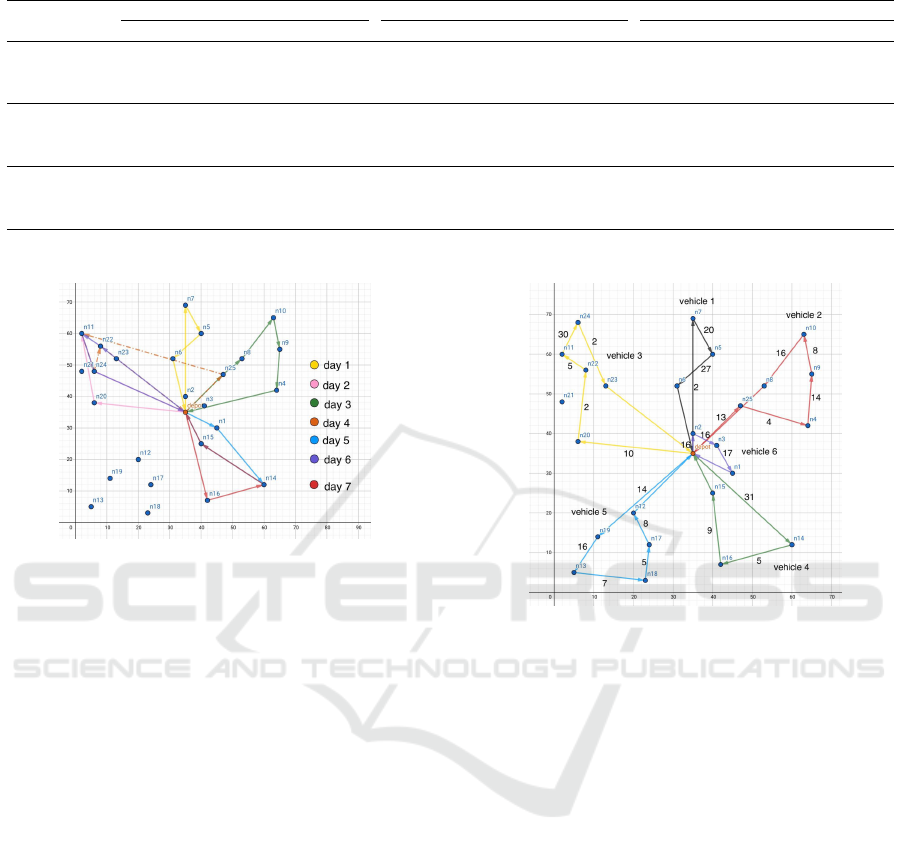
Table 3: Solution weighted costs for the modified Solomon’s Dataset.
Setup 1 Setup 2 Setup 3
Datasets Distance Unsatisfied Variance Weekly cost Distance Unsatisfied Variance Weekly cost Distance Unsatisfied Variance Weekly cost
Dataset 1-Type R 3,717 5,257,411 39,755 5,300,883 3,789 6,519,331 53,007 6,576,127 3,999 4,849,787 26,504 4,880,290
Dataset 2-Type R 3,751 11,555,526 92,762 11,652,039 4,709 12,321,124 92,762 12,418,595 4,036 11,780,452 92,762 11,877,251
Dataset 3-Type R 3,465 8,486,067 66,259 8,555,791 4,058 8,170,676 79,511 8,254,244 4,091 7,094,634 53,007 7,151,732
Dataset 4-Type R 2,670 9,910,719 66,259 9,979,648 3,467 11,361,697 106,014 11,471,178 3,042 9,741,450 119,266 9,863,757
Dataset 1-Type C 2,510 19,648,281 159,021 19,809,813 2,460 19,660,473 145,769 19,808,702 2,320 19,682,029 172,273 19,856,622
Dataset 2-Type C 2,276 12,176,945 119,266 12,298,487 2,760 13,080,714 106,014 13,189,488 2,627 12,211,753 92,762 12,307,142
Dataset 3-Type C 3,482 24,555,669 172,273 24,731,424 3,445 24,554,433 172,273 24,730,150 3,393 24,610,267 172,273 24,785,932
Dataset 4-Type C 3,791 18,510,574 145,769 18,660,135 3,696 19,305,326 145,769 19,454,791 3,595 18,402,617 145,769 18,551,981
Dataset 1-Type RC 3,603 29,517,301 185,525 29,706,428 3,636 29,551,403 198,776 29,753,815 4,789 29,610,417 172,273 29,787,479
Dataset 2-Type RC 4,550 18,110,902 159,021 18,274,473 4,554 17,572,174 159,021 17,735,749 4,899 15,971,893 145,769 16,122,561
Dataset 3-Type RC 3,779 6,944,624 66,259 7,014,661 3,560 6,980,138 66,259 7,049,957 3,625 6,907,342 66,259 6,977,226
Dataset 4-Type RC 3,504 12,491,983 92,762 12,588,249 3,468 13,007,388 106,014 13,116,870 3,551 12,118,460 145,769 12,267,781
where ω
1
= 1, ω
2
= 17669 and ω
3
= 1325175
Remark: Bold texts present the best objective value among the three setups.
Figure 1: A routing plan of vehicle 1 for dataset 2 type R.
did not perform well when considered weekly costs,
unsatisfied demands and supply distribution variance.
Overall, Setup 3 found solutions with the lowest
weekly cost, which contributed from low unsatisfied
demands and delivered supply variance. Setup 1 had
the shortest total distance.
As we mention that we preferred a solution with
equal supplies distribution. From this view, we can
conclude that Setup 3 is the best setup because it pro-
vided the best solution for seven instances. From Ta-
ble 3, we recommended that the dataset type R should
be solved by applying Setup 1 because it provides the
best overall costs. Next, we present a solution from
using Setup 1 to solve Dataset 2-Type R.
Figure 1 illustrates a 7-day vehicle scheduling so-
lution for vehicle 1 applying setup 1 to solve Dataset
2-Type R. The instance has 25 visiting locations and
one service depot. There are six vehicles, each has
capacity of 50 units. From the figure, vehicle 1 is de-
ployed every day. There are locations where the vehi-
cle has visited multiple days such as a path from lo-
cation n
14
to location n
15
and return to depot on day 5
and day 7. The solution also shows the case where the
visiting order may not so efficient in term of travelling
distances, such as a route on day 1 (yellow path). This
is because the sub-problem solution is not the optimal
solution because the mathematical solver reaches the
Figure 2: A routing plan of all vehicles on the first period
using Setup 1.
computation time limit.
Figure 2 displays a one-day plan of all vehicles.
Each line represents a vehicle path to delivery com-
modities. Numbers on each line represent the number
of commodity to delivery at destination location. For
example, the plan for vehicle 5 is to delivery 14 units
at location n
19
, 16 units at location n
13
, 7 units at lo-
cation n
18
, 5 units at location n
17
, 8 units at location
n
12
and return to depot. From this one-day plan, six
vehicles make visits to 24 locations and only one lo-
cation, n
21
, has not been visited on day 1. The plan to
visit location n
21
is on day 3 and day 6.
6 CONCLUSIONS AND FUTURE
WORK
This paper explains a multi-day distribution problem
while supplies are shortage or there are transporta-
tion difficulties limiting supply delivery. The aim is
to build visiting plans having fair supply distribution
over planning horizon. We assume a constant de-
mand from every location every day, which then accu-
ICORES 2020 - 9th International Conference on Operations Research and Enterprise Systems
362