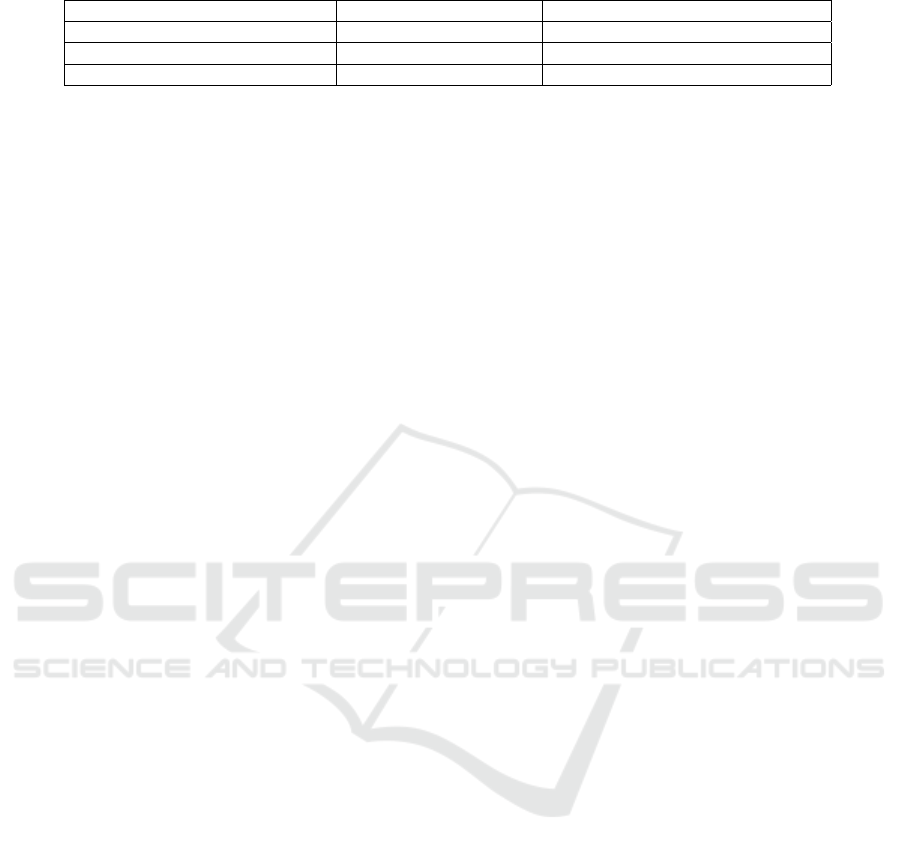
Table 5: Comparison of results.
Approach Result
Proposed Method (SPM+RandomForest) Subject 1: 99.38%Subject 2: 95.45%
(Raeiszadeh and Tahayori, 2018) (UP-DM+RandomForest) Subject 1: 97.45%Subject 2: 91.37%
(Tapia et al., 2004) (Naive Bayes Classifier) Subject 1: 60.6% Subject 2: 41.09%
Agrawal, R. and Srikant, R. (1995). Mining sequential pat-
terns. In The International Conference on Data Engi-
neering, pp. 3-14.
Agrawal, R. and Srikant, R. (1996). Mining sequential
patterns: Generalizations and performance improve-
ments. In The International Conference on Extending
Database Technology, pp. 1-17.
Atev, S., Masoud, O., and Papanikolopoulos, N. (2006).
Learning traffic patterns at intersections by spectral
clustering of motion trajectories. In Conf. Intell.
Robots and Systems, Bejing, China, pp. 4851–4856.
IEEE.
Ayres, J., Flannick, J., Gehrke, J., and Yiu, T. (2002). Se-
quential pattern mining using a bitmap representation.
In International Conference on Knowledge Discovery
and Data Mining, pp. 429-435. ACM SIGKDD.
Fournier-Viger, P., Gomariz, A., Campos, M., and Thomas,
R. (2014). Fast vertical mining of sequential patterns
using co-occurrence information. In The Pacic-Asia
Conference on Knowledge Discovery and Data Min-
ing, pp. 40-52.
Hassani, M., Beecks, C., ows, D. T., and Seidl, T. (2015).
Mining sequential patterns of event streams in a smart
home application. In The LWA 2015 Workshops:
KDML, FGWM, IR, and FGD.
Li, L., Li, X., Lu, Z., Lloret, J., and Song, H. (2017).
Sequential behavior pattern discovery with frequent
episode mining and wireless sensor network. In Com-
munications Magazine. IEEE.
Makris, D. and Ellis, T. (2005). Learning semantic scene
models from observing activity in visual surveillance.
In Trans. Syst., Man, Cybern. B, vol. 35, no. 3, pp.
397–408. IEEE.
Menaka, J. and Gayathri, K. S. (2013). Activity modeling
in smart home using high utility pattern mining over
data streams. In The Journal of Computer Science and
Network.
Morris, B. T. and Trivedi, M. M. (2008). Learning, mod-
eling, and classification of vehicle track patterns from
live video. In Trans. Intell. Transp. Syst., vol. 9, no. 3,
pp. 425–437. IEEE.
Moutacalli, M. T., Bouzouane, A., and Bouchard, B.
(2012). Unsupervised activity recognition using tem-
poral data mining. In The First International Confer-
ence on Smart Systems, Devices and Technologies.
Piciarelli, C. and Foresti, G. L. (2006). On-line trajectory
clustering for anomalous events detection. In Pattern
Recognition Letters, vol. 27, no. 15, pp. 1835–1842.
Raeiszadeh, M. and Tahayori, H. (2018). A novel method
for detecting and predicting resident’s behavior in
smart home. In 6th Iranian Joint Congress on Fuzzy
and Intelligent Systems. IEEE.
Schweizer, D., Zehnder, M., Wache, H., and Witschel, H.
(2015). Using consumer behavior data to reduce en-
ergy consumption in smart homes. In 14th Interna-
tional Conference on Machine Learning and Applica-
tions.
Singh, S. and Yassine, A. (2017). Mining energy consump-
tion behavior patterns for house holds in smart grid.
In Transactions on Emerging Topics in Computing.
IEEE.
Stauffer, C. and Grimson, W. E. L. (2000). Learn-
ing patterns of activity using real-time tracking. In
Trans. Pattern Anal. Mach. Intell., vol. 22, no. 8, pp.
747–757. IEEE.
Suryadevara, N. (2017). Wireless sensor sequence data
model for smart home and iot data analytics. In
First International Conferenceon Computational In-
telligence and Informatics, Advances in Intelligent
Systems and Computing.
Tapia, E. M., Intille, S. S., and Larson, K. (2004). Activ-
ity recognition in the home setting using simple and
ubiquitous sensors. In Pervasive Computing.
Zaki, M. J. (2000). Scalable algorithms for association min-
ing. In Transactions on Knowledge and Data Engi-
neering, vol. 12(3), pp. 372-390. IEEE.
Zaki, M. J. (2001). Spade: An effcient algorithm for mining
frequent sequences. In Machine learning, vol. 42(1-
2), pp. 31-60.
Activity Mining in a Smart Home from Sequential and Temporal Databases
547