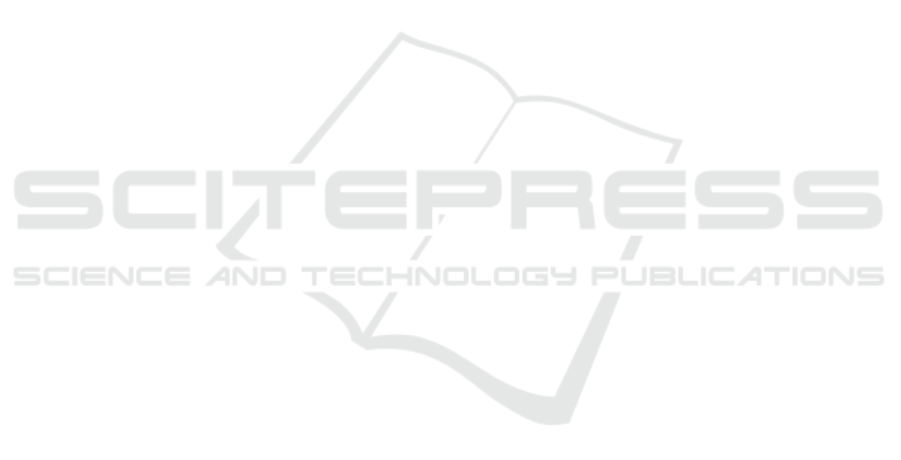
5 CONCLUSIONS
In this paper we review various classifiers that use
both hand-crafted features and deep learning tech-
niques for classifying images as pornographic/benign.
However, no known deep learning approaches were
found to label sexual organs inside these images.
Hence, we propose a two-step approach, which uses
a first classifier to classify an image as pornographic
or benign, then tessellates pornographic images into
windows. A second classifier checks these windows
for sexual organs and augments the initial classifica-
tion of the pornographic image by describing the ex-
plicit content in that image.
Our results show that the MobileNet architecture
has similar classification performance as the other
networks tested but reduces the computation time by
at least 20%. Thus, it was considered suitable for
our system and achieved an accuracy of 95% in the
first step of our pipeline. This result implies that the
pornographic image classifier correctly distinguishes
between benign and pornographic images.
The second step of our pipeline reached an accu-
racy of 72.58% for windows centred around the pri-
vate body parts. This demonstrates that deep learn-
ing approaches can be used to detect and label private
body parts in pornographic images. When evaluating
the full pipeline, an accuracy of 62% was achieved.
While this is larger than a pure chance classifier, we
note that this classifier can be further improved by
having more training data and introducing smarter
window selection schemes. We propose that window
selection can be achieved by extracting a feature map
from the first pornography classifier and using this
map to localise regions of interest presumably con-
taining private body parts.
ACKNOWLEDGEMENTS
This research has been funded with support from the
European Commission under the 4NSEEK project
with Grant Agreement 821966. This publication re-
flects the views only of the authors, and the Euro-
pean Commission cannot be held responsible for any
use which may be made of the information contained
therein.
REFERENCES
Bhoyar (2010). Skin color detection model using neural
networks and its performance evaluation. Journal of
Computer Science, 6(9):963–968.
Choi, B., Han, S., Chung, B., and Ryou, J. (2011). Human
body parts candidate segmentation using Laws texture
energy measures with skin color. In 13th International
Conference on Advanced Communication Technology
(ICACT2011), pages 556–560.
Forsyth, D. A. and Fleck, M. M. (1999). Automatic detec-
tion of human nudes. International Journal of Com-
puter Vision, 32(1):63–77.
Fuangkhon, P. and Tanprasert, T. (2005). Nipple detection
for obscene pictures. In Proceedings of the 5th WSEAS
International Conference on Signal, Speech and Im-
age Processing, SSIP’05, pages 242–247.
Hajraoui, A. and Sabri, M. (2014). Face detection algo-
rithm based on skin detection, watershed method and
gabor filters. International Journal of Computer Ap-
plications, 94:33–39.
Howard, A. G., Zhu, M., Chen, B., Kalenichenko, D.,
Wang, W., Weyand, T., Andreetto, M., and Adam,
H. (2017). Mobilenets: Efficient convolutional neu-
ral networks for mobile vision applications.
Hu, W., Wu, O., Chen, Z., Fu, Z., and Maybank, S. (2007).
Recognition of pornographic web pages by classify-
ing texts and images. IEEE Transactions on Pattern
Analysis and Machine Intelligence, 29:1019–1034.
Huang, L. and Ren, X. (2018). Erotic image recogni-
tion method of bagging integrated convolutional neu-
ral network. In Proceedings of the 2nd International
Conference on Computer Science and Application En-
gineering, CSAE ’18, pages 107:1–107:7, New York,
NY, USA. ACM.
Huang, Y. and Kong, A. W. K. (2016). Using a CNN ensem-
ble for detecting pornographic and upskirt images. In
2016 IEEE 8th International Conference on Biomet-
rics Theory, Applications and Systems (BTAS), pages
1–7.
Jin, X., Wang, Y., and Tan, X. (2018). Pornographic image
recognition via weighted multiple instance learning.
IEEE Transactions on Cybernetics, pages 1–9.
Kejun, X., Jian, W., Pengyu, N., and Jie, H. (2012). Auto-
matic nipple detection using cascaded AdaBoost clas-
sifier. In 2012 Fifth International Symposium on Com-
putational Intelligence and Design, volume 2, pages
427–432.
Kim, Y., Hwang, I., and Cho, N. I. (2017). Convolutional
neural networks and training strategies for skin detec-
tion. In 2017 IEEE International Conference on Im-
age Processing (ICIP), pages 3919–3923.
Lienhart, R. and Maydt, J. (2002). An extended set of haar-
like features for rapid object detection. In Proceed-
ings. International Conference on Image Processing,
volume 1, pages I–I. IEEE.
Lv, L., Zhao, C., Lv, H., Shang, J., Yang, Y., and Wang,
J. (2011). Pornographic images detection using high-
level semantic features. In 2011 Seventh International
Conference on Natural Computation, volume 2, pages
1015–1018.
Macedo, J., Costa, F., and dos Santos, J. A. (2018a). A
benchmark methodology for child pornography detec-
tion. In 2018 31st SIBGRAPI Conference on Graph-
ics, Patterns and Images (SIBGRAPI). IEEE.
ICPRAM 2020 - 9th International Conference on Pattern Recognition Applications and Methods
210