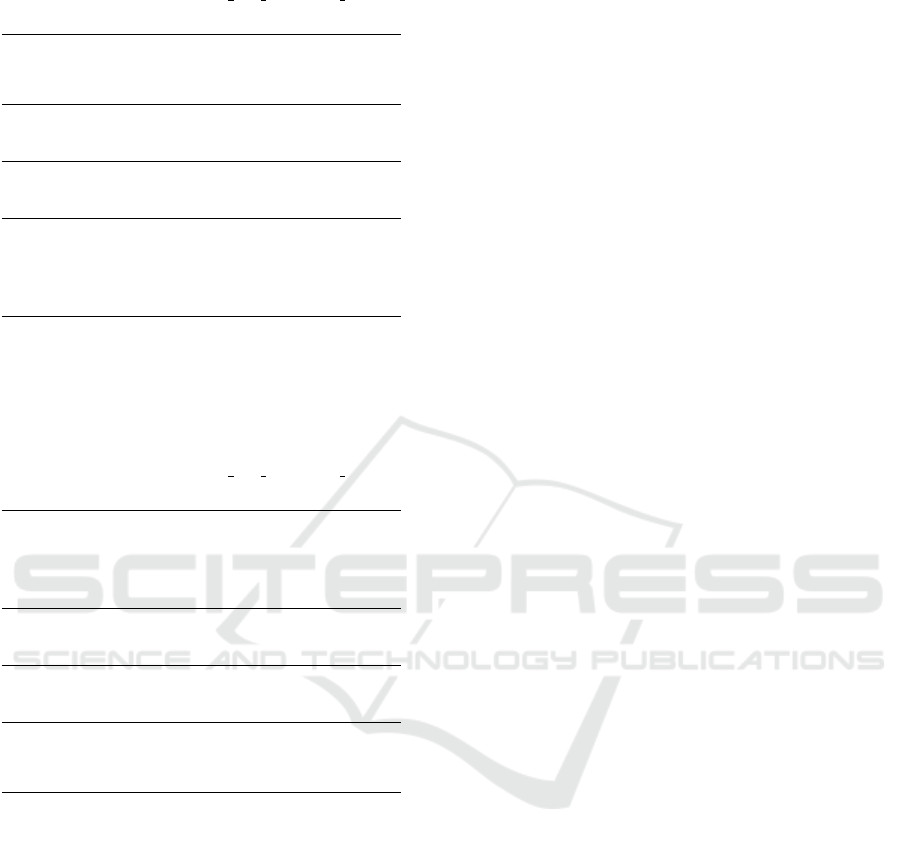
Table 2: Major Tweets and Contents of Top Five Micro-
Clusters of Burst1: Experiment 1 (s 1 = 0.5, s’ 2 = 0.2).
ID Major Tweets Contents
1 Kaneka was authorized as a child-rearing
support company by the the Health and
Welfare Ministry. I can’t believe it. We
cannot trust the authorization. Kaneka bul-
lies employees.
This micro-cluster shows the
beginning of condemnation for
Kaneka.
2 The page for childcare leave has been
deleted, so I’ll post it from the cache.
This micro-cluster contains
tweets about the deletion of
childcare pages from Kaneka’s
website.
3 Kaneka deleted the childcare leave pages,
because Twitter erupted with a post about
a male employee who has taken childcare
leave.
This micro-cluster directs us to
the site containing an archived
version of the deleted childcare
pages from Kaneka’s website.
4 I personally think that it is not his childcare
leave but his home building. There are a lot
of employees who were forced to leave the
house just after their home was completed.
Apparently it’s just after a mortgage, so it’s
likely that he won’t quit even if he makes a
violent personnel change.
This micro-cluster suggests
another reason for the com-
pany’s decision: construction
of his home.
5 I think that there are some old Japanese
companies that used excuses like “trans-
ferred when he bought a house” and “trans-
ferred when a child was born”. The era
has definitely changed since things will
be spread by SNS. Kaneka should be de-
feated.
This micro-cluster shows con-
demnation of Kaneka’s deci-
sion to transfer the employee.
Table 3: Major Tweets and Contents of Top Five Micro-
Clusters of Burst1: Experiment 2 (s 2 = 0.3, s’ 2 = 0.2).
