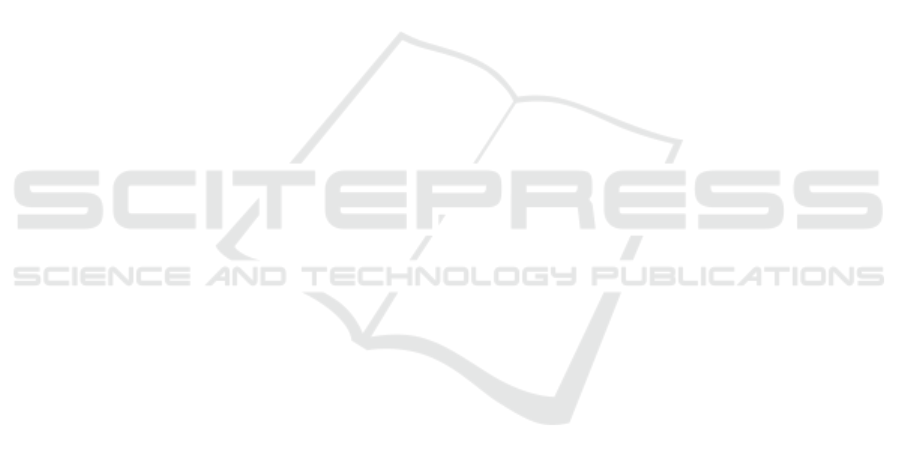
ACKNOWLEDGEMENTS
This work is partly supported by the NSFC
Research Program (61672065, 61906010), Bei-
jing Municipal Education Research Plan Project
(KM202010005032), China Postdoctoral Science
Foundation funded project (71007011201801), Bei-
jing Postdoctoral Research Foundation (2017-ZZ-
024), and Chaoyang Postdoctoral Research Founda-
tion (2018ZZ-01-05).
REFERENCES
Aimin Zhou, Bo-Yang Qu, H. L. S.-Z. Z. and Zhang, Q.
(2011). Multiobjective evolutionary algorithms: A
survey of the state of the art. Swarm and Evolutionary
Computation, volume 1, pages 32–49.
Bili Chen, Wenhua Zeng, Y. L. and Zhang, D. (2015).
A new local search-based multiobjective optimization
algorithm. IEEE Transactions on Evolutionary Com-
putation, volume 19, pages 50–73.
Bosman, P. and Thierens, D. (2003). The balance between
proximity and diversity in multiobjective evolutionary
algorithms. IEEE Transactions on Evolutionary Com-
putation, volume 7, pages 174–188.
Coello, C. A., Pulido, G. T., and Lechuga, M. S. (2004).
Handling multiple objectives with particle swarm op-
timization. IEEE Transactions on Evolutionary Com-
putation, volume 8, pages 256–279.
Corne, D., Knowles, J., and Oates, M. (2000). The pareto-
envelope based selection algorithm for multiobjective
optimization. InInternational Conference on Parallel
Problem Solving from Nature, pages 869–878.
Corne D W, Jerram N R, K. J. D. e. a. (2001). PESA-II:
Region-based selection in evolutionary multiobjective
optimization. In GECCO’2001’ Proceedings of the
Genetic and Evolutionary Computation Conference,
pages 283–290.
Deb (2001). Multi-objective Optimization using Evoulu-
tionary Algorithms. Chichester, john wiley & sons
edition.
Deb (2014). Multi-objective Optimization, Search method-
ologies. Springer, Boston,MA.
E. Elejalde, L. Ferres, E. H. and Bollen, J. (2018). Quanti-
fying the ecological diversity and health of online
news. Journal of computational science, volume 27,
pages 218–226.
Eckart, Z. and K
¨
unzli, S. (2004). Indicator-based selection
in multiobjective search. In in Proc. 8th International
Conference on Parallel Problem Solving from Nature
(PPSN VIII), pages 832–842. Springer.
Ge, H., Zhao, M., Sun, L., Wang, Z., Tan, G., Zhang, Q.,
and Chen, C. L. P. (2019). A many-objective evolu-
tionary algorithm with two interacting processes: Cas-
cade clustering and reference point incremental learn-
ing. IEEE Transactions on Evolutionary Computa-
tion, pages 1–1.
Huband, S., Barone, L., While, L., and Hingston, P. (2005).
A scalable multi-objective test problem toolkit. In
Evolutionary Multiobjective Optimization,Springer.
JD, K. and DW, C. (2000). Approximating the non-
dominated front using the pareto archived evoluton
strategy. IEEE Transactions on Evolutionary Com-
putation, volume 8, pages 149–172.
K. Deb, L. Thiele, M. L. and Zitzler, E. (2005). Scal-
able test problems for evolutionary multiobjective op-
timization. IEEE Transactions on Evolutionary Com-
putation, volume 20, pages 105–145. Springer.
K. Deb, A. Samir, P. A. and Meyarivan, T. (2000). A fast
elitist non-dominated sorting genetic algorithm for
multi-objective optimization: NSGA-II. IEEE Trans-
actions on Evolutionary Computation, pages 849–
858. Springer.
Li, M., Yang, S., and Liu, X. (2014). Shift-based den-
sity estimation for pareto-based algorithms in many-
objective optimization. IEEE Transactions on Evolu-
tionary Computation, volume 18, pages 348–365.
Margarita, R. and Coello, C. C. A. (2006). Multi-objective
particle swarm optimizers: A survey of the state-of-
the-art. International journal of computational in-
teligence research, volume 2, pages 287–308.
X. Cai, Z. F. and Zhang, Q. (2017). A conatrained decom-
position approach with grids for evolutionary multi-
objective optimization. IEEE Transactions on Evolu-
tionary Computation, volume 22, pages 564–557.
Yang, Shengxiang, L. M., Xiaohui, L., and Jinhua, Z.
(2013). A grid-based evolutionary algorithm for
many-objective optimization. IEEE Transactions on
Evolutionary Computation, volume 17, pages 721–
736.
Z. Eckart, K. J. and Lothar, T. (2008). Quality assessment
of pareto set approximations. In Evolutionary Mul-
tiobjective Optimization, volume 52, pages 373–404.
Springer.
Zhang, Q. and Li, H. (2007). MOEA/D: A multiobjec-
tive evolutionary algorithm based on decomposition.
In IEEE Transactions on Evolutionary Computation,
volume 11, pages 712–731.
A New Diversity Maintenance Strategy based on the Double Granularity Grid for Multiobjective Optimization
95