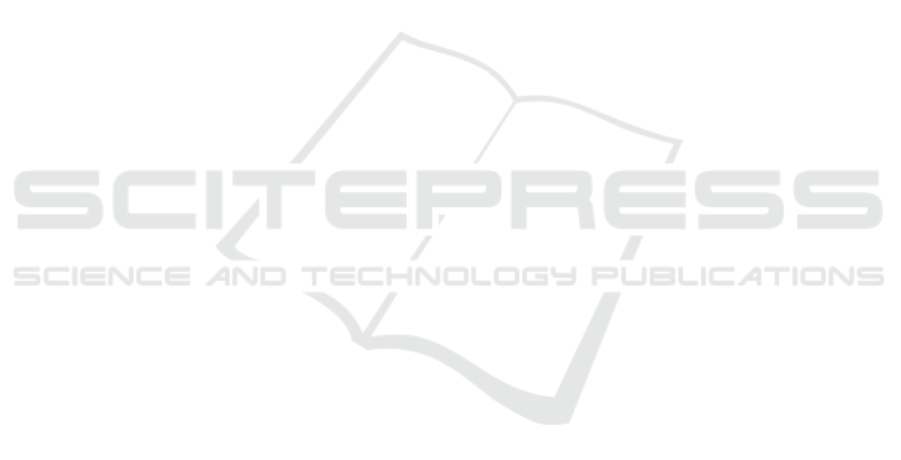
4 CONCLUSION
Automatic necrosis zone segmentation in follow-up
MR scans after RF ablations of spinal metastases has
the potential to quantify and objectify the treatment
outcome validation. It provides important informa-
tion regarding the improvement of ablation proce-
dures and it may help understanding and predicting
possible tumor reoccurrence. We proposed a CNN-
based segmentation approach and examined the im-
pact of various input modalities and dimensions on
the segmentation accuracy. Our results were on par
with those of Egger et al. (Egger et al., 2015), which
were the only quantitative results available (77.2.0 %
vs 77 %), altough the latter focused on necrotized
liver lesions in CT imaging. Overall, our study in-
dicates promising results and constitutes a valuale ap-
proach towards this ambitious and challenging issue.
ACKNOWLEDGEMENTS
This work was supported by the German Ministry
of Education and Research (13GW0095A) within the
STIMULATE research campus.
REFERENCES
Bricault, I., Kikinis, R., Morrison, P. R., vansonnenberg, E.,
Tuncali, K., and Silverman, S. G. (2006). Liver metas-
tases: 3d shape–based analysis of ct scans for detec-
tion of local recurrence after radiofrequency ablation.
Radiology, 241(1):243–250.
Chmelik, J., Jakubicek, R., Walek, P., et al. (2018). Deep
convolutional neural network-based segmentation and
classification of difficult to define metastatic spinal le-
sions in 3d ct data. Medical image analysis, 49:76–88.
Dupuy, D. E., Liu, D., Hartfeil, D., et al. (2010). Per-
cutaneous radiofrequency ablation of painful osseous
metastases. Cancer, 116:989–997.
Egger, J., Busse, H., Brandmaier, P., Seider, D., Gawlitza,
M., Strocka, S., Voglreiter, P., Dokter, M., Hofmann,
M., Kainz, B., et al. (2015). Rfa-cut: semi-automatic
segmentation of radiofrequency ablation zones with
and without needles via optimal st-cuts. In 2015 37th
Annual International Conference of the IEEE Engi-
neering in Medicine and Biology Society (EMBC),
pages 2423–2429. IEEE.
Harrington, K. (1986). Metastatic disease of the spine.
JBJS, 68:1110–1115.
Hille, G., D
¨
unnwald, M., Becker, M., Steffen, J., Saalfeld,
S., and T
¨
onnies, K. (2019). Segmentation of vertebral
metastases in mri using an u-net like convolutional
neural network. In Bildverarbeitung f
¨
ur die Medizin
2019, pages 31–36. Springer.
Isensee, F., Kickingereder, P., Bonekamp, D., Bendszus,
M., Wick, W., Schlemmer, H.-P., and Maier-Hein, K.
(2017). Brain tumor segmentation using large recep-
tive field deep convolutional neural networks. In Proc.
of Bildverarbeitung f
¨
ur die Medizin 2017, pages 86–
91. Springer.
Isensee, F., Kickingereder, P., Wick, W., Bendszus, M., and
Maier-Hein, K. H. (2019). No new-net. In Brain-
lesion: Glioma, Multiple Sclerosis, Stroke and Trau-
matic Brain Injuries, pages 234–244. Springer.
Kingma, D. P. and Ba, J. (2014). Adam: A method for
stochastic optimization. arXiv:1412.6980.
Klimo, P. and Schmidt, M. H. (2004). Surgical management
of spinal metastases. The Oncologist, 9:188–196.
McCreedy, E. S., Cheng, R., Hemler, P. F., Viswanathan, A.,
Wood, B. J., and McAuliffe, M. J. (2006). Radio fre-
quency ablation registration, segmentation, and fusion
tool. IEEE Transactions on Information Technology in
Biomedicine, 10(3):490–496.
Passera, K., Selvaggi, S., Scaramuzza, D., Garbagnati, F.,
Vergnaghi, D., and Mainardi, L. (2013). Radiofre-
quency ablation of liver tumors: quantitative assess-
ment of tumor coverage through ct image processing.
BMC Medical Imaging, 13:3.
Ronneberger, O., Fischer, P., and Brox, T. (2015). U-net:
Convolutional networks for biomedical image seg-
mentation. In Proc. of MICCAI 2015, pages 234–241.
Springer.
Rosenthal, D. I., Hornicek, F. J., Wolfe, M. W., et al. (1998).
Percutaneous radiofrequency coagulation of osteoid
osteoma compared with operative treatment. J Bone
Joint Surg Am, 80:815–21.
Salehi, S. S. M., Erdogmus, D., and Gholipour, A. (2017).
Tversky loss function for image segmentation us-
ing 3d fully convolutional deep networks. In Ma-
chine Learning in Medical Imaging, pages 379–387.
Springer.
Taha, A. A. and Hanbury, A. (2015). Metrics for evaluating
3d medical image segmentation: analysis, selection,
and tool. BMC medical imaging, 15(1):29.
Tversky, A. (1977). Features of similarity. Psychological
review, 84(4):327.
Weihusen, A., Hinrichsen, L., Carus, T., Dammer, R.,
Rascher-Friesenhausen, R., Kr
¨
oger, T., Peitgen, H.-
O., and Preusser, T. (2010). Towards a verified
simulation model for radiofrequency ablations. In
International Conference on Information Processing
in Computer-Assisted Interventions, pages 179–189.
Springer.
Wong, D. A., Fornasier, V. L., and MacNab, I. (1990).
Spinal metastases: the obvious, the occult, and the im-
postors. Spine, 15(1):1–4.
ICPRAM 2020 - 9th International Conference on Pattern Recognition Applications and Methods
102