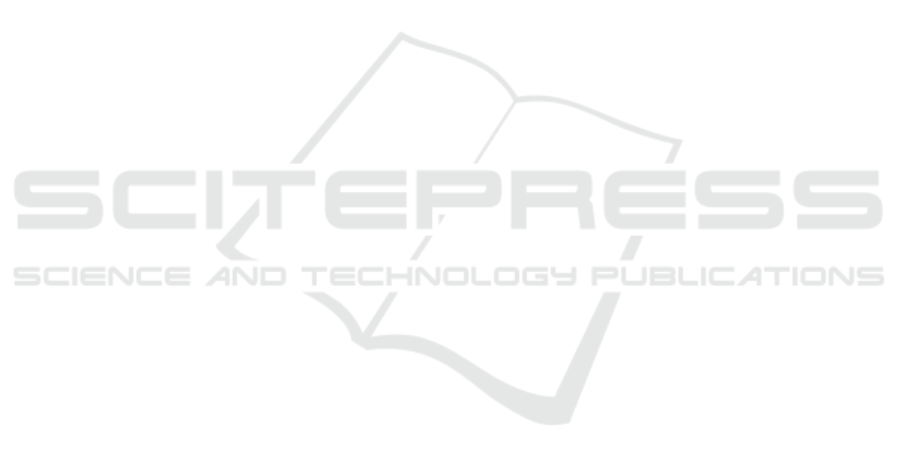
5.3 Comparison of Average
Performance of OSAOC versus
Omniscient Control
Ten samples each of Poisson and uniformly dis-
tributed demands were generated and the average ef-
ficiency (resp. standard deviation) were found to be
78.25%(3.28%) (Poisson) and 73.52%(5.77%) (uni-
form).
The coefficient of variation is a measure of relative
variability, defined as the ratio of the standard devia-
tion to the mean of the variable under consideration.
Thus, a measure of amplification of demand variabil-
ity, also known as bullwhip, is the ratio of the coef-
ficients of variation of the ordering and the demand,
and is denoted B
x
od
, where the subscript od refers to
the ratio being calculated and the superscript x can be
os (resp. om) for one step (resp.omniscient). A related
measure is the ratio of the coefficients of variation of
the inventory (store) level and the demand, denoted
B
x
std
. For the Poisson demand of sec. 5.2, the calcu-
lations, carried out over the interval [D,K
f
− D] so as
to exclude both initial transient due to pipeline ini-
tialization, and final transient due to the turnpike ef-
fect for the omniscient case, yield: B
os
od
= 0.43,B
os
std
=
0.32,B
om
od
= 0.70,B
om
std
= 0.28. Since all these mea-
sures are less than unity, the conclusion is that the
proposed one step ahead controller does not cause
amplification of the demand uncertainty. This should
be contrasted with the behavior of controllers of the
IOBPCS family (Lin and Naim, 2019) and the MPC
family with targets (Subramanian et al., 2014).
6 CONCLUDING REMARKS
This paper proposed a one step ahead optimal control
scheme for a single echelon supply chain that does
not require prediction but only the solution of a low-
dimensional (in terms of the number of decision vari-
ables) optimization problem at each time step, thus
being perfectly adequate for real time supply chain
control. This one step ahead or greedy control is
shown to be quite efficient, attaining, for Poisson or
uniformly distributed demands, at least 70% of the
(unattainable) omniscient global optimum. In addi-
tion, experiments show that the proposed OSAO con-
trol scheme does not cause amplification of the de-
mand uncertainty or bullwhip, which is attributable to
the fact that no target levels for inventory or pipeline
are used. Another important observation is that the
model (1)-(12), with the exception of (5), consists
of linear equations. It is easy to write (5) in piece-
wise linear form, which means that both the omni-
scient (13) and OSAOC optimization problems (18)
can be rewritten as linear programming problems,
which, from a computational viewpoint, implies that
the simple examples in Sec. 5, can easily be scaled to
much larger dimension. It should also be noted that a
large number of supply chain problems, including the
multi-echelon case, are described by linear or piece-
wise linear dynamics. Thus, the proposed scheme is
scalable and can: (i) be generalized to large multi-
echelon supply chains, which will be the subject of fu-
ture work, and (ii) used in a planning mode, to choose
inventory size, shipping capacity requirements and
prices that ensure profit margins, since these choices
can be recast as feasibility problems. This is also the
subject of ongoing work, to be reported in a future
version of this paper.
REFERENCES
Hoberg, K., Bradley, J. R., and Thonemann, U. W. (2007).
Analyzing the effect of the inventory policy on or-
der and inventory variability with linear control the-
ory. European Journal of Operational Research,
176:1620–1642.
Ivanov, D., Sethi, S., Dolgui, A., and Sokolov, B. (2018).
A survey on control theory applications to operational
systems, supply chain management, and industry 4.0.
Annual Reviews in Control.
Lavretsky, E. (2000). Greedy optimal control. In Amer-
ican Control Conference (ACC), pages 3888–3892,
Chicago,IL.
Lin, J. and Naim, M. (2019). Why do nonlinearities
matter? the repercussions of linear assumptions
on the dynamic behaviour of assemble-to-order sys-
tems. International Journal of Production Research,
57(20):6424–6451.
Lin, J., Naim, M., Purvis, L., and Gosling, J. (2017). The
extension and exploitation of the inventory and order
based production control system archetype from 1982
to 2015. International Journal of Production Eco-
nomics, 194:135–152.
Lin, J., Naim, M., and Spiegler, V. (2019). Delivery time
dynamics in an assemble-to-order inventory and order
based production control system. International Jour-
nal of Production Economics.
McGarvey, B. and Hannon, B. (2004). Dynamic Modeling
for Business Management: An Introduction. Springer,
New York.
Subramanian, K., Rawlings, J. B., and Maravelias, C. T.
(2014). Economic model predictive control for inven-
tory management in supply chains. Computers and
Chemical Engineering, 64:71–80.
Wang, W.-L., Rivera, D. E., and Kempf, K. G. (2007).
Model predictive control strategies for supply chain
management in semiconductor manufacturing. Int. J.
Production Economics, 107:56–77.
One Step Ahead Optimal Control of a Single Echelon Supply Chain using Mathematical Programming
237