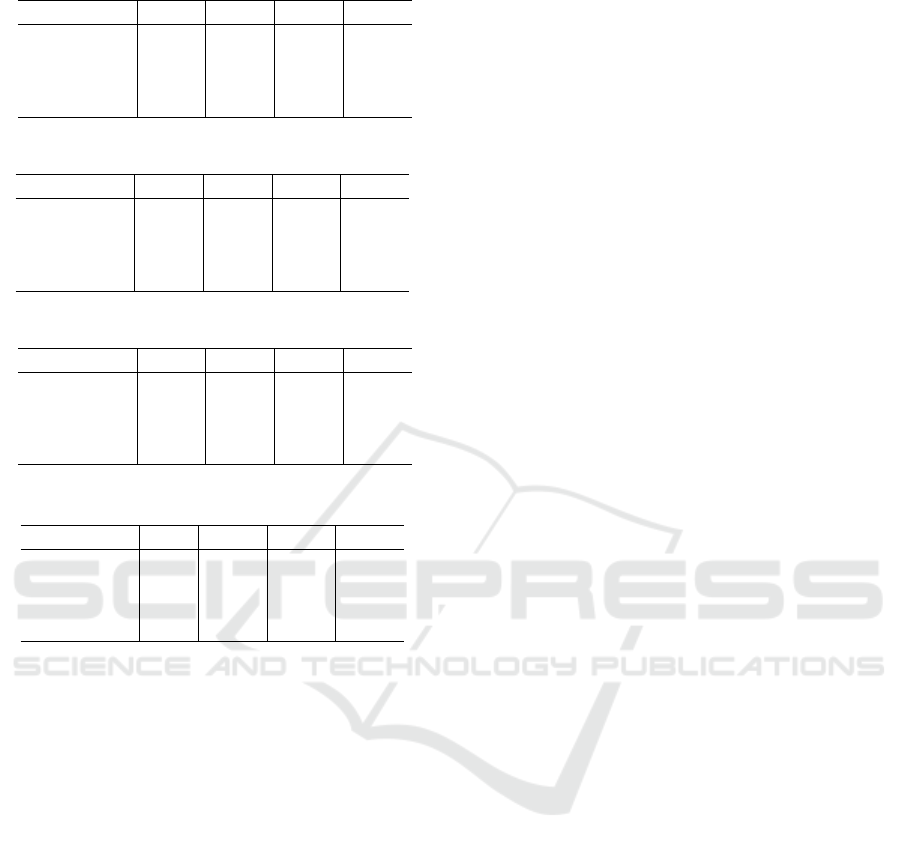
Table 1: Detection rates of our proposed method (β = 0.1).
Attack size 0.05 0.1 0.15 0.2
Random 0.887 0.941 0.963 0.974
Average 0.880 0.938 0.960 0.970
Bandwagon 0.793 0.859 0.897 0.918
Obfuscated 0.827 0.921 0.938 0.956
Table 2: Detection rates of PCA based method (β = 0.1).
Attack size 0.05 0.1 0.15 0.2
Random 0.0 0.010 0.029 0.121
Average 0.667 0.754 0.758 0.762
Bandwagon 0.733 0.770 0.802 0.780
Obfuscated 0.0 0.111 0.323 0.475
Table 3: Detection rates of our proposed method (α = 0.1).
Filler size 0.05 0.1 0.15 0.2
Random 0.761 0.941 0.993 1.0
Average 0.757 0.938 0.980 1.0
Bandwagon 0.318 0.859 0.948 0.987
Obfuscated 0.333 0.921 0.970 1.0
Table 4: Detection rates of PCA based method (α = 0.1).
Filler size 0.05 0.1 0.15 0.2
Random 0.0 0.010 0.367 0.711
Average 0.0 0.754 0.754 0.784
Bandwagon 0.06 0.770 0.770 0.770
Obfuscated 0.0 0.111 0.692 0.715
4.2 Evaluation Results
Tables 1 and 2 show detection rates of our pro-
posed method and the PCA-based method, respec-
tively, when the filler size β = 0.1. Tables 3 and 4
show the results for α = 0.1. It can be shown that the
detection rate of our proposed method is much higher
than that of the PCA-based method for all the settings.
Consequently, we can say that our proposed method
improves robustness to data poisoning dramatically.
5 CONCLUSIONS
This paper proposed recommender systems robust to
data poisoning. Our proposed method is a combina-
tion of matrix factorization and trim learning. The
algorithm trains a model for recommendation while
trimming malicious users and contaminated items.
The experimental results showed that our proposed
method improves robustness to data poisoning dra-
matically.
In the feature, we will conduct additional experi-
ments with other real-world datasets as well as theo-
retical analysis of our proposed method. Furthermore,
we will apply the concept of our proposed method to
more complicated learning methods utilized in rec-
ommender systems.
REFERENCES
Burke, R., Mobasher, B., and Bhaumik, R. (2005). Lim-
ited knowledge shilling attacks in collaborative filter-
ing systems. In Proceedings of the 3rd IJCAI Work-
shop in Intelligent Techniques for Personalization.
Burke, R., Mobasher, B., Williams, C., and Bhaumik, R.
(2006). Classification features for attack detection in
collaborative recommender systems. In Proceedings
of the 12th ACM SIGKDD International Conference
on Knowledge Discovery and Data Mining.
Chirita, P., Nejdl, W., and Zamfir, C. (2005). Preventing
shilling attacks in online recommender systems. In
Proceedings of the 7th ACM international workshop
on Web information and data management (WIDM),
pages 67–74.
GroupLens Research (2016). MovieLens 1M Dataset.
http://grouplens.org/datasets/movielens/.
Jagielski, M., Oprea, A., Biggio, B., Liu, C., Nita-Rotaru,
C., and Li, B. (2018). Manipulating machine learning:
Poisoning attacks and countermeasures for regression
learning. In Proceedings of the 39th IEEE Symposium
on Security and Privacy (S&P).
Koren, Y., Bell, R., and Volinsky, C. (2009). Matrix factor-
ization techniques for recommender systems. Com-
puter and Information Science, 42(8):30–37.
Li, W., Gao, M., Li, H., and Zeng, J. (2016). Shilling at-
tack detection in recommender systems via selecting
patterns analysis. IEICE Transactions on Information
and Systems, E99-10(10).
Mehta, B. (2007). Unsupervised shilling detection for col-
laborative filtering. In Proceedings of the 22nd AAAI
Conference on Artificial Intelligence.
Mobasher, B., Burke, R., Bhaumik, R., and Williams,
C. (2007). Toward trustworthy recommender sys-
tems: An analysis of attack models and algorithm ro-
bustness. ACM Transactions on Internet Technology
(TOIT), 7(23).
Williams, C., Mobasher, B., Burke, R. D., Sandvig, J. J.,
and Bhaumik, R. (2006). Detection of obfuscated
attacks in collaborative recommender systems. In
Proceedings of the ECAI 2006 Workshop on Recom-
mender Systems.
Wu, Z., Cao, J., Mao, B., and Wang, Y. (2011). Semi-SAD:
Applying semi-supervised learning to shilling attack
detection. In Proceedings of the 5th ACM Conference
on Recommender Systems (RecSys).
Wu, Z., Wu, J., Cao, J., and Tao, D. (2012). HySAD:
A semi-supervised hybrid shilling attack detector for
trustworthy product recommendation. In Proceedings
of the 18th ACM SIGKDD International Conference
on Knowledge Discovery and Data Mining.
ICISSP 2020 - 6th International Conference on Information Systems Security and Privacy
724