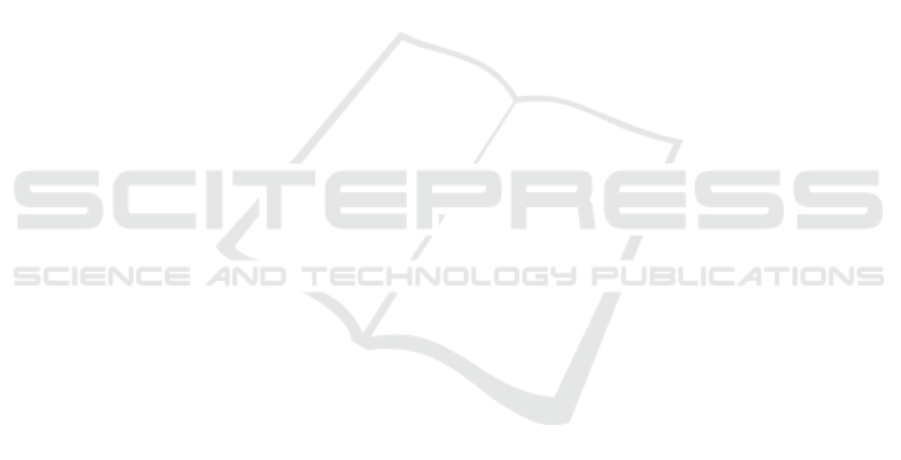
one under analysis.
Additionally, for LSTM and CNN architectures
two different concatenation schemes and a pre-
training process was also implemented. Overall, 15
models were tested and the results indicated promis-
ing architecture schemes for price prediction, as well
as the importance of developing more complex archi-
tectures when dealing with such a volatile informa-
tion. The one-dimensional CNN showed the best re-
sults among all models and, therefore, it is the recom-
mended architecture for further research within this
task.
Suggestions for future work include:
• Testing and validating the results of the best per-
forming models with larger data sets and with
more inputs related to the price dynamic of the
Nordic market.
• Adding information of neighboring internal bid-
ding areas to assess the transmission bottlenecks
present around Finland.
• Considering external markets that are directly
connected to the examined country in order to
improve the predictive accuracy of the proposed
models.
ACKNOWLEDGEMENTS
The research was supported by the Hungarian Gov-
ernment and co-financed by the European Social
Fund through the project ”Talent management in
autonomous vehicle control technologies” (EFOP-
3.6.3-VEKOP-16-2017-00001).
REFERENCES
Bai, S., Kolter, J. Z., and Koltun, V. (2018). An em-
pirical evaluation of generic convolutional and recur-
rent networks for sequence modeling. arXiv preprint
arXiv:1803.01271.
Bunn, D. W. and Gianfreda, A. (2010). Integration and
shock transmissions across european electricity for-
ward markets. Energy Economics, 32(2):278–291.
Catal
˜
ao, J. P. d. S., Mariano, S. J. P. S., Mendes, V.,
and Ferreira, L. (2007). Short-term electricity prices
forecasting in a competitive market: A neural net-
work approach. Electric Power Systems Research,
77(10):1297–1304.
de Menezes, L. M. and Houllier, M. A. (2016). Reassess-
ing the integration of european electricity markets: A
fractional cointegration analysis. Energy Economics,
53:132–150.
Jamasb, T. and Pollitt, M. (2005). Electricity market reform
in the european union: review of progress toward lib-
eralization & integration. The Energy Journal, pages
11–41.
Kohavi, R. et al. (1995). A study of cross-validation and
bootstrap for accuracy estimation and model selec-
tion. In Ijcai, volume 14, pages 1137–1145. Montreal,
Canada.
Kong, W., Dong, Z. Y., Jia, Y., Hill, D. J., Xu, Y., and
Zhang, Y. (2017). Short-term residential load fore-
casting based on lstm recurrent neural network. IEEE
Transactions on Smart Grid, 10(1):841–851.
Lago, J., De Ridder, F., Vrancx, P., and De Schutter, B.
(2018). Forecasting day-ahead electricity prices in eu-
rope: the importance of considering market integra-
tion. Applied Energy, 211:890–903.
Lu, Y. and Salem, F. M. (2017). Simplified gating in long
short-term memory (lstm) recurrent neural networks.
In 2017 IEEE 60th International Midwest Symposium
on Circuits and Systems (MWSCAS), pages 1601–
1604. IEEE.
Panapakidis, I. P. and Dagoumas, A. S. (2016). Day-ahead
electricity price forecasting via the application of arti-
ficial neural network based models. Applied Energy,
172:132–151.
Peng, L., Liu, S., Liu, R., and Wang, L. (2018). Effec-
tive long short-term memory with differential evolu-
tion algorithm for electricity price prediction. Energy,
162:1301–1314.
Rodriguez, C. P. and Anders, G. J. (2004). Energy price
forecasting in the ontario competitive power sys-
tem market. IEEE Transactions on power systems,
19(1):366–374.
Shafie-Khah, M., Moghaddam, M. P., and Sheikh-El-
Eslami, M. (2011). Price forecasting of day-ahead
electricity markets using a hybrid forecast method.
Energy Conversion and Management, 52(5):2165–
2169.
Singhal, D. and Swarup, K. (2011). Electricity price
forecasting using artificial neural networks. Interna-
tional Journal of Electrical Power & Energy Systems,
33(3):550–555.
Souhir, B. A., Heni, B., and Lotfi, B. (2019). Price risk
and hedging strategies in nord pool electricity mar-
ket evidence with sector indexes. Energy Economics,
80:635–655.
Ugurlu, U., Oksuz, I., and Tas, O. (2018). Electricity price
forecasting using recurrent neural networks. Energies,
11(5):1255.
Ventosa, M., Baıllo, A., Ramos, A., and Rivier, M. (2005).
Electricity market modeling trends. Energy policy,
33(7):897–913.
Weron, R. (2007). Modeling and forecasting electricity
loads and prices: A statistical approach, volume 403.
John Wiley & Sons.
Weron, R. (2014). Electricity price forecasting: A review
of the state-of-the-art with a look into the future. In-
ternational journal of forecasting, 30(4):1030–1081.
Zachmann, G. (2008). Electricity wholesale market prices
in europe: Convergence? Energy Economics,
30(4):1659–1671.
ICAART 2020 - 12th International Conference on Agents and Artificial Intelligence
834