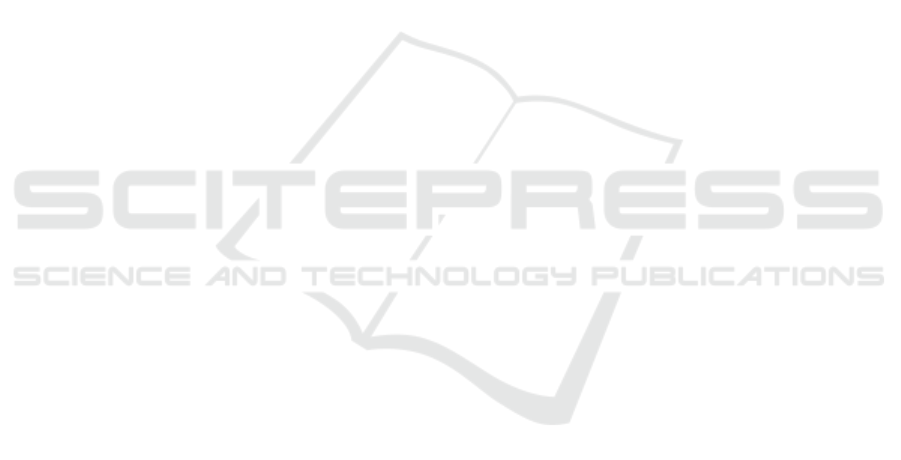
13th IEEE International Conference on, pages 263–
270. IEEE.
Li, J. and Lam, E. Y. (2015). Facial expression recogni-
tion using deep neural networks. In Imaging Systems
and Techniques (IST), 2015 IEEE International Con-
ference on, pages 1–6. IEEE.
Liu, M., Li, S., Shan, S., and Chen, X. (2013). Au-aware
deep networks for facial expression recognition. In
FG, pages 1–6.
Liu, P., Han, S., Meng, Z., and Tong, Y. (2014). Fa-
cial expression recognition via a boosted deep belief
network. In Proceedings of the IEEE Conference
on Computer Vision and Pattern Recognition, pages
1805–1812.
Liu, X., Kumar, B. V., You, J., and Jia, P. (2017). Adaptive
deep metric learning for identity-aware facial expres-
sion recognition. In CVPR Workshops, pages 522–
531.
Lopes, A. T., de Aguiar, E., De Souza, A. F., and Oliveira-
Santos, T. (2017). Facial expression recognition with
convolutional neural networks: coping with few data
and the training sample order. Pattern Recognition,
61:610–628.
Lucey, P., Cohn, J. F., Kanade, T., Saragih, J., Ambadar,
Z., and Matthews, I. (2010). The extended cohn-
kanade dataset (ck+): A complete dataset for action
unit and emotion-specified expression. In Computer
Vision and Pattern Recognition Workshops (CVPRW),
2010 IEEE Computer Society Conference on, pages
94–101. IEEE.
Lyons, M., Akamatsu, S., Kamachi, M., and Gyoba,
J. (1998). Coding facial expressions with gabor
wavelets. In Automatic Face and Gesture Recognition,
1998. Proceedings. Third IEEE International Confer-
ence on, pages 200–205. IEEE.
Ouellet, S. (2014). Real-time emotion recognition for gam-
ing using deep convolutional network features. arXiv
preprint arXiv:1408.3750.
Pons, G. and Masip, D. (2018). Multi-task, multi-label and
multi-domain learning with residual convolutional
networks for emotion recognition. arXiv preprint
arXiv:1802.06664.
Reed, S., Sohn, K., Zhang, Y., and Lee, H. (2014). Learn-
ing to disentangle factors of variation with manifold
interaction. In International Conference on Machine
Learning, pages 1431–1439.
Rifai, S., Bengio, Y., Courville, A., Vincent, P., and Mirza,
M. (2012). Disentangling factors of variation for facial
expression recognition. In Computer Vision–ECCV
2012, pages 808–822. Springer.
Salmam, F. Z., Madani, A., and Kissi, M. (2018). Emotion
recognition from facial expression based on fiducial
points detection and using neural network. Interna-
tional Journal of Electrical and Computer Engineer-
ing, 8(1):52.
Shan, K., Guo, J., You, W., Lu, D., and Bie, R. (2017). Au-
tomatic facial expression recognition based on a deep
convolutional-neural-network structure. In Software
Engineering Research, Management and Applications
(SERA), 2017 IEEE 15th International Conference on,
pages 123–128. IEEE.
Shang, W., Sohn, K., Almeida, D., and Lee, H. (2016). Un-
derstanding and improving convolutional neural net-
works via concatenated rectified linear units. In In-
ternational Conference on Machine Learning, pages
2217–2225.
Shikkenawis, G. and Mitra, S. K. (2016). On some vari-
ants of locality preserving projection. Neurocomput-
ing, 173:196–211.
Simard, P. Y., Steinkraus, D., and Platt, J. C. (2003). Best
practices for convolutional neural networks applied to
visual document analysis. In null, page 958. IEEE.
Simonyan, K. and Zisserman, A. (2014). Very deep con-
volutional networks for large-scale image recognition.
arXiv preprint arXiv:1409.1556.
Su, W., Chen, L., Wu, M., Zhou, M., Liu, Z., and Cao, W.
(2017). Nesterov accelerated gradient descent-based
convolution neural network with dropout for facial ex-
pression recognition. In Control Conference (ASCC),
2017 11th Asian, pages 1063–1068. IEEE.
Szegedy, C., Liu, W., Jia, Y., Sermanet, P., Reed, S.,
Anguelov, D., Erhan, D., Vanhoucke, V., and Rabi-
novich, A. (2015). Going deeper with convolutions.
In Proceedings of the IEEE conference on computer
vision and pattern recognition, pages 1–9.
Taheri, S., Qiu, Q., and Chellappa, R. (2014). Structure-
preserving sparse decomposition for facial expression
analysis. IEEE Transactions on Image Processing,
23(8):3590–3603.
Yang, H., Ciftci, U., and Yin, L. (2018). Facial expression
recognition by de-expression residue learning. In Pro-
ceedings of the IEEE Conference on Computer Vision
and Pattern Recognition, pages 2168–2177.
Yao, A., Cai, D., Hu, P., Wang, S., Sha, L., and Chen, Y.
(2016). Holonet: towards robust emotion recognition
in the wild. In Proceedings of the 18th ACM Interna-
tional Conference on Multimodal Interaction, pages
472–478. ACM.
Zeng, N., Zhang, H., Song, B., Liu, W., Li, Y., and
Dobaie, A. M. (2018). Facial expression recognition
via learning deep sparse autoencoders. Neurocomput-
ing, 273:643–649.
Zhang, F., Zhang, T., Mao, Q., and Xu, C. (2018). Joint
pose and expression modeling for facial expression
recognition. In Proceedings of the IEEE Conference
on Computer Vision and Pattern Recognition, pages
3359–3368.
Zhao, G., Huang, X., Taini, M., Li, S. Z., and Pietik
¨
aInen,
M. (2011). Facial expression recognition from
near-infrared videos. Image and Vision Computing,
29(9):607–619.
Zhao, X., Shi, X., and Zhang, S. (2015). Facial expression
recognition via deep learning. IETE technical review,
32(5):347–355.
DNNFG: DNN based on Fourier Transform Followed by Gabor Filtering for the Modular FER
219