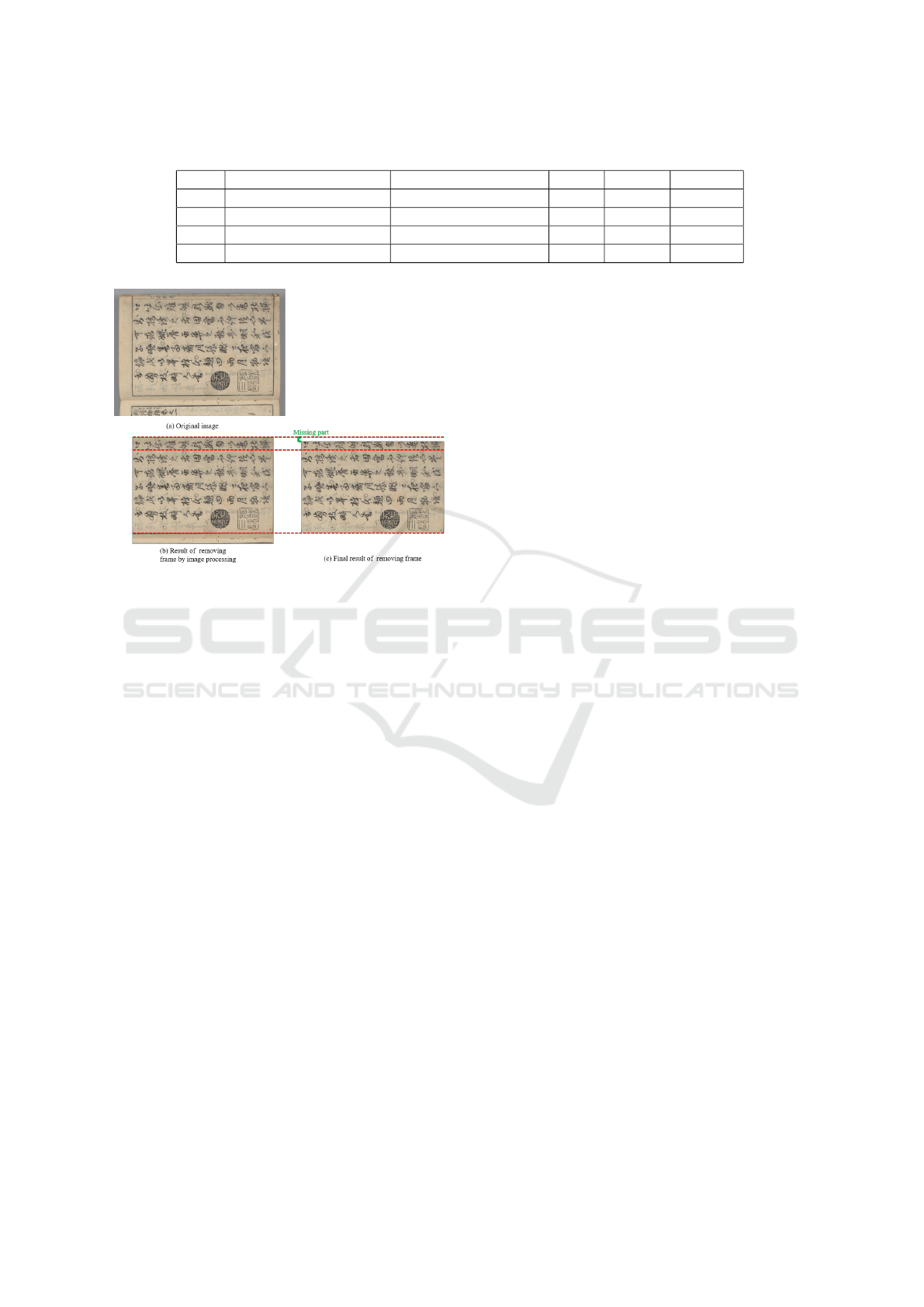
Table 1: Results of character recognition accuracy.