ID Major Tweets Contents
1 I personally think that it is not his childcare
leave but his home building. There are a lot
of employees who were forced to leave the
house just after their home was completed.
Apparently it’s just after a mortgage, so it’s
likely that he won’t quit even if he make a
violent personnel change.
This micro-cluster is a com-
bined cluster consists of #1, #4
and #5 of Figure refF6.
2 The page for childcare leave has been
deleted, so I’ll post it from the cache.
This micro-cluster is also
related to the deletion of
childcare pages from Kaneka’s
website.
3 Kaneka deleted the childcare leave pages,
because Twitter erupted with a post about
a male employee who has taken childcare
leave.
This micro-cluster directs us
to a site containing an archive
of the deleted childcare pages
from Kaneka’s website.
4 It is a retirement issue due to forced trans-
fer, but if you are a shareholder, it is very
bad.
This micro-cluster examines
another reason for the com-
pany’s decision. It was not the
childcare leave, but his home
building.
5 Moreover, it is a clear violation of the law
not to allow paid vacation use. It is also
a violation for the company to specify the
retirement date. Even my previous job
(SMEs close to black) was paid when I quit
...
This micro-cluster is a com-
bined cluster for the condem-
nation for Kaneka’s decision
related to the transfer. Posters
are worries about the stock
price.
event in this burst.
For Burst2 and Burst3 in Figure 2, micro-
clustering can be adopted as well (Figure 7). To an-
alyze the micro-clusters in Figure 7, we detect the
causes of the burst.
5 CONCLUSION
This paper proposed a visualization method for ex-
amining bursting Twitter topics based on micro-
clustering. The finer degree of detail offered
by micro-clustering makes the differences between
users’ reactions clearer by subdividing those reactions
into subtopics. Evaluating micro-clusters over time
allows us to identify the causes of a target burst topic.
In our future work, we intend to apply our method
to different topics about a greater variety of events.
We also plan to propose a model for detecting topic
bursts in social media automatically.
ACKNOWLEDGEMENTS
This work was partially supported by JSPS KAK-
ENHI Grant Numbers 18K11443, 19K12125,
19H01133, 19J00871, and 17H00762.
REFERENCES
Blei, D. M., Ng, A. Y., and Jordan, M. I. (2003). Latent
dirichlet allocation. Journal of machine Learning re-
search, 3(Jan):993–1022.
Fujino, I. and Hoshino, Y. (2014). A method for identifying
topics in twitter and its application for analyzing the
transition of topics. In Proc. of DEIM 2014, C4-2.
Hashimoto, T., Uno, T., Kuboyama, T., Shin, K., and Shep-
ard, D. (2019a). Time series topic transition based on
micro-clustering. In 2019 IEEE International Confer-
ence on Big Data and Smart Computing (BigComp),
pages 1–8. IEEE.
Hashimoto, T., Uno, T., Kuboyama, T., Shin, K., and Shep-
ard, D. (2019b). Time series topic transition based on
micro-clustering. In 2019 IEEE International Confer-
ence on Big Data and Smart Computing (BigComp),
pages 1–8. IEEE.
Jaccard, P. (1912). The distribution of the flora in the alpine
zone. 1. New phytologist, 11(2):37–50.
Jaradat, S. and Matskin, M. (2019). On dynamic topic mod-
els for mining social media. In Emerging Research
Challenges and Opportunities in Computational So-
cial Network Analysis and Mining, pages 209–230.
Springer.
Jin, H., Toyoda, M., and Yoshinaga, N. (2017). Can cross-
lingual information cascades be predicted on twitter?
In International Conference on Social Informatics,
pages 457–472. Springer.
Kitada, T., Kazama, K., Toriumi, T. S. F., Kurihara, A.,
Shinoda, K., Noda, I., and Saito, K. (2015). Analysis
and visualization of topic series using tweets in great
east japan earthquake. In The 29th Annual Confer-
ence of the Japanese Society for Artificial Intelligence,
2B3-NFC-02a-1.
Kudo, T. (2006). Mecab: Yet another part-of-speech and
morphological analyzer. http://mecab. sourceforge. jp.
Kwak, H., Lee, C., Park, H., and Moon, S. (2010). What
is twitter, a social network or a news media? In
Proceedings of the 19th international conference on
World wide web, pages 591–600. AcM.
Twitter Topic Progress Visualization using Micro-clustering
591