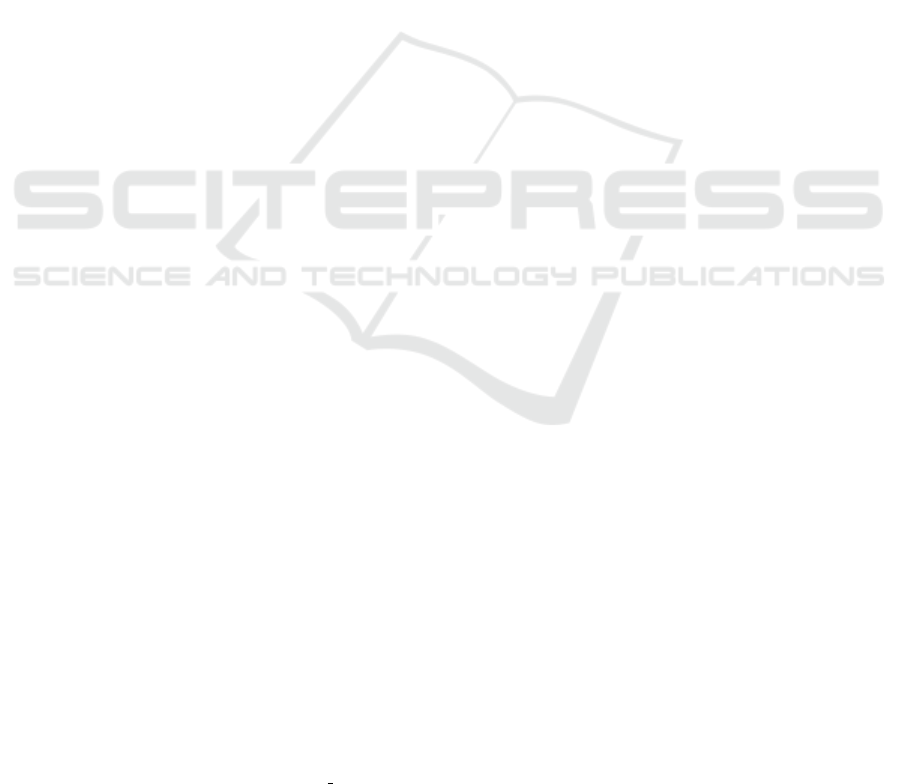
dia. In Proceedings of the 2016 CHI conference on hu-
man factors in computing systems, pages 2098–2110.
ACM.
Dorogush, A. V., Ershov, V., and Gulin, A. (2018). Cat-
boost: gradient boosting with categorical features sup-
port. arXiv preprint arXiv:1810.11363.
Ferwerda, B. and Tkalcic, M. (2018). You are what you
post: What the content of instagram pictures tells
about users personality. In The 23rd International on
Intelligent User Interfaces, March 7-11, Tokyo, Japan.
Ferwerda, B., Tkalcic, M., and Schedl, M. (2017). Person-
ality traits and music genres: What do people prefer
to listen to? In Proceedings of the 25th Conference
on User Modeling, Adaptation and Personalization,
pages 285–288. ACM.
Guntuku, S. C., Preotiuc-Pietro, D., Eichstaedt, J. C., and
Ungar, L. H. (2019). What twitter profile and posted
images reveal about depression and anxiety. In Pro-
ceedings of the International AAAI Conference on
Web and Social Media, volume 13, pages 236–246.
Huang, Y. C., Chiang, C.-F., and Chen, A. L. (2019). Pre-
dicting depression tendency based on image, text and
behavior data from instagram.
Iacobelli, F., Gill, A. J., Nowson, S., and Oberlander, J.
(2011). Large scale personality classification of blog-
gers. In international conference on affective com-
puting and intelligent interaction, pages 568–577.
Springer.
Kosinski, M., Stillwell, D., and Graepel, T. (2013). Pri-
vate traits and attributes are predictable from digital
records of human behavior. Proceedings of the Na-
tional Academy of Sciences, 110(15):5802–5805.
Kursuncu, U., Gaur, M., Lokala, U., Thirunarayan, K.,
Sheth, A., and Arpinar, I. B. (2019). Predictive analy-
sis on twitter: Techniques and applications. In Emerg-
ing Research Challenges and Opportunities in Com-
putational Social Network Analysis and Mining, pages
67–104. Springer.
Lin, T.-Y., Maire, M., Belongie, S., Hays, J., Perona, P.,
Ramanan, D., Doll
´
ar, P., and Zitnick, C. L. (2014).
Microsoft coco: Common objects in context. In Euro-
pean conference on computer vision, pages 740–755.
Springer.
Losada, D. E., Crestani, F., and Parapar, J. (2018).
Overview of erisk: early risk prediction on the in-
ternet. In International Conference of the Cross-
Language Evaluation Forum for European Lan-
guages, pages 343–361. Springer.
Moussavi, S., Chatterji, S., Verdes, E., Tandon, A., Pa-
tel, V., and Ustun, B. (2007). Depression, chronic
diseases, and decrements in health: results from the
world health surveys. The Lancet, 370(9590):851–
858.
Nolan, R. F., Dai, Y., and Stanley, P. D. (1995). An inves-
tigation of the relationship between color choice and
depression measured by the beck depression inven-
tory. Perceptual and motor skills, 81(3
suppl):1195–
1200.
Reece, A. G. and Danforth, C. M. (2017). Instagram pho-
tos reveal predictive markers of depression. EPJ Data
Science, 6(1):15.
Ren, S., He, K., Girshick, R., and Sun, J. (2015). Faster
r-cnn: Towards real-time object detection with region
proposal networks. In Advances in neural information
processing systems, pages 91–99.
Schwartz, H. A., Eichstaedt, J. C., Kern, M. L., Dziurzyn-
ski, L., Ramones, S. M., Agrawal, M., Shah, A.,
Kosinski, M., Stillwell, D., Seligman, M. E., et al.
(2013). Personality, gender, and age in the language
of social media: The open-vocabulary approach. PloS
one, 8(9):e73791.
Segalin, C., Cheng, D. S., and Cristani, M. (2017). So-
cial profiling through image understanding: Person-
ality inference using convolutional neural networks.
Computer Vision and Image Understanding, 156:34–
50.
Segalin, C., Perina, A., Cristani, M., and Vinciarelli, A.
(2016). The pictures we like are our image: contin-
uous mapping of favorite pictures into self-assessed
and attributed personality traits. IEEE Transactions
on Affective Computing, 8(2):268–285.
Shatte, A. B., Hutchinson, D. M., and Teague, S. J. (2019).
Machine learning in mental health: a scoping review
of methods and applications. Psychological medicine,
49(9):1426–1448.
Stankevich, M., Latyshev, A., Kuminskaya, E., Smirnov, I.,
and Grigoriev, O. (2019). Depression detection from
social media texts. In Selected Papers of the XXI In-
ternational Conference on Data Analytics and Man-
agement in Data Intensive Domains (DAMDID/RCDL
2019), pages 279–289.
Valdez, P. and Mehrabian, A. (1994). Effects of color on
emotions. Journal of experimental psychology: Gen-
eral, 123(4):394.
Widiger, T. A. and Mullins-Sweatt, S. N. (2010). Clini-
cal utility of a dimensional model of personality disor-
der. Professional Psychology: Research and Practice,
41(6):488.
Wieloch, M., Kabzi
´
nska, K., Filipiak, D., and Filipowska,
A. (2018). Profiling user colour preferences with bfi-
44 personality traits. In International Conference on
Business Information Systems, pages 63–76. Springer.
Wongkoblap, A., Vadillo, M. A., and Curcin, V. (2017). Re-
searching mental health disorders in the era of social
media: systematic review. Journal of medical Internet
research, 19(6):e228.
Yazdavar, A. H., Al-Olimat, H. S., Ebrahimi, M., Bajaj, G.,
Banerjee, T., Thirunarayan, K., Pathak, J., and Sheth,
A. (2017). Semi-supervised approach to monitoring
clinical depressive symptoms in social media. In Pro-
ceedings of the 2017 IEEE/ACM International Con-
ference on Advances in Social Networks Analysis and
Mining 2017, pages 1191–1198. ACM.
ICPRAM 2020 - 9th International Conference on Pattern Recognition Applications and Methods
240