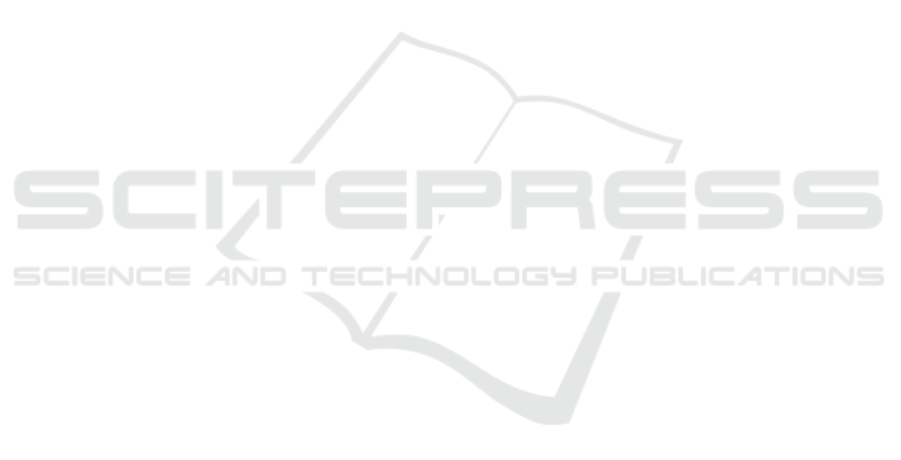
the Universities of Cambridge, East Anglia, Exeter,
and Queen Mary University of London.
REFERENCES
Alex, B. and Burns, J. (2014). Estimating and rating the
quality of optically character recognised text. In Pro-
ceedings of the First International Conference on Digi-
tal Access to Textual Cultural Heritage - DATeCH ’14,
pages 97–102, Madrid, Spain. ACM Press.
Alex, B., Grover, C., Klein, E., and Tobin, R. (2012). Digi-
tised Historical Text: Does It Have to Be MediOCRe?
In Proceedings of the 9th Conference on Natural Lan-
guage Processing (KONVENS 2012).
Ardanuy, M. C., McDonough, K., Krause, A., Wilson, D.
C. S., Hosseini, K., and van Strien, D. (2019). Resolv-
ing places, past and present: Toponym resolution in
historical british newspapers using multiple resources.
In Proceedings of the 13th Workshop on Geographic
Information Retrieval, GIR ’19, New York, NY, USA.
Association for Computing Machinery.
Azzopardi, L. and Vinay, V. (2008). Retrievability: an evalu-
ation measure for higher order information access tasks.
In Proceedings of the 17th ACM conference on Infor-
mation and knowledge management, pages 561–570.
ACM.
Blei, D. M., Ng, A. Y., and Jordan, M. I. (2003). Latent
dirichlet allocation. the Journal of machine Learning
research, 3:993–1022.
British Library Labs (2014). Digitised Books. c. 1510 -
c. 1900. JSON (OCR derived text). Available via:
https://doi.org/10.21250/db14.
Chiron, G., Doucet, A., Coustaty, M., and Moreux, J. (2017).
Icdar2017 competition on post-ocr text correction. In
2017 14th IAPR International Conference on Docu-
ment Analysis and Recognition (ICDAR), volume 01,
pages 1423–1428.
Cordell, R. (2017). "Q i-jtb the Raven": Taking Dirty OCR
Seriously. Book History, 20:188–225.
Cordell, R. (2019). Why You (A Humanist) Should Care
About Optical Character Recognition. Blog available
via https://ryancordell.org/research/why-ocr.
De Wilde, M. and Hengchen, S. (2017). Semantic enrich-
ment of a multilingual archive with linked open data.
Digital Humanities Quarterly, 11(4).
Ehrmann, M., Colavizza, G., Rochat, Y., and Kaplan, F.
(2016). Diachronic Evaluation of NER Systems on
Old Newspapers. Proceedings of the 13th Conference
on Natural Language Processing (KONVENS 2016),
pages 97–107.
Evershed, J. and Fitch, K. (2014). Correcting noisy OCR:
Context beats confusion. In Proceedings of the First
International Conference on Digital Access to Textual
Cultural Heritage, pages 45–51. ACM.
Franzini, G., Kestemont, M., Rotari, G., Jander, M., Ochab,
J. K., Franzini, E., Byszuk, J., and Rybicki, J. (2018).
Attributing Authorship in the Noisy Digitized Corre-
spondence of Jacob and Wilhelm Grimm. Frontiers in
Digital Humanities, 5:4.
Hagberg, A. A., Schult, D. A., and Swart, P. J. (2008).
Exploring network structure, dynamics, and function
using networkx. In Varoquaux, G., Vaught, T., and
Millman, J., editors, Proceedings of the 7th Python
in Science Conference, pages 11 – 15, Pasadena, CA
USA.
Hakala, K., Vesanto, A., Miekka, N., Salakoski, T., and
Ginter, F. (2019). Leveraging Text Repetitions
and Denoising Autoencoders in OCR Post-correction.
arXiv:1906.10907 [cs]. arXiv: 1906.10907.
Hämäläinen, M. and Hengchen, S. (2019). From the paft
to the fiiture: a fully automatic nmt and word embed-
dings method for ocr post-correction. arXiv preprint
arXiv:1910.05535.
Hamdi, A., Jean-Caurant, A., Sidere, N., Coustaty, M., and
Doucet, A. (2019). An Analysis of the Performance of
Named Entity Recognition over OCRed Documents. In
2019 ACM/IEEE Joint Conference on Digital Libraries
(JCDL), pages 333–334.
Hill, M. J. and Hengchen, S. (2019). Quantifying the impact
of dirty OCR on historical text analysis: Eighteenth
Century Collections Online as a case study. Digital
Scholarship in the Humanities.
Honnibal, M. and Montani, I. (2017). spaCy: Natural lan-
guage understanding with Bloom embeddings, convo-
lutional neural networks and incremental parsing. To
appear.
Howard, J. and Ruder, S. (2018). Universal language model
fine-tuning for text classification. In Proceedings of
the 56th Annual Meeting of the Association for Com-
putational Linguistics (Volume 1: Long Papers), pages
328–339, Melbourne, Australia. Association for Com-
putational Linguistics.
Jarlbrink, J. and Snickars, P. (2017). Cultural heritage as
digital noise: nineteenth century newspapers in the
digital archive. Journal of Documentation, 73(6):1228–
1243.
Jie, Z., Muis, A. O., and Lu, W. (2018). Efficient
dependency-guided named entity recognition. CoRR,
abs/1810.08436.
Leavy, S., Wade, K., Meaney, G., and Greene, D. (2018).
Navigating Literary Text with Word Embeddings and
Semantic Lexicons. In Workshop on Computational
Methods in the Humanities 2018, address = Luasanne,
Switzerland.
Levenshtein, V. I. (1966). Binary codes capable of correcting
deletions, insertions, and reversals. In Soviet physics
doklady, volume 10, pages 707–710.
Mikolov, T., Chen, K., Corrado, G., and Dean, J. (2013).
Efficient Estimation of Word Representations in Vector
Space. arXiv preprint.
Milligan, I. (2013). Illusionary Order: Online Databases,
Optical Character Recognition, and Canadian History,
1997–2010. Canadian Historical Review, 94(4):540–
569.
Mimno, D., Wallach, H. M., Talley, E., Leenders, M., and
McCallum, A. (2011). Optimizing semantic coherence
in topic models. In Proceedings of the Conference on
Empirical Methods in Natural Language Processing,
EMNLP ’11, pages 262–272, Stroudsburg, PA, USA.
Association for Computational Linguistics.
Assessing the Impact of OCR Quality on Downstream NLP Tasks
495