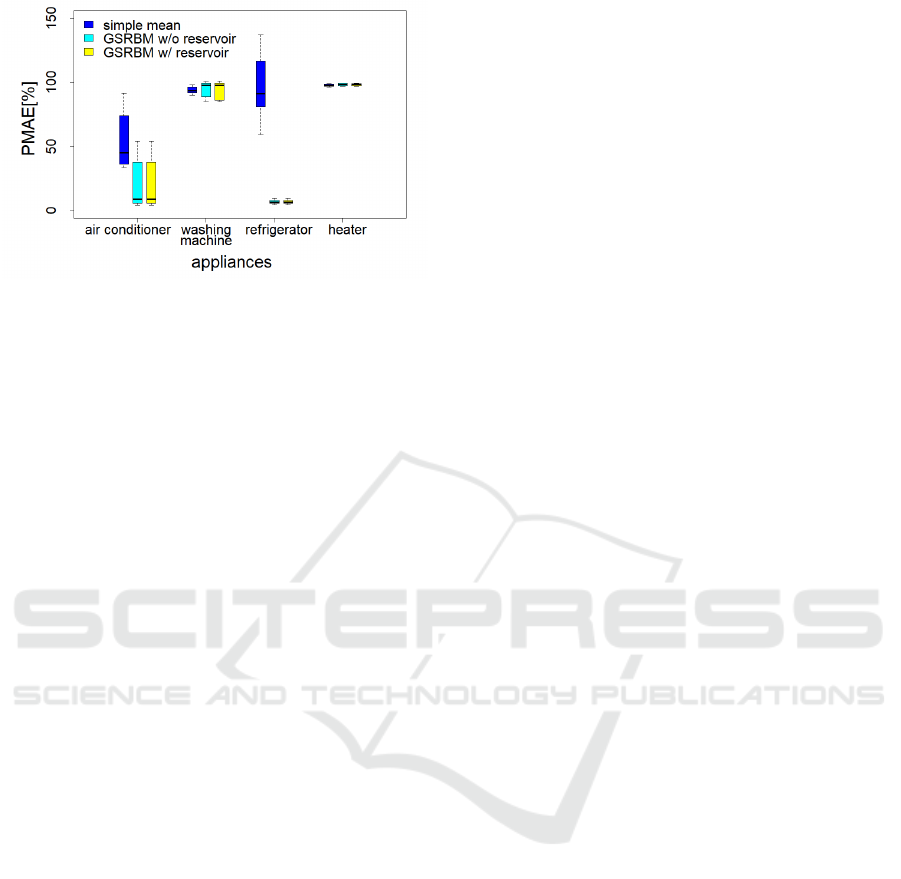
Figure 3: Comparison of appliance-wise percentage mean
absolute errors (PMAE
i
).
ered to reduce the estimation errors of the appliances
that only works occasionally, such as washing ma-
chines and heaters.
5 CONCLUDING REMARKS
In this study, we focused on a nonintrusive appliance
load monitoring scheme based on Gaussian-softmax
RBM, and proposed to introduce the temporal reser-
voir function for the purpose of modeling the nonlin-
ear temporal behavior of the appliance load consump-
tion. The proposed framework was evaluated from the
viewpoint of estimation accuracy by numerical exper-
iments. The experimental results show that the in-
troduction of the reservoir tends to improve the es-
timation accuracy of the appliance-wise energy con-
sumption. Thus, the proposed architecture will be a
promising framework for handling nonlinear tempo-
ral dynamics in appliance load.
Our experimental results suggested that an explicit
mechanism is required to further improve the estima-
tion accuracy for the appliances that only work occa-
sionally, such as washing machines and heaters. Ad-
ditionally, we also plan to develop a scheme utilizing
information stored in the reservoir during the NILM
process for the short-term forecast of appliance-wise
electricity consumption, though it remains as a future
work.
ACKNOWLEDGEMENTS
A part of this work was supported by the Japan Sci-
ence and Technology Agency (JST), Core Research
for Evolutional Science and Technology (CREST)
Program, under Grant JPMJCR15K5.
REFERENCES
Abate, F., Carrat
`
u, M., Liguori, C., Ferro, M., and Paciello,
V. (2018). Smart meter for the IoT. In 2018 IEEE In-
ternational Instrumentation and Measurement Tech-
nology Conference: Discovering New Horizons in In-
strumentation and Measurement, Proceedings, pages
1–6.
Bonfigli, R., Felicetti, A., Principi, E., Fagiani, M., Squar-
tini, S., and Piazza, F. (2018). Denoising autoencoders
for Non-Intrusive Load Monitoring: Improvements
and comparative evaluation. Energy and Buildings,
158:1461–1474.
Chatzis, S. P. and Demiris, Y. (2011). Echo state Gaus-
sian process. IEEE Transactions on Neural Networks,
22(9):1435–1445.
Cole, A. I. and Albicki, A. (1998). Algorithm for non-
Intrusive Identification of Residential Appliances. In
I, volume III, pages 338–341.
Farinaccio, L. and Zmeureanu, R. (1999). Using a Pattern
Recognition Approach to Disaggregate the Total Elec-
tricity COnsumption in a House into the Major End-
Uses. Energy and Buildings, 30:245–259.
Fischer, A. and Igel, C. (2014). Training restricted Boltz-
mann machines: An introduction. Pattern Recogni-
tion, 47(1):25–39.
Ghahramani, Z. and Jordan, M. I. (1997). Factorial Hidden
Markov Models. Machine Learning, 29:245–273.
Hart, G. W. (1992). Nonintrusive Appliance Load Monitor-
ing. Proceedings of the IEEE, 80(12):1870–1891.
He, W. and Chai, Y. (2016). An Empirical Study on En-
ergy Disaggregation via Deep Learning. In 2nd In-
ternational Conference on Artificial Intelligence and
Industrial Engineering, volume 133, pages 338–342.
Hinton, G. E. (2002). Training Products of Experts by Mini-
mizing Contrastive Divergence. Neural Computation,
14(8):1771–1800.
Hinton, G. E. (2012). A practical guide to training restricted
Boltzmann machines. In Neural Networks: Tricks
of the Trade, pages 599–619. Springer Berlin Heidel-
berg.
Hinton, G. E. and Salakhutdinov, R. R. (2006). Reduc-
ing the Dimensionality of Data with Neural Networks.
Science, 313:504–507.
Kelly, J. and Knottenbelt, W. (2015). Neural NILM: Deep
Neural Networks Applied to Energy Disaggregation.
In BuildSys@SenSys.
Kim, H., Marwah, M., Arlitt, M., Lyon, G., and Han, J.
(2011). Unsupervised Disaggregation of Low Fre-
quency Power Measurements. In SIAM Conference
on Data Mining 2011, pages 747–758.
Kolter, J. Z., Batra, S., and Ng, A. Y. (2010). Energy Disag-
gregation via Discriminative Sparse Coding. In Neu-
ral Information Processing Systems.
Kolter, J. Z. and Johnson, M. J. (2011). REDD: A Pub-
lic Data Set for Energy Disaggregation Research. In
Workshop on Data Mining Applications in Sustain-
ability.
ICAART 2020 - 12th International Conference on Agents and Artificial Intelligence
908