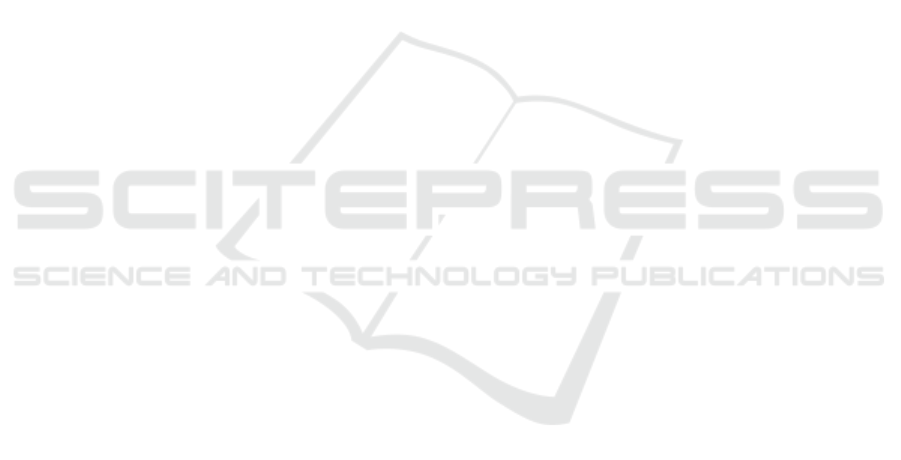
number of deferrals for each clinic varies widely. The
number of estimated deferrals will not be considered
in the developed regression model.
1.1 Literature Review
In (Muthuraman and Lawley, 2008), a stochastic op-
timization model is developed where patient service
times are exponentially distributed and individual pa-
tients categorized by similar attributes share a com-
mon no-show probability. The work is theoretical in
nature and, as such, the authors do not posit on what
attributes might be used to cluster patients and how a
no-show probability might be developed for that clus-
ter. The objective function of the optimization model
is to maximize profit and consequently, the model is
applicable only to environments having a profit mo-
tive. In (Li et al., 2019), the optimization model of
(Muthuraman and Lawley, 2008) is leveraged with a
no-show probability unique to each individual patient
based on a variety of socio-demographic and con-
textual parameters. Most notably, a patient’s histor-
ical propensity to not show up for an appointment is
a strong indicator of future no-shows. Monte Carlo
simulation of empirical patient records suggest the
combination of these models is effective in maximiz-
ing clinic profit. The motivations of Canadians in do-
nating blood is specifically explored by Smith et al.
((Smith et al., 2011)). Through extensive donor inter-
views, their research suggests that none of the above
socio-demographic variables are influential to an indi-
vidual’s propensity to donate blood. Nor do Canadian
blood donors subscribe to individual altruism. Rather,
they are motivated by aspirations to fit within norma-
tive behaviour defined by social or workplace groups.
It’s common for sports teams, clubs, or workplaces to
organize and commit to blood donation events. In this
context, it is neither altruism nor socio-demographic
variables that are the most valuable of predictors but
what social groups the individual belongs to.
A utility function first proposed in (LaGanga and
Lawrence, 2007) was later applied to the overbooking
policy of a medical clinic in (LaGanga and Lawrence,
2012). This utility function consider the time patients
spend waiting, overtime of medical staff, and conse-
quently represents a tradeoff between the costs asso-
ciated with overbooking (wait and overtime) versus
no-shows (resource idleness). In this case, a common
no-show probability was applied uniformly across all
patients. As in (Muthuraman and Lawley, 2008), ap-
pointment slots are also of consistent length. These
same costs are included in the model presented in
(Chen et al., 2018) but, here, the authors adopt flexible
appointment start times in lieu of fixed appointment
slots. Zacharias and Pinedo (2014) present a similar
model but includes weights for patients representing
differing costs by patient. In (Kros et al., 2009), an
additional cost is included in the utility function - that
is the cost of burnout among service providers result-
ing from sustained overbooking. Liu et al. (N. and
Ziya, 2014) consider policies meant to encourage pa-
tient attendance (e.g., reminder phone calls) and their
associated costs as part of the objective function.
A game theoretic approach is adopted in (Zeng
et al., 2009) and (Zeng et al., 2013) where the prob-
ability of the patient not showing up for the appoint-
ment is a function of the overbooking strategy itself.
As the clinic overbooks more aggressively, the in-
creased patient waiting time dissuades patients from
showing up to the appointment. Zeng et al. (2010)
also demonstrate that when the traditional problem
formulation includes homogeneous patients having a
common no-show probability, the objective function
(to minimize costs - or maximize profit) is convex.
For heterogenous patients, the authors propose a local
neighbourhood search solution strategy.
In both (Huang and Zuniga, 2012) and (Huang
and Hanauer, 2014) the no-show probability is con-
sidered dynamic. Various scenarios are simulated and
ANOVA (in conjunction with the Tukey post-hoc test)
to identify strategies having significantly better re-
sults. It also considers patients to be homogeneous
in this regard. The focus of (Huang and Hanauer,
2014) was to predict the patient’s probability of no-
show as a function of a variety of socio-demographic
and contextual parameters, as in (Li et al., 2019). Un-
like in (Li et al., 2019), the authors of (Huang and
Hanauer, 2014) translated this probability to a binary
show/no-show variable by minimizing the error rate
(rather than the system’s total cost).
Other notable works include a discrete event sim-
ulation is leveraged by (Fan et al., 2016) to establish
the optimal length of schedule slots and the optimal
number of patients to schedule in those slots. Both
booked appointments and walk-in patients are consid-
ered in (Kim and Giachetti, 2006) where the paper’s
aim is to develop the stochastic functions leading to a
mean patient no-show probability. Despite the prolific
use of overbooking strategies within the tourism in-
dustry, (Riasi et al., 2019) noted that few hotels have
adopted the theoretically superior risk-based models
similar to those described above. Instead, a determin-
istic approach is chosen based on the ratio of hotel
capacity to historical show rates.
Notwithstanding the prevalence of these prob-
lems throughout the extent literature, most authors
have focused on largely theoretical applications hav-
ing untested utility or objective functions. The long-
Regression Analysis of Historical Blood Donors to Improve Clinic Scheduling
345