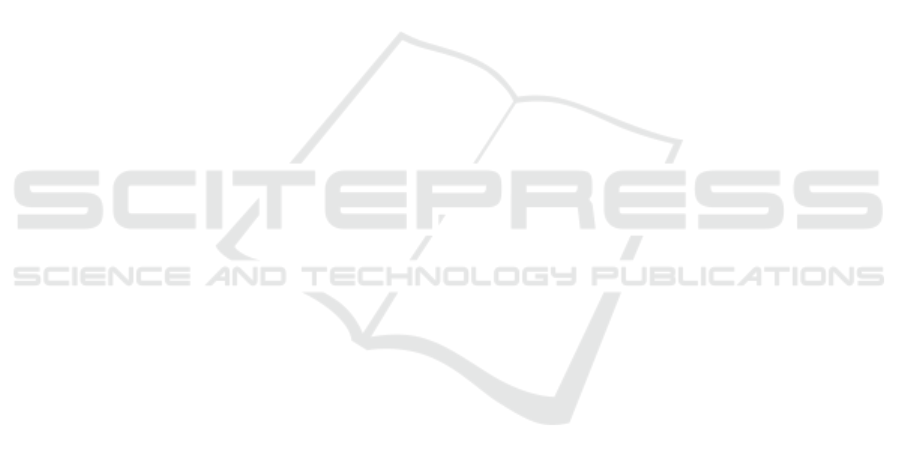
used to improve decisions made by farmers and in-
crease their profit. With such models, they can an-
ticipate almost any decision. Future direction is to
include more data and integrate new factors. We also
shed light on some interesting paths we should follow
in order to improve the results.
REFERENCES
Abadi, M., Barham, P., Chen, J., Chen, Z., Davis, A.,
Dean, J., Devin, M., Ghemawat, S., Irving, G., Isard,
M., et al. (2016). Tensorflow: A system for large-
scale machine learning. In 12th {USENIX} Sympo-
sium on Operating Systems Design and Implementa-
tion ({OSDI} 16), pages 265–283.
Auldist, M. and Hubble, I. (1998). Effects of mastitis on
raw milk and dairy products. Australian journal of
dairy technology, 53(1):28.
Bengio, Y., Simard, P., Frasconi, P., et al. (1994). Learning
long-term dependencies with gradient descent is diffi-
cult. IEEE transactions on neural networks, 5(2):157–
166.
Borchers, M., Chang, Y., Proudfoot, K., Wadsworth, B.,
Stone, A., and Bewley, J. (2017). Machine-learning-
based calving prediction from activity, lying, and ru-
minating behaviors in dairy cattle. Journal of dairy
science, 100(7):5664–5674.
Box, G. E., Jenkins, G. M., and Reinsel, G. (1970). Time se-
ries analysis: forecasting and control holden-day san
francisco. BoxTime Series Analysis: Forecasting and
Control Holden Day1970.
Box, G. E., Jenkins, G. M., Reinsel, G. C., and Ljung, G. M.
(2015). Time series analysis: forecasting and control.
John Wiley & Sons.
Bruinsma, J. (2017). World agriculture: towards
2015/2030: an FAO study. Routledge.
Calus, M., Goddard, M., Wientjes, Y., Bowman, P., and
Hayes, B. (2018). Multibreed genomic prediction us-
ing multitrait genomic residual maximum likelihood
and multitask bayesian variable selection. Journal of
dairy science, 101(5):4279–4294.
Canova, F. and Hansen, B. E. (1995). Are seasonal patterns
constant over time? a test for seasonal stability. Jour-
nal of Business & Economic Statistics, 13(3):237–
252.
Cho, K., Van Merri
¨
enboer, B., Gulcehre, C., Bahdanau, D.,
Bougares, F., Schwenk, H., and Bengio, Y. (2014).
Learning phrase representations using rnn encoder-
decoder for statistical machine translation. arXiv
preprint arXiv:1406.1078.
Choi, E., Bahadori, M. T., Schuetz, A., Stewart, W. F., and
Sun, J. (2016). Doctor ai: Predicting clinical events
via recurrent neural networks. In Machine Learning
for Healthcare Conference, pages 301–318.
Chollet, F. e. a. (2015). Keras. https://keras.io.
Contreras, J., Espinola, R., Nogales, F. J., and Conejo,
A. J. (2003). Arima models to predict next-day elec-
tricity prices. IEEE transactions on power systems,
18(3):1014–1020.
Dohoo, I. R. and Martin, S. (1984). Subclinical ketosis:
prevalence and associations with production and dis-
ease. Canadian Journal of Comparative Medicine,
48(1):1.
Emmons, D., Tulloch, D., and Ernstrom, C. (1990).
Product-yield pricing system. 1. technological consid-
erations in multiple-component pricing of milk. Jour-
nal of dairy science, 73(7):1712–1723.
Erdman, R. A. and Varner, M. (1995). Fixed yield responses
to increased milking frequency. Journal of dairy sci-
ence, 78(5):1199–1203.
Graves, A., Mohamed, A.-r., and Hinton, G. (2013).
Speech recognition with deep recurrent neural net-
works. In 2013 IEEE international conference on
acoustics, speech and signal processing, pages 6645–
6649. IEEE.
Graves, A. and Schmidhuber, J. (2009). Offline handwrit-
ing recognition with multidimensional recurrent neu-
ral networks. In Advances in neural information pro-
cessing systems, pages 545–552.
Grinblat, G. L., Uzal, L. C., Larese, M. G., and Granitto,
P. M. (2016). Deep learning for plant identification
using vein morphological patterns. Computers and
Electronics in Agriculture, 127:418–424.
Hochreiter, S. and Schmidhuber, J. (1997). Lstm can solve
hard long time lag problems. In Advances in neural
information processing systems, pages 473–479.
Hyndman, R. and Khandakar, Y. (2007). Automatic time
series forecasting: the forecast package for r 7, 2008.
URL http://www. jstatsoft. org/v27/i03.
Jones, J. W., Antle, J. M., Basso, B., Boote, K. J., Co-
nant, R. T., Foster, I., Godfray, H. C. J., Herrero, M.,
Howitt, R. E., Janssen, S., et al. (2017). Toward a
new generation of agricultural system data, models,
and knowledge products: State of agricultural systems
science. Agricultural systems, 155:269–288.
Kamilaris, A. and Prenafeta-Bold
´
u, F. X. (2018). Deep
learning in agriculture: A survey. Computers and elec-
tronics in agriculture, 147:70–90.
Kussul, N., Lavreniuk, M., Skakun, S., and Shelestov, A.
(2017). Deep learning classification of land cover and
crop types using remote sensing data. IEEE Geo-
science and Remote Sensing Letters, 14(5):778–782.
Kuwata, K. and Shibasaki, R. (2015). Estimating crop
yields with deep learning and remotely sensed data.
In 2015 IEEE International Geoscience and Remote
Sensing Symposium (IGARSS), pages 858–861. IEEE.
Kwiatkowski, D., Phillips, P. C., Schmidt, P., and Shin,
Y. (1992). Testing the null hypothesis of stationarity
against the alternative of a unit root: How sure are we
that economic time series have a unit root? Journal of
econometrics, 54(1-3):159–178.
Lipton, Z. C., Kale, D. C., Elkan, C., and Wetzel, R. (2015).
Learning to diagnose with lstm recurrent neural net-
works. arXiv preprint arXiv:1511.03677.
Maupom
´
e, D., Queudot, M., and Meurs, M.-J. (2019). In-
ter and intra document attention for depression risk
assessment. In Canadian Conference on Artificial In-
telligence, pages 333–341. Springer.
Nair, V. and Hinton, G. E. (2010). Rectified linear units
improve restricted boltzmann machines. In Proceed-
Towards an Effective Decision-making System based on Cow Profitability using Deep Learning
957