps and future work will imply different
tasks related to the validation of these results, using
more animals and more days, and development of
new possible strategies. In order to verify the
results achieved in this work, devices programmed
with the proposed solution will be used in future
studies. This task should be done in collaboration
with the company and experimental farms that
allow the new stage of information gathering. On
the other hand, devise of new strategies can be
done expanding the study by introducing different
amount of bits per axis and variables, by using
different representation techniques or even by using
alternative levels definitions. In this way, the Trend
Segmentation Algorithm (Siordia et al., 2011), the
Trend Feature Symbolic Aggregate approXimation
(Yu et al., 2019) or the Fast Low-cost Online
Semantic Segmentation (Gharghabi et al., 2019)
could be considered.
ACKNOWLEDGEMENTS
Research supported by grants from Madrid
Autonomous Community (Ref: IND2018/TIC-
9665) and European Union’s H2020 Research and
Innovation Program, through the IoF2020 project
(H2020-IoT-2016) under subgrant agreement no.
2282300206-UC010. Special thanks to MISC
International S.L.
REFERENCES
Akyildiz, I. F., Su, W., Sankarasubramaniam, Y., and
Cayirci, E. (2002). Wireless sensor networks: a
survey. Computer networks, 38(4):393–422.
Berndt, D. J. and Clifford, J. (1994). Using dynamic time
warping to find patterns in time series. In KDD
workshop, volume 10, pages 359–370. Seattle, WA.
Brezmes, T., Gorricho, J.-L., and Cotrina, J. (2009).
Activity recognition from accelerometer data on a
mobile phone. In International Work-Conference on
Artificial Neural Networks, pages 796–799. Springer.
CattleChain (2019). Cattlechain, 2019. https://www.
cattlechain.eu/.
Chan, K.-P. and Fu, A. W.-C. (1999). Efficient time
series matching by wavelets. In Proceedings 15th
International Conference on Data Engineering (Cat.
No. 99CB36337), pages 126–133. IEEE.
Chen, L. and Ng, R. (2004). On the marriage of lp-norms
and edit distance. In Proceedings of the Thirtieth
international conference on Very large data bases-
Volume 30, pages 792–803. VLDB Endowment.
Digitanimal (2019). Digitanimal, 2019. https://www.
digitanimal.com/.
Diosdado, J. A. V., Barker, Z. E., Hodges, H. R.,
Amory, J. R., Croft, D. P., Bell, N. J., and Codling,
E. A. (2015). Classification of behaviour in housed
dairy cows using an accelerometer-based activity
monitoring system. Animal Biotelemetry, 3(1):15.
Duarte-Melo, E. J. and Liu, M. (2002). Analysis of energy
consumption and lifetime of heterogeneous wireless
sensor networks. In Global Telecommunications
Conference, 2002. GLOBECOM’02. IEEE, volume 1,
pages 21–25. IEEE.
Faloutsos, C., Ranganathan, M., and Manolopoulos, Y.
(1994). Fast subsequence matching in time-series
databases, volume 23. ACM.
Gharghabi, S., Yeh, C.-C. M., Ding, Y., Ding, W., Hibbing,
P., LaMunion, S., Kaplan, A., Crouter, S. E., and
Keogh, E. (2019). Domain agnostic online semantic
segmentation for multi-dimensional time series. Data
mining and knowledge discovery, 33(1):96–130.
Keogh, E., Chakrabarti, K., Pazzani, M., and Mehrotra, S.
(2001). Dimensionality reduction for fast similarity
search in large time series databases. Knowledge and
information Systems, 3(3):263–286.
Korn, F., Jagadish, H. V., and Faloutsos, C. (1997).
Efficiently supporting ad hoc queries in large datasets
of time sequences. In Acm Sigmod Record, volume 26,
pages 289–300. ACM.
Larsen, R. J., Marx, M. L., et al. (1986). An introduction to
mathematical statistics and its applications, volume 2.
Prentice-Hall Englewood Cliffs, NJ.
Lin, J., Keogh, E., Lonardi, S., and Chiu, B. (2003).
A symbolic representation of time series, with
implications for streaming algorithms. In Proceedings
of the 8th ACM SIGMOD workshop on Research
issues in data mining and knowledge discovery, pages
2–11. ACM.
New Commercial Representation for Cattle Information Gathering
533