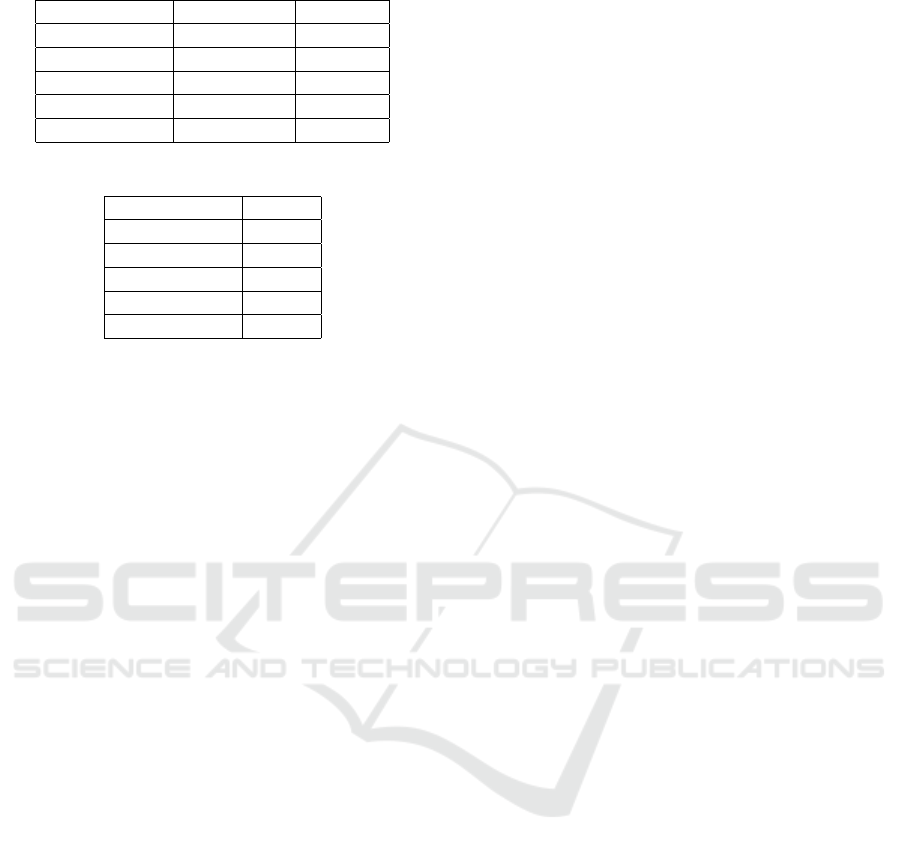
Table 1: Training/Test accuracies.
Classification Method Training accuracy Test accuracy
LR 0.53 0.52
BR 0.87 0.60
LDA 0.93 0.40
SVM 0.87 0.80
DNN 0.86 0.80
Table 2: Classification results in terms of MMSE.
Classification Method MMSE
LR 0.3404
BR 0.6324
LDA 0.7745
SVM 0.3638
DNN 0.4472
(hardware tasks). The observation on the classi-
fication results favors SVM learning algorithm for
classification problems since the correctness percent-
age is high compared to the competitive algorithms.
Though, the differences are not much.
The results reported in Tables 1 and 2, therefore,
prove the success of these approaches recognize task
type through the implicit relationships between input
and output variables. From these results, both DNN
and SVM are the most successful approaches to pre-
dict the task type with the highest accuracy and with-
out complex procedure.
4 CONCLUSION AND FUTURE
WORK
In this paper, we investigated the feasibility of su-
pervised learning algorithms for hardware/software
partitioning. Experiments were conducted using a
database that were collected from real tasks within
Altran technologies.
In fact, In general, this paper talks about classifi-
cation of functions in terms of their implementation
through hardware or software to raise the efficiency
of the system in terms of time and energy consump-
tion for FPGA-based Applications. Basically, it in-
troduces hardware/software partitioning algorithms in
order to solve the classification task in real-time sys-
tems. Such a system consists of 6 parts. First , the
database where we contain several tasks with 3 pa-
rameters, Energy, Allocations, Execution time and all
together with their types. Second, Linear Regression
model as the task type is represented linearly with the
three database parameters each multiplied by coeffi-
cient. This model aims to minimize the cost by find-
ing the best proper coefficient value for each parame-
ter. Third, Logistic Regression, where it differs from
the linear regression model as the calculation of the
weight based on the probability that falls into one of
two categories. Mainly, it is the statistical technique
that used to predict the relationship between inputs.
Fourth, Linear Discriminant Analysis (LDA), where it
aims to characterize or separate different classes that
share linear features in order to optimize the compu-
tations. Fifth, Support Vector Machine (SVM), as it
used to classify the data by finding a hyperplane in an
N-dimensional space where N is the number of fea-
tures. Finally, the last part of this paper is Deep Neu-
ral Network (DNN). DNN consists of 4 layers where
2 are hidden. The inputs of the trained DNN are the
three database parameters and the output is the item
type. Each layer have number of neurons with acti-
vation function. After applying these algorithms on
real experimentations on FPGA and CPU, we found
that they are efficient especially DNN and SVN algo-
rithms where they were more accurate in classifying
task type.
Hence, we prove the applicability of these meth-
ods and the high accuracy of the task type decision.
The tested models were trained offline based on
the collected data from real experimentations on
FPGA and CPU. The evaluation results show the ef-
fectiveness of AI in solving the partitioning prob-
lem. For real-time applications, it is important for the
tested methods to have a good generalization ability
so that they can still work effectively when the con-
ditions of online deployment could not be satisfied in
the training stage (Mohammad and Hannachi, 2019) ,
(Mohamed, 2019). Hence, motivated by the promis-
ing results, future work may address the unsupervised
learning for a hardware/software partitioning in order
to achieve a fully autonomous real-time system.
REFERENCES
Agostinelli, F., Hoffman, M., Sadowski, P., and Baldi, P.
(2014). Learning activation functions to improve deep
neural networks. arXiv preprint arXiv:1412.6830.
Alawieh, M., Kasparek, M., Franke, N., and Hupfer, J.
(2015). A high performance fpga-gpu-cpu platform
for a real-time locating system. In 2015 23rd Eu-
ropean Signal Processing Conference (EUSIPCO),
pages 1576–1580. IEEE.
Antuvan, C. W. and Masia, L. (2019). An lda-based
approach for real-time simultaneous classification of
movements using surface electromyography. IEEE
Transactions on Neural Systems and Rehabilitation
Engineering, 27(3):552–561.
Gola, J., Webel, J., Britz, D., Guitar, A., Staudt, T., Winter,
M., and M
¨
ucklich, F. (2019). Objective microstructure
classification by support vector machine (svm) using
Supervised Hardware/Software Partitioning Algorithms for FPGA-based Applications
863