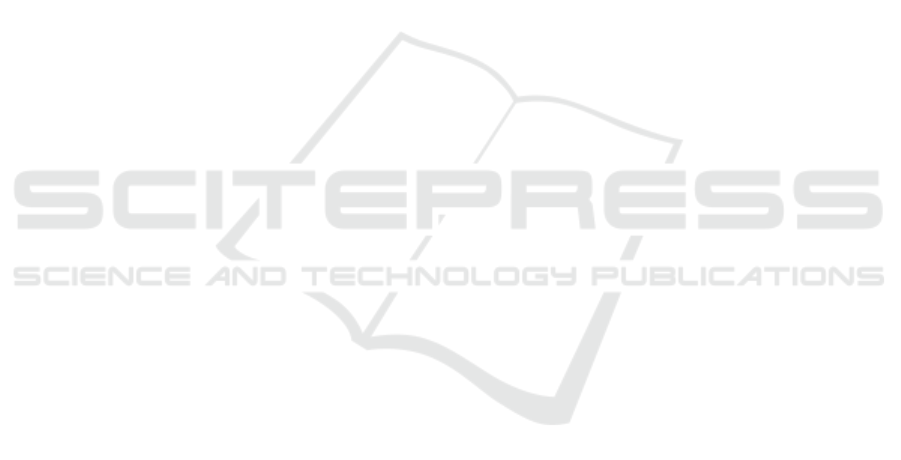
ranking performance simultaneously. The model is
ready for production with immediate application to
social media monitoring, campaign engagement fore-
casting, influence prediction, and maximization. We
propose the ability to engage the audience as a new,
more holistic baseline for social influence analysis.
We share the compound engagement workflow and
parameters (Eq. (3) and Table (4)) to ensure repro-
ducibility and inspire future work on engagement
modeling. We hope the future work will balance any
negative impact of diffusion-based influence maxi-
mization, on our collective attention and well-being.
ACKNOWLEDGEMENTS
This project is supported by Microsoft Development
Center Copenhagen and the Danish Innovation Fund,
Case No. 5189-00089B. We would like to acknowl-
edge the invaluable support of Sandeep Aparajit, J
¨
org
Derungs, Ralf Gautschi, Tomasz Janiczek, Charlotte
Mark, Pushpraj Shukla and Walter Sun. Any opin-
ions, findings, conclusions or recommendations ex-
pressed in this material are those of the authors and
do not necessarily reflect those of the sponsors.
REFERENCES
Agarwal, N., Dokoohaki, N., and Tokdemir, S., editors
(2019). Emerging Research Challenges and Opportu-
nities in Computational Social Network Analysis and
Mining. Lecture Notes in Social Networks. Springer
International Publishing, Cham.
Beyersdorf, B. (2019). Regulating the Most Accessible
Marketplace of Ideas in History: Disclosure Require-
ments in Online Political Advertisements after the
2016 Election. California Law Review, 107.
Bhattacharya, N., Arpinar, I. B., and Kursuncu, U. (2017).
Real Time Evaluation of Quality of Search Terms dur-
ing Query Expansion for Streaming Text Data Us-
ing Velocity and Relevance. In Proceedings - IEEE
11th International Conference on Semantic Comput-
ing, ICSC 2017, pages 280–281. Institute of Electrical
and Electronics Engineers Inc.
Bueno, C. C. (2016). The Attention Economy: Labour, Time
and Power in Cognitive Capitalism. Rowman & Lit-
tlefield International.
Bybee, K. J. and Jenkins, L. (2019). Free Speech, Free
Press, and Fake News: What If the Marketplace of
Ideas Isn’t About Identifying Truth? SSRN Electronic
Journal.
Can, E. F., Oktay, H., and Manmatha, R. (2013). Predicting
retweet count using visual cues. In Proceedings of the
22nd ACM international conference on Conference on
information & knowledge management - CIKM ’13.
Cappallo, S., Mensink, T., and Snoek, C. G. (2015). Latent
Factors of Visual Popularity Prediction. In Proceed-
ings of the 5th ACM on International Conference on
Multimedia Retrieval - ICMR ’15.
Cohen, J. (1988). Statistical Power Analysis for the Behav-
ioral Sciences. Lawrence Erlbaum Associates.
Davenport, T. H. and Beck, J. C. (2001). The attention
economy: Understanding the new currency of busi-
ness. Harvard Business Press.
Eshgi, S., Maghsudi, S., Restocchi, V., Stein, S., and Tassiu-
las, L. (2019). Efficient influence maximization under
network uncertainty. In INFOCOM 2019 Workshop
proceedings.
Fisher, W. D. (1958). On Grouping For Maximum Homo-
geneity. American Statistical Association Journal.
Franck, G. (2019). The economy of attention. Journal of
Sociology, 55(1):8–19.
Garimella, K., Weber, I., and De Choudhury, M. (2016).
Quote rts on twitter: Usage of the new feature for po-
litical discourse. In Proceedings of the 8th ACM Con-
ference on Web Science, WebSci ’16, pages 200–204,
New York, NY, USA. ACM.
Hansen, L. K., Arvidsson, A., Nielsen, F. A., Colleoni, E.,
and Etter, M. (2011). Good friends, bad news - Affect
and virality in twitter. In Communications in Com-
puter and Information Science.
Horn, J. L. (1965). A rationale and test for the number of
factors in factor analysis. Psychometrika, 30(2):179–
185.
Jorgensen, K. W. and Hansen, L. K. (2011). Model selection
for gaussian kernel pca denoising. IEEE transactions
on neural networks and learning systems, 23(1):163–
168.
Ke, G., Meng, Q., Wang, T., Chen, W., Ma, W., Liu, T.-
Y., Finley, T., Wang, T., Chen, W., Ma, W., Ye, Q.,
and Liu, T.-Y. (2017). LightGBM: A highly efficient
gradient boosting decision tree. Advances in Neural
Information Processing Systems.
Khosla, A., Das Sarma, A., and Hamid, R. (2014). What
makes an image popular? In Proceedings of the 23rd
international conference on World wide web - WWW
’14.
Kim, H., Jang, S. M., Kim, S.-H., and Wan, A. (2018).
Evaluating Sampling Methods for Content Analy-
sis of Twitter Data. Social Media + Society,
4(2):205630511877283.
Kowalczyk, D. K. and Larsen, J. (2019). Scalable Privacy-
Compliant Virality Prediction on Twitter. In Proceed-
ings of AffCon 2019 @ AAAI, volume 2328, pages 12–
27.
Lee, D., Hosanagar, K., and Nair, H. S. (2018). Advertis-
ing content and consumer engagement on social me-
dia: evidence from facebook. Management Science,
64(11):5105–5131.
Lorenz-Spreen, P., Mønsted, B. M., H
¨
ovel, P., and
Lehmann, S. (2019). Accelerating dynamics of collec-
tive attention. Nature Communications, 10(1):1759.
Mazloom, M., Rietveld, R., Rudinac, S., Worring, M., and
van Dolen, W. (2016). Multimodal Popularity Pre-
diction of Brand-related Social Media Posts. In Pro-
ICAART 2020 - 12th International Conference on Agents and Artificial Intelligence
924