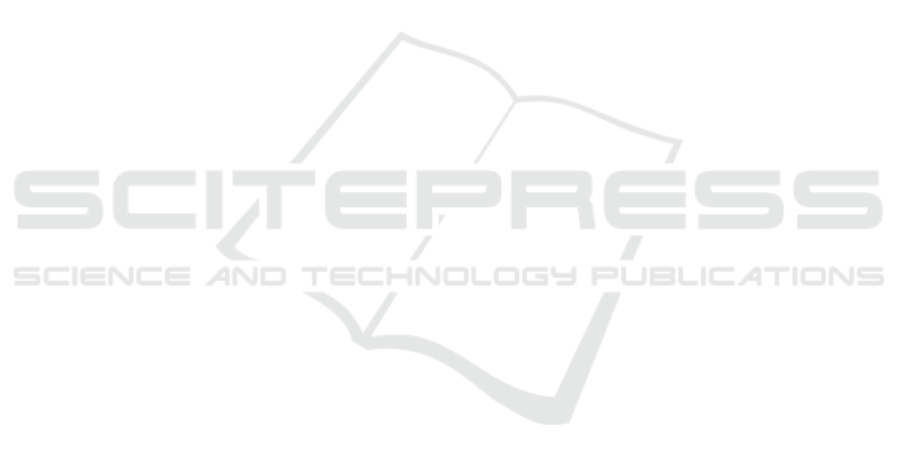
photo reconstruction: Application in breeding trials.
Remote Sensing, 7(4):4213–4232.
Diez., Y., Suzuki., T., Vila., M., and Waki., K. (2019). Com-
puter vision and deep learning tools for the automatic
processing of wasan documents. In Proceedings of
the 8th International Conference on Pattern Recogni-
tion Applications and Methods - Volume 1: ICPRAM,,
pages 757–765. INSTICC, SciTePress.
Erikson, M. and Olofsson, K. (2005). Comparison of three
individual tree crown detection methods. Machine Vi-
sion and Applications, 16(4):258–265.
Ester, M., Kriegel, H.-P., Sander, J., and Xu, X. (1996).
A density-based algorithm for discovering clusters in
large spatial databases with noise. In KKD-96 Pro-
ceedings, pages 226–231. AAAI Press.
Gambella, F., Sistu, L., Piccirilli, D., Corposanto, S., Caria,
M., Arcangeletti, E., Proto, A. R., Chessa, G., and
Pazzona, A. (2016). Forest and uav: A bibliometric
review. Contemporary Engineering Sciences, 9:1359–
1370.
Garc
´
ıa, E., Diez, Y., Diaz, O., Llad
´
o, X., Gubern-M
´
erida,
A., Mart
´
ı, R., Mart
´
ı, J., and Oliver, A. (2019). Breast
mri and x-ray mammography registration using gradi-
ent values. Medical Image Analysis, 54:76 – 87.
Gougeon, F. and Leckie, D. (2006). The individual tree
crown approach applied to ikonos images of a conif-
erous plantation area. Photogrammetric Engineering
& Remote Sensing, 72:1287–1297.
Gougeon, F. A. (1995). A crown-following approach to
the automatic delineation of individual tree crowns in
high spatial resolution aerial images. Canadian Jour-
nal of Remote Sensing, 21(3):274–284.
Grenzd
¨
orffer, G. and Teichert, B. (2008). The photogram-
metric potential of low-cost uavs in forestry and agri-
culture. Int. Arch. Photogramm. Remote Sens. Spatial
Inf. Sci., XXXVII.
Haralick, R. M. and Shapiro, L. G. (1992). Computer and
Robot Vision. Addison-Wesley Longman Publishing
Co., Inc., Boston, MA, USA, 1st edition.
Hern
´
andez Hern
´
andez, J. L., Garc
´
ıa-Mateos, G., Esquiva,
J. M., Escarabajal-Henarejos, D., Ruiz-Canales, A.,
and Mart
´
ınez, J. (2016). Optimal color space selec-
tion method for plant/soil segmentation in agriculture.
Computers and Electronics in Agriculture, 122:124–
132.
Hirschmugl, M., Ofner, M., Raggam, J., and Schardt, M.
(2007). Single tree detection in very high resolution
remote sensing data. Remote Sensing of Environment,
110(4):533 – 544. ForestSAT Special Issue.
Honkavaara, E., Saari, H., Kaivosoja, J., P
¨
ol
¨
onen, I.,
Hakala, T., Litkey, P., M
¨
akynen, J., and Pesonen, L.
(2013). Processing and assessment of spectromet-
ric, stereoscopic imagery collected using a lightweight
uav spectral camera for precision agriculture. Remote
Sensing, 5(10):5006–5039.
Hossein Mojaddadi Rizeei, Helmi Z. M. Shafri, M. A. M.
B. P. and Kalantar, B. (2018). Oil palm counting and
age estimation from worldview-3 imagery and lidar
data using an integrated obia height model and regres-
sion analysis. Journal of Sensors, 2018.
Jusoff, K. (2009). Sustainable management of a matured
oil palm plantation in upm campus, malaysia using
airborne remote sensing. Journal of Sustainable De-
velopment, 2.
Ke, Y. and Quackenbush, L. J. (2011). A comparison of
three methods for automatic tree crown detection and
delineation from high spatial resolution imagery. In-
ternational Journal of Remote Sensing, 32(13):3625–
3647.
Korpela, I., Dahlin, B., Sch
¨
afer, H., Bruun, E., Haa-
paniemi, F., Honkasalo, J., Ilvesniemi, S., Kuutti, V.,
Linkosalmi, M., Mustonen, J., Salo, M., Suomi, O.,
and Virtanen, H. (2007). Single-tree forest inventory
using lidar and aerial images for 3d treetop position-
ing, species recognition, height and crown width esti-
mation. Proc. IAPRS, 36.
Larsen, M., Eriksson, M., Descombes, X., Perrin, G.,
Brandtberg, T., and Gougeon, F. A. (2011). Compar-
ison of six individual tree crown detection algorithms
evaluated under varying forest conditions. Interna-
tional Journal of Remote Sensing, 32(20):5827–5852.
Li, W., Fu, H., Yu, L., and Cracknell, A. (2017). Deep
learning based oil palm tree detection and counting
for high-resolution remote sensing images. Remote
Sensing, 9(1).
Lloyd, S. (1982). Least squares quantization in pcm. IEEE
Transactions on Information Theory, 28(2):129–137.
Lopez, L., Hayashida, P., Mori, P., Koyama, P., Ashitani,
P., and Nobori, P. Y. (2014). 8th forest plan. Internal
Report.
Mubin, N. A., Nadarajoo, E., Shafri, H. Z. M., and Ha-
medianfar, A. (2019). Young and mature oil palm tree
detection and counting using convolutional neural net-
work deep learning method. International Journal of
Remote Sensing, 40(19):7500–7515.
Natesan, S., Armenakis, C., and Vepakomma, U. (2019).
Resnet-based tree species classification using uav im-
ages. ISPRS - International Archives of the Pho-
togrammetry, Remote Sensing and Spatial Informa-
tion Sciences, XLII-2/W13:475–481.
Onishi, M. and Ise, T. (2018). Automatic classification of
trees using a uav onboard camera and deep learning.
ArXiv, abs/1804.10390.
Paneque-G
´
alvez, J., McCall, M. K., Napoletano, B. M.,
Wich, S. A., and Koh, L. P. (2014). Small drones
for community-based forest monitoring: An assess-
ment of their feasibility and potential in tropical areas.
Forests, 5(6):1481–1507.
Pedregosa, F., Varoquaux, G., Gramfort, A., Michel, V.,
Thirion, B., Grisel, O., Blondel, M., Prettenhofer, P.,
Weiss, R., Dubourg, V., Vanderplas, J., Passos, A.,
Cournapeau, D., Brucher, M., Perrot, M., and Duch-
esnay, E. (2011). Scikit-learn: Machine Learning
in Python . Journal of Machine Learning Research,
12:2825–2830.
Pinz, A. (1989). Final results of the vision expert sys-
tem ves: Finding trees in aerial photographs. In Wis-
sensbasierte Mustererkennung, volume 49 of OCG-
Schriftenreihe, pages 90–111. .
ICPRAM 2020 - 9th International Conference on Pattern Recognition Applications and Methods
86