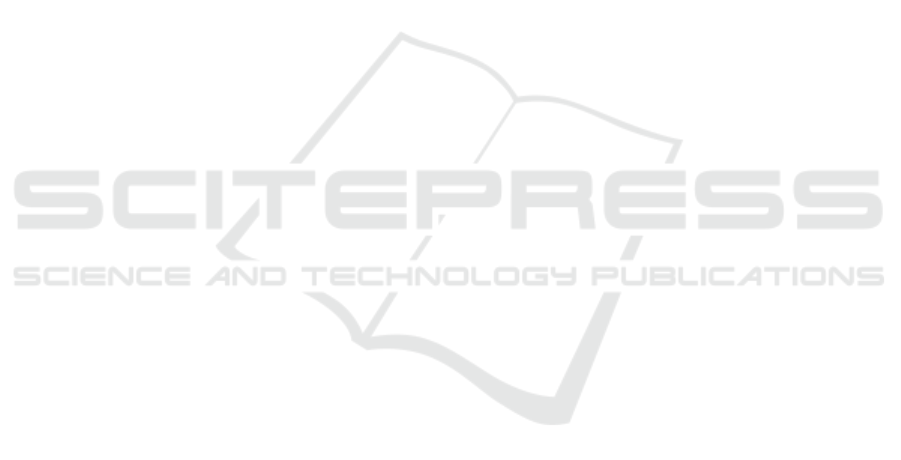
use which may be made of the information contained
therein.
REFERENCES
Bayar, B. and Stamm, M. C. (2016). A deep learning ap-
proach to universal image manipulation detection us-
ing a new convolutional layer. In Proceedings of the
4th ACM Workshop on Information Hiding and Multi-
media Security, pages 5–10. ACM.
Bayar, B. and Stamm, M. C. (2017). On the robustness
of constrained convolutional neural networks to jpeg
post-compression for image resampling detection. In
2017 IEEE International Conference on Acoustics,
Speech and Signal Processing (ICASSP), pages 2152–
2156. IEEE.
Bayram, S., Sencar, H., Memon, N., and Avcibas, I. (2005).
Source camera identification based on cfa interpola-
tion. In IEEE International Conference on Image Pro-
cessing 2005, volume 3, pages III–69. IEEE.
Bondi, L., Baroffio, L., G
¨
uera, D., Bestagini, P., Delp,
E. J., and Tubaro, S. (2016). First steps toward cam-
era model identification with convolutional neural net-
works. IEEE Signal Processing Letters, 24(3):259–
263.
Chang, C.-C. and Lin, C.-J. (2011). Libsvm: a library for
support vector machines. ACM transactions on intel-
ligent systems and technology (TIST), 2(3):27.
Chen, C. and Stamm, M. C. (2015). Camera model identi-
fication framework using an ensemble of demosaicing
features. In 2015 IEEE International Workshop on In-
formation Forensics and Security (WIFS), pages 1–6.
IEEE.
Chen, J., Kang, X., Liu, Y., and Wang, Z. J. (2015). Median
filtering forensics based on convolutional neural net-
works. IEEE Signal Processing Letters, 22(11):1849–
1853.
Cozzolino, D. and Verdoliva, L. (2019). Noiseprint: a cnn-
based camera model fingerprint. IEEE Transactions
on Information Forensics and Security.
Geradts, Z. J., Bijhold, J., Kieft, M., Kurosawa, K., Kuroki,
K., and Saitoh, N. (2001). Methods for identification
of images acquired with digital cameras. In Enabling
technologies for law enforcement and security, vol-
ume 4232, pages 505–513. International Society for
Optics and Photonics.
Gloe, T. and B
¨
ohme, R. (2010). The dresden image
database for benchmarking digital image forensics. In
Proceedings of the 2010 ACM Symposium on Applied
Computing, pages 1584–1590. Acm.
Holst, G. (1998). CCD arrays, cameras, and displays. JCD
Publishing.
Kharrazi, M., Sencar, H. T., and Memon, N. (2004). Blind
source camera identification. In 2004 International
Conference on Image Processing, 2004. ICIP’04., vol-
ume 1, pages 709–712. IEEE.
Kurosawa, K., Kuroki, K., and Saitoh, N. (1999). Ccd
fingerprint method-identification of a video camera
from videotaped images. In Proceedings 1999 In-
ternational Conference on Image Processing (Cat.
99CH36348), volume 3, pages 537–540. IEEE.
Li, C.-T. (2010). Source camera identification using en-
hanced sensor pattern noise. IEEE Transactions on
Information Forensics and Security, 5(2):280–287.
Luk
´
a
ˇ
s, J., Fridrich, J., and Goljan, M. (2006). Digital cam-
era identification from sensor pattern noise. IEEE
Transactions on Information Forensics and Security,
1(2):205–214.
Mayer, O. and Stamm, M. C. (2018). Learned forensic
source similarity for unknown camera models. In
2018 IEEE International Conference on Acoustics,
Speech and Signal Processing (ICASSP), pages 2012–
2016. IEEE.
Mayer, O. and Stamm, M. C. (2019). Forensic similarity for
digital images. arXiv preprint arXiv:1902.04684.
Qian, Y., Dong, J., Wang, W., and Tan, T. (2015). Deep
learning for steganalysis via convolutional neural net-
works. In Media Watermarking, Security, and Foren-
sics 2015, volume 9409, page 94090J. International
Society for Optics and Photonics.
Swaminathan, A., Wu, M., and Liu, K. R. (2007). Nonintru-
sive component forensics of visual sensors using out-
put images. IEEE Transactions on Information Foren-
sics and Security, 2(1):91–106.
Tuama, A., Comby, F., and Chaumont, M. (2016). Cam-
era model identification with the use of deep con-
volutional neural networks. In 2016 IEEE Interna-
tional workshop on information forensics and security
(WIFS), pages 1–6. IEEE.
Zhang, K., Zuo, W., Chen, Y., Meng, D., and Zhang, L.
(2017). Beyond a gaussian denoiser: Residual learn-
ing of deep cnn for image denoising. IEEE Transac-
tions on Image Processing, 26(7):3142–3155.
Zhu, X., Qian, Y., Zhao, X., Sun, B., and Sun, Y. (2018). A
deep learning approach to patch-based image inpaint-
ing forensics. Signal Processing: Image Communica-
tion, 67:90–99.
ICPRAM 2020 - 9th International Conference on Pattern Recognition Applications and Methods
584