class training image numbers testing image numbers epoch time accuracy
10 19323 1000 200 0:10:35 0.930
20 28412 2000 300 0:32:28 0.884
30 35165 3000 400 1:06:44 0.899
40 41425 4000 500 1:49:01 0.890
Figure 12: Problems of processed image.
books to help automatic understanding. the proposal
includes ARU-Net based method and the two-step
method. In the experiment, the frame deletion of our
proposed method is greatly improved, which verifies
the correctness of our method. But the correctness of
the cut out blank part has not been verified, which will
be our future work. Moreover, our purpose is to real-
ize the automatic recognition of early Japanese books,
so the automatic extraction of text lines and automatic
character recognition are also our future work.
ACKNOWLEDGEMENTS
This research is supported by the Art Research Cen-
ter of Ritsumeikan University. In addition, We would
like to thank Prof. Akama Ryo Prof.Takaaki Kaneko
for his advice.
REFERENCES
A. Krizhevsky, I. Sutskever, G. H. (2012). Imagenetclassi-
fication with deep convolutional neural networks. Ad-
vances in Neural Information Processing System Sys-
tems 25.
Bing Lyu, Ryo Akama, H. T. and Meng, L. (2019). The
early japanese books text line segmentation base on
image processing and deep learning. In The 2019
International Conference on Advanced Mechatronic
Systems (ICAMechS 2019).
C. Szegedy, W. Liu, Y. J. P. S. S. R. D. A. D. E. V. V. A. R.
(2015). Goingdeeper with convolutions. 2015 IEEE
Conference on Computer Vision and Pattern Recogni-
tion.
C.C. Tappert, C.Y. Suen, T. W. (1990). U-net: Convolu-
tional networks for biomedical image segmentation.
In IEEE Transactions on Pattern Analysis and Ma-
chine Intelligence.
K. He, X. Zhang, S. R. J. S. (2016). Deep residual learn-
ing for image recognition. 2016 IEEE Conference on
Computer Vision and Pattern Recognition.
L. Meng, C.V. Aravinda, K. R. U. K. R. e. a. (2018). An-
cient asian character recognition for literature preser-
vation and understanding. In Euromed 2018 Interna-
tional Conference on Digital Heritage. Springer Na-
ture.
L. Meng, T. I. and Oyanagi, S. (2016). Recognition of orac-
ular bone inscriptions by clustering and matching on
the hough space. J. of the Institute of Image Electron-
ics Engineers of Japan, 44(4):627–636.
L. Meng, Y. F. e. a. (2016). Recognition of oracular bone
inscriptions using template matching. Int. J. of Com-
puters Theory and Engineering, 8(1):53–57.
L. Yann, L. Bottou, Y. B. and Haffner, P. (1998). Gradient-
based learning applied to document recognition. In
Proceeding of the IEEE.
Meng, L. (2017). Recognition of oracle bone inscriptions by
extracting line features on image processing. In Pro. of
the 6th Int. Conf. on Pattern Recognition Applications
and Methods (ICPRAM2017).
Redmon and Farhadi, A. (2018). Yolov3: An incremental
improvement. In Computer Vision and Pattern Recog-
nition (ECCV 2018).
Simonyan, K. and Zisserman, A. (2015). Very deep convo-
lutional networks for large-scale image recognition. In
Advances in Neural Information Processing Systems
28 (NIPS 2015).
T. Grning, G. Leifert, T. S. and Labahn, R. (2018). A two-
stage method for text line detection in historical doc-
uments. In Computer Vision and Pattern Recognition.
W. Liu, D. Anguelov, D. C. S. S. R. C. F. and Berg, A. C.
(2016). Ssd: Single shot multibox detector. In Com-
puter Vision and Pattern Recognition (ECCV 2016).
Zhou, X., Wang, D., and Kr
¨
ahenb
¨
uhl, P. (2019). Objects as
points. In arXiv preprint arXiv:1904.07850.
ICPRAM 2020 - 9th International Conference on Pattern Recognition Applications and Methods
